This page is no longer up to date, please go to my current website.
Philipp Hennig
Philipp is a research scientist at the Max Planck Institute for Intelligent Systems, in T�bingen. As an undergraduate, he studied physics at the University of Heidelberg and at Imperial College in London. While he was preparing his Diplom thesis in Winfried Denk's group, at the Max Planck Institute for Medical Research in Heidelberg, simulating electrons in a scanning electron microscope, he became enthralled by the concepts of probability and uncertainty. So he moved to Cambridge, UK, to work on a doctoral thesis in David MacKay's Inference Group, and at Microsoft Research. At TU Darmstadt, he is currently co-teaching the Seminar Autonome Lernsysteme.
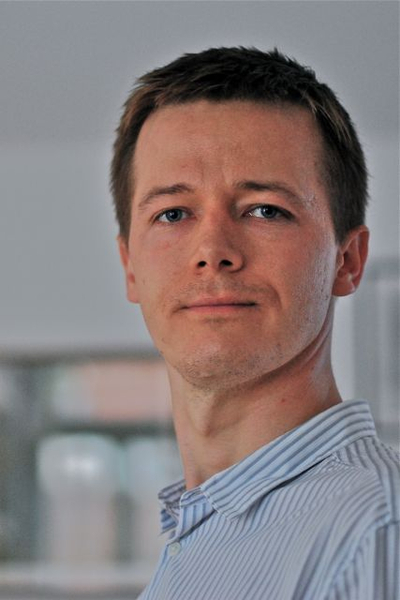
Philipp is interested in all aspects of machine learning. His work in the past has touched supervised, unsupervised, and reinforcement learning, and has found application in areas as diverse as semantic text modelling, automated game playing, psychometrics, and cancer therapy. He focusses on probabilistic methods, such as approximate inference algorithms, graphical models, Monte Carlo algorithms, and nonparametric Bayesian methods.
Probability theory has a number of properties that makes it a promising framework for the "brain" of autonomously acting machines. For example, probabilistic methods can make optimal use of available information to learn as fast as possible. They provide a natural concept of decision making under uncertainty that simultaneously addresses the problems of exploration and exploitation. Probabilistic models are inherently hierarchical and hence suited for complex, structured tasks. And, on a fundamental level, since every rational inference paradigm is isomorphic to that of probability theory, the latter provides a mathematically rigorous framework for uncertainty, learning, and decision making. This power comes at the price of computational complexity: Many probabilistic problems are not analytically solvable. A main focus of Philipp's work is thus the search for good, tractable approximations.
For more up to date information on Philipp's work, including a list of publications, please see his Tübingen site
Philipp can also be found at Google Citations and DBLP
Key References
-
- Philipp Hennig, David Stern, Ralf Herbrich and Thore Graepel (2011). Kernel Topic Models, arXiv preprint.
-
- Philipp Hennig (2011). Optimal Reinforcement Learning for Gaussian Systems, Advances in Neural Information Processing Systems.
-
- Philipp Hennig, David Stern, and Thore Graepel (2010). Coherent Inference on Optimal Play in Game Trees, Artificial Intelligence and Statistics.
Contact Information
Mail: Philipp Hennig, Spemannstr. 38, 72076 Tübingen, Germany
+49-7071-601-542
phennig@tuebingen.mpg.de