Robot Learning: Integrated Project 1+2
The IP Project will continue during the Summer Semester 2025. The Introduction presentation will be held both in-person and via Zoom on 24.04.2025 at 15:20 (see details below in the Meetings section). In case you require more information, please contact ip-projekt@ias.informatik.tu-darmstadt.de asap.
Quick Facts
Outstanding students who have completed 20-00-0753-pj Robot Learning: Integrated Project - Part 1 and 20-00-0754-pj Robot Learning: Integrated Project - Part 2 will be considered for the 20-00-1108-pp Expert Lab on Robot Learning.
In the Robot Learning: Integrated Project, we offer the possibility for highly motivated students to increase their knowledge in robotics and machine learning. This project will allow participants to understand these fields in greater depth and provide hands-on experience. For this purpose, new methods from the Robot Learning lecture are to be implemented. These methods are then proven useful for solving learning problems regarding robotics applications. Participants join together in groups of 2-3 people and get supervision from one or more members of the Intelligent Autonomous Systems Lab. Students may focus on more theoretical or practical work depending on their preference and on the available projects. We are always delighted to answer questions, so please contact us, for example by writing an email to ip-projekt@ias.informatik.tu-darmstadt.de
!
Part 1: Literature Review, Toy Evaluations and Simulation Studies. In the Robot Learning: Integrated Project - Part 1, a current problem in robot learning is tackled by students with the aid of their supervisor. The students conduct a literature review that corresponds to their research interests. Based on this preliminary work, a project plan is developed, the necessary algorithms are tested and a prototypical realization in simulation is created.
Part 2: Evaluation and Submission to a Conference In the Robot Learning: Integrated Project - Part 2 the solutions from Part 1 are completed and applied to a real robot. A scientific article is written on the issue, methods and results, and submitted to a high quality scientific conference or journal. For Part 2, there are numerous exciting robot systems available to students.
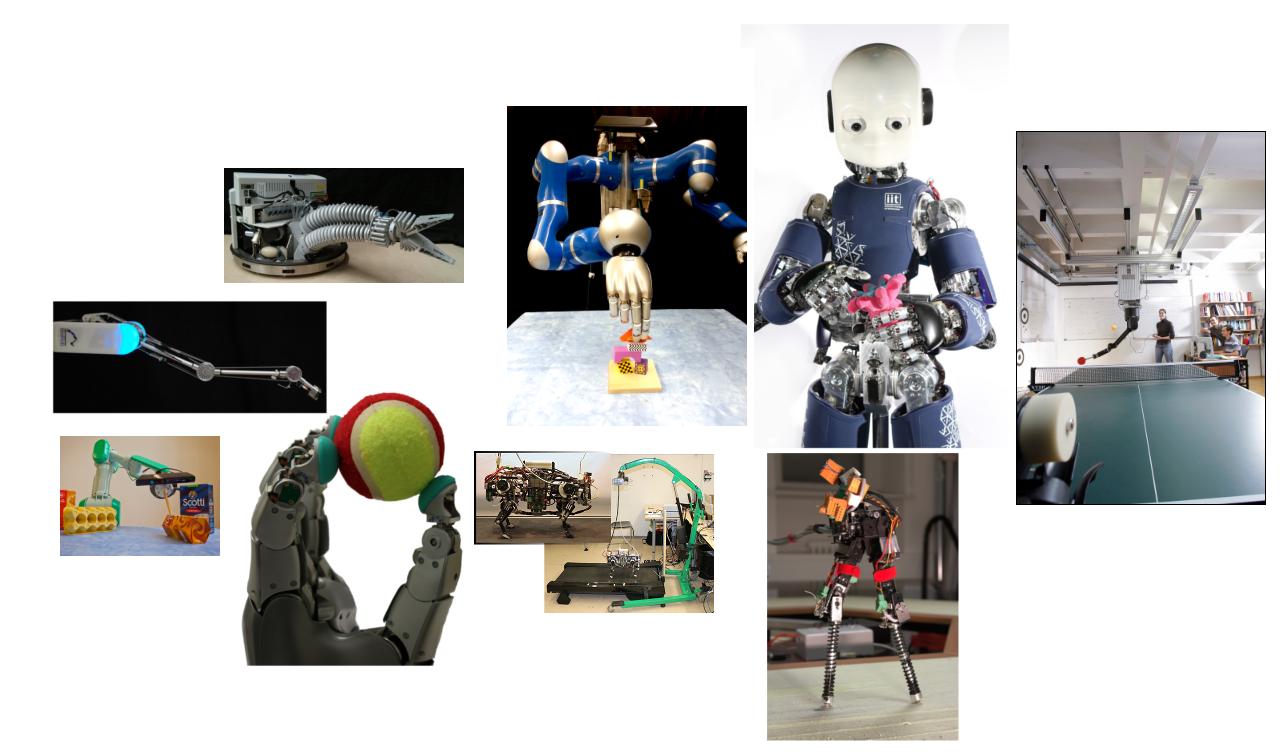
Requirements and Background Knowledge. Mathematics from your undergraduate studies (calculus, statistics), programming (project dependent but usually C/C++, Python), and computer science fundamentals (algorithms). Simultaneous or previous attendance of the Statistical Machine Learning and Robot Learning lectures is recommended!
Meetings & Deadlines
Introduction & Topics presentations | 24.04.2025 15:20-17:00 | Room: S2|02/C110 and Zoom |
Project Application | 30.04.2025 | |
Topic assignment | 08.05.2025 | |
Report Submission | 04.09.2025 | |
Review Submission | 11.09.2025 | |
Final Report Submission | 18.09.2025 | |
Final Presentation | 25.09.2025, 10:00-18:00 | Room: S202/C120 and Zoom |
Project Application
Please apply for a project from the list of offered projects by writing a short motivation letter. In the letter, please address the following questions:
- Which project would you like to try and why?
- Why do you think this project is important?
- What helpful background do you have for the project and what makes you special for that project?
- Your academic aspirations: 1 semester? 2 semesters? Future thesis?
See the Introduction and Topic slides here for the rules and the list of projects! Participants can only apply for two projects. Please specify your priority for the two projects. If you already have a group, please send a joint email.
To apply for a project, please send your project wishes by email to the project supervisor and cc (ip-projekt@ias.informatik.tu-darmstadt.de
) by 30.04.2025.
After an internal discussion with the potential supervisors, it will be decided which topics are assigned to which students. Unfortunately, it is possible that some students do not get a topic!
Please get in contact with the supervisor beforehand to know more about the project.
Report, Review and Presentation
At the end of the IP project (either part 1 or 2), your group is expected to produce three outputs:
- Submit a final report in the ICML style or IROS style as a PDF file. Papers are limited to eight pages, including figures and tables; and additional pages containing only cited references and an appendix (no page limit) are allowed.
- Send your report to ip-projekt@ias.informatik.tu-darmstadt.de, and your supervisors.
- Review and evaluate a report of another group. This process emulates the standard procedure at scientific conferences.
- Give a final presentation on the work you did - 10 minutes + 5 minutes questions.
Your project will be marked based on the following Grading Criteria:
- Creativity and effort on the project
- Technical quality and robustness of the solution
- Has the student grasped and presented the big picture?
- Quality of the report
- Quality of the presentation
To see the offered projects, please login with your TU-ID!
Ongoing IP Projects
Topic | Students | Advisor |
---|---|---|
Robotic Knot Untangling | Tim Missal, Tom Roehner, Gina Leonie Wigginghaus | Berk Gueler from IAS |
Long Horizon Shared Autonomy | Zhihao Han, Yuchao Wu, Ahmad Hamad | Berk Gueler from IAS |
Completed IP Projects
Topic | Students | Advisor |
---|---|---|
Super Fast Multi-Task Reinforcement Learning | Artur Zelik, David Masloub | Ahmed Hendawy, Firas Al-Hafez |
Real-Time Deformable Object Instance Segmentation and Tracking | Jiahong Xue, Yu Deng, Teng Cao | Berk Gueler from IAS, Yufeng Jin from PEARL |
Blending Deep Generative Models using Stochastic Optimization | Julian Ferrer Rodriguez | Kay Hansel and Niklas Funk from IAS |
Training Robots to Play Badminton | Christian Bialas | Alap Kshirsagar |
VLM Humanoid: AI-Powered Robotics for Next-Gen Human-Robot Interaction | Fei Long | Aiswarya Menon from IAS, Suman Pal from IAS, Changqi Chen? from IAS |
AutoPlan: Autonomous Gearbox Assembly | Keqi Zeng | Aiswarya Menon from IAS, Changqi Chen? from IAS, Suman Pal from IAS |
AutoPaint: Automatic Path Planning for High-Precision Robotic Spray Painting | Jannis Neus | Aiswarya Menon from IAS, Suman Pal from IAS, Arjun Vir Datta from IAS |
Building a Test Bench for Tactile Slip Detection | Thomas Ellenberger, Faris Begic, Florian Mach, Paul List, Timo Kolbe | Tim Schneider and Niklas Funk from IAS, Janosch Moos and Omar Elsarha from IMS |
Autoplan- THT Assembly | Yu Jiang | Suman Pal from IAS, Aiswarya Menon from IAS |
Visuotactile Shear Force Estimation | Erik Helmut, Luca Dziarski | Niklas Funk, Boris Belousov |
AI Olympics with RealAIGym | Tim Faust, Erfan Aghadavoodi, Habib Maraqten | Boris Belousov |
Solving Insertion Tasks with RL in the Real World | Nick Striebel,Adriaan Mulder | Joao Carvalho |
Student-Teacher Learning for simulated Quadrupeds | Keagan Holmes, Oliver Griess, Oliver Grein | Nico Bohlinger |
Reinforcement Learning for Contact Rich Manipulation | Dustin Gorecki | Aiswarya Menon, Arjun Datta, Suman Pal |
Autonomous Gearbox Assembly by Assembly by Disassembly | Jonas Chaselon, Julius Thorwarth | Aiswarya Menon, Felix Kaiser, Arjun Datta |
Learning to Assemble from Instruction Manual | Erik Rothenbacker, Leon De Andrade, Simon Schmalfuss | Aiswarya Menon,Vignesh Prasad,Felix Kaiser,Arjun Datta |
Black-box System Identification of the Air Hockey Table | Shihao Li, Yu Wang | Theo Gruner, Puze Liu |
Unveiling the Unseen: Tactile Perception and Reinforcement Learning in the Real World | Alina Böhm, Inga Pfenning, Janis Lenz | Daniel Palenicek, Theo Gruner, Tim Schneider |
XXX: eXploring X-Embodiment with RT-X | Daniel Dennert, Christian Scherer, Faran Ahmad | Daniel Palenicek, Theo Gruner, Tim Schneider, Maximilian Tölle |
Kinodynamic Neural Planner for Robot Air Hockey | Niclas Merten | Puze Liu |
XXX: eXploring X-Embodiment with RT-X | Tristan Jacobs | Daniel Palenicek, Theo Gruner, Tim Schneider, Maximilian Tölle |
Reactive Human-to-Robot Handovers | Fabian Hahne | Vignesh Prasad, Alap Kshirsagar |
Analysis of multimodal goal prompts for robot control | Max Siebenborn | Aditya Bhatt, Maximilian Tölle |
Control Barrier Functions for Assistive Teleoperation | Yihui Huang,Yuanzheng Sun | Berk Gueler, Kay Hansel |
Learning Torque Control for Quadrupeds | Daniel Schmidt, Lina Gaumann | Nico Bohlinger |
Robot Learning for Dynamic Motor Skills | Marcus Kornamann, Qimeng He | Kai Ploeger, Alap Kshirsagar |
Q-Ensembles as a Multi-Armed Bandit | Henrik Metternich | Ahmed Hendawy, Carlo D'Eramo |
Pendulum Acrobatics | Florian Wolf | Kai Ploeger, Pascal Klink |
Learn to play Tangram | Max Zimmermann, Marius Zöller, Andranik Aristakesyan | Kay Hansel, Niklas Funk |
Characterizing Fear-induced Adaptation of Balance by Inverse Reinforcement Learning | Zeyuan Sun | Alap Kshirsagar, Firas Al-Hafez |
Tactile Environment Interaction | Changqi Chen, Simon Muchau, Jonas Ringsdorf | Niklas Funk |
Latent Generative Replay in Continual Learning | Marcel Mittenbühler | Ahmed Hendawy, Carlo D'Eramo |
Memory-Free Continual Learning | Dhruvin Vadgama | Ahmed Hendawy, Carlo D'Eramo |
Simulation of Vision-based Tactile Sensors | Duc Huy Nguyen | Boris Belousov, Tim Schneider |
Learning Bimanual Robotic Grasping | Hanjo Schnellbächer, Christoph Dickmanns | Julen Urain De Jesus, Alap Kshirsagar |
Learning Deep probability fields for planning and control | Felix Herrmann, Sebastian Zach | Davide Tateo, Georgia Chalvatzaki, Jacopo Banfi |
On Improving the Reliability of the Baseline Agent for Robotic Air Hockey | Haozhe Zhu | Puze Liu |
Self-Play Reinforcement Learning for High-Level Tactics in Robot Air Hockey | Yuheng Ouyang | Puze Liu, Davide Tateo |
Kinodynamic Neural Planner for Robot Air Hockey | Niclas Merten | Puze Liu |
Robot Drawing With a Sense of Touch | Noah Becker, Zhijingshui Yang, Jiaxian Peng | Boris Belousov, Mehrzad Esmaeili |
Black-Box System Identification of the Air Hockey Table | Anna Klyushina, Marcel Rath | Theo Gruner, Puze Liu |
Autonomous Basil Harvesting | Jannik Endres, Erik Gattung, Jonathan Lippert | Aiswarya Menon, Felix Kaiser, Arjun Vir Datta, Suman Pal |
Latent Tactile Representations for Model-Based RL | Eric Krämer | Daniel Palenicek, Theo Gruner, Tim Schneider |
Model Based Multi-Object 6D Pose Estimation | Helge Meier | Felix Kaiser, Arjun Vir Datta, Suman Pal |
Reinforcement Learning for Contact Rich Manipulation | Noah Farr, Dustin Gorecki | Aiswarya Menon, Arjun Vir Datta, Suman Pal |
Measuring Task Similarity using Learned Features | Henrik Metternich | Ahmed Hendawy, Pascal Klink, Carlo D'Eramo |
Interactive Semi-Supervised Action Segmentation | Martina Gassen, Erik Prescher, Frederic Metzler | Lisa Scherf, Felix Kaiser, Vignesh Prasad |
Kinematically Constrained Humanlike Bimanual Robot Motion | Yasemin Göksu, Antonio De Almeida Correia | Vignesh Prasad, Alap Kshirsagar |
Control and System identification for Unitree A1 | Lu Liu | Junning Huang, Davide Tateo |
System identification and control for Telemax manipulator | Kilian Feess | Davide Tateo, Junning Huang |
Tactile Active Exploration of Object Shapes | Irina Rath, Dominik Horstkötter | Tim Schneider, Boris Belousov, Alap Kshirsagar |
Object Hardness Estimation with Tactile Sensors | Mario Gomez, Frederik Heller | Alap Kshirsagar, Boris Belousov, Tim Schneider |
Task and Motion Planning for Sequential Assembly | Paul-Hallmann, Nicolas Nonnengießer | Boris Belousov, Tim Schneider, Yuxi Liu |
A Digital Framework for Interlocking SL-Blocks Assembly with Robots | Bingqun Liu | Mehrzad Esmaeili, Boris Belousov |
Learn to play Tangram | Max Zimmermann, Dominik Marino, Maximilian Langer | Kay Hansel, Niklas Funk |
Learning the Residual Dynamics using Extended Kalman Filter for Puck Tracking | Haoran Ding | Puze Liu, Davide Tateo |
ROS Integration of Mitsubishi PA 10 robot | Jonas Günster | Puze Liu, Davide Tateo |
6D Pose Estimation and Tracking for Ubongo 3D | Marek Daniv | Joao Carvalho, Suman Pal |
Task Taxonomy for robots in household | Amin Ali, Xiaolin Lin | Snehal Jauhri, Ali Younes |
Task and Motion Planning for Sequential Assembly | Paul-Hallmann, Patrick Siebke, Nicolas Nonnengießer | Boris Belousov, Tim Schneider, Yuxi Liu |
Learning the Gait for Legged Robot via Safe Reinforcement Learning | Joshua Johannson, Andreas Seidl Fernandez | Puze Liu, Davide Tateo |
Active Perception for Mobile Manipulation | Sophie Lueth, Syrine Ben Abid, Amine Chouchane | Snehal Jauhri |
Combining RL/IL with CPGs for Humanoid Locomotion | Henri Geiss | Firas Al-Hafez, Davide Tateo |
Multimodal Attention for Natural Human-Robot Interaction | Aleksandar Tatalovic, Melanie Jehn, Dhruvin Vadgama, Tobias Gockel | Oleg Arenz, Lisa Scherf |
Hybrid Motion-Force Planning on Manifolds | Chao Jin, Peng Yan, Liyuan Xiang | An Thai Le, Junning Huang |
Stability analysis for control algorithms of Furuta Pendulum | Lu liu, Jiahui Shi, Yuheng Ouyang | Junning Huang, An Thai Le |
Multi-sensorial reinforcement learning for robotic tasks | Rickmer Krohn | Georgia Chalvatzaki, Snehal Jauhri |
Learning Behavior Trees from Video | Nick Dannenberg, Aljoscha Schmidt | Lisa Scherf, SumanPal |
Subgoal-Oriented Shared Control | Zhiyuan Gao, Fengyun Shao | Kay Hansel |
Task Taxonomy for robots in household | Amin Ali, Xiaolin Lin | Snehal Jauhri, Ali Younes |
Learn to play Tangram | Maximilian Langer | Kay Hansel, Niklas Funk |
Theory of Mind Models for HRI under partial Observability | Franziska Herbert, Tobias Niehues, Fabian Kalter | Dorothea Koert, Joni Pajarinen, David Rother |
Learning Safe Human-Robot Interaction | Zhang Zhang | Puze Liu, Snehal Jauhri |
Active-sampling for deep Multi-Task RL | Fabian Wahren | Carlo D'Eramo, Georgia Chalvatzaki |
Interpretable Reinforcement Learning | patrick Vimr | Davide Tateo, Riad Akrour |
Optimistic Actor Critic | Niklas Kappes,Pascal Herrmann | Joao Carvalho |
Active Visual Search with POMDPs | Jascha Hellwig, Mark Baierl | Joao Carvalho, Julen Urain De Jesus |
Utilizing 6D Pose-Estimation over ROS | Johannes Weyel | Julen Urain De Jesus |
Learning Deep Heuristics for Robot Planning | Dominik Marino | Tianyu Ren |
Learning Behavior Trees from Videos | Johannes Heeg, Aljoscha Schmidt and Adrian Worring | Suman Pal, Lisa Scherf |
Learning Decisions by Imitating Human Control Commands | Jonas Günster, Manuel Senge | Junning Huang |
Combining Self-Paced Learning and Intrinsic Motivation | Felix Kaiser, Moritz Meser, Louis Sterker | Pascal Klink |
Self-Paced Reinforcement Learning for Sim-to-Real | Fabian Damken, Heiko Carrasco | Fabio Muratore |
Policy Distillation for Sim-to-Real | Benedikt Hahner, Julien Brosseit | Fabio Muratore |
Neural Posterior System Identification | Theo Gruner, Florian Wiese | Fabio Muratore, Boris Belousov |
Syntethic Dataset generation for Articulation prediction | Johannes Weyel, Niklas Babendererde | Julen Urain, Puze Liu |
Guided Dimensionality Reduction for Black-Box Optimization | Marius Memmel | Puze Liu, Davide Tateo |
Learning Laplacian Representations for continuous MCTS | Daniel Mansfeld, Alex Ruffini | Tuan Dam, Georgia Chalvatzaki, Carlo D'Eramo |
Object Tracking using Depth Carmera | Leon Magnus, Svenja Menzenbach, Max Siebenborn | Niklas Funk, Boris Belousov, Georgia Chalvatzaki |
GNNs for Robotic Manipulation | Fabio d'Aquino Hilt, Jan Kolf, Christian Weiland | Joao Carvalho |
Benchmarking advances in MCTS in Go and Chess | Lukas Schneider | Tuan Dam, Carlo D'Eramo |
Architectural Assembly: Simulation and Optimization | Jan Schneider | Boris Belousov, Georgia Chalvatzaki |
Probabilistic Object Tracking using Depth Carmera | Jan Emrich, Simon Kiefhaber | Niklas Funk, Boris Belousov, Georgia Chalvatzaki |
Bayesian Optimization for System Identification in Robot Air Hockey | Chen Xue. Verena Sieburger | Puze Liu, Davide Tateo |
Benchmarking MPC Solvers in the Era of Deep Reinforcement Learning | Darya Nikitina, Tristan Schulz | Joe Watson |
Enhancing Attention Aware Movement Primitives | Artur Kruk | Dorothea Koert |
Towards Semantic Imitation Learning | Pengfei Zhao | Julen Urain & Georgia Chalvatzaki |
Can we use Structured Inference Networks for Human Motion Prediction? | Hanyu Sun, Liu Lanmiao | Julen Urain & Georgia Chalvatzaki |
Reinforcement Learning for Architectural Combinatorial Optimization | Jianpeng Chen, Yuxi Liu, Martin Knoll, Leon Wietschorke | Boris Belousov, Georgia Chalvatzaki, Bastian Wibranek |
Architectural Assembly With Tactile Skills: Simulation and Optimization | Tim Schneider, Jan Schneider | Boris Belousov, Georgia Chalvatzaki, Bastian Wibranek |
Bayesian Last Layer Networks | Jihao Andreas Lin | Joe Watson, Pascal Klink |
BATBOT: BATter roBOT for Baseball | Yannick Lavan, Marcel Wessely | Carlo D'Eramo |
Benchmarking Deep Reinforcement Learning | Benedikt Volker | Davide Tateo, Carlo D'Eramo, Tianyu Ren |
Model Predictive Actor-Critic Reinforcement Learning of Robotic Tasks | Daljeet Nandha | Georgia Chalvatzaki |
Dimensionality Reduction for Reinforcement Learning | Jonas Jäger | Michael Lutter |
From exploration to control: learning object manipulation skills through novelty search and local adaptation | Leon Keller | Svenja Stark, Daniel Tanneberg |
Robot Air-Hockey | Patrick Lutz | Puze Liu, Davide Tateo |
Learning Robotic Grasp of Deformable Object | Mingye Zhu, Yanhua Zhang | Tianyu Ren |
Teach a Robot to solve puzzles with intrinsic motivation | Ali Karpuzoglu | Georgia Chalvatzaki, Svenja Stark |
Inductive Biases for Robot Learning | Rustam Galljamov | Boris Belousov, Michael Lutter |
Accelerated Mirror Descent Policy Search | Maximilian Hensel | Boris Belousov, Tuan Dam |
Foundations of Adversarial and Robust Learning | Janosch Moos, Kay Hansel | Svenja Stark, Hany Abdulsamad |
Likelihood-free Inference for Reinforcement Learning | Maximilian Hensel, Kai Cui | Boris Belousov |
Risk-Aware Reinforcement Learning | Maximillian Kircher, Angelo Campomaggiore, Simon Kohaut, Dario Perrone | Samuele Tosatto, Dorothea Koert |
Jonas Eschmann, Robin Menzenbach, Christian Eilers | Boris Belousov, Fabio Muratore | |
Learning Symbolic Representations for Abstract High-Level Planning | Zhiyuan Hu, Claudia Lölkes, Haoyi Yang | Svenja Stark Daniel Tanneberg |
Learning Perceptual ProMPs for Catching Balls | Axel Patzwahl | Dorothea Koert, Michael Lutter |
Bayesian Inference for Switching Linear Dynamical Systems | Markus Semmler, Stefan Fabian | Hany Abdulsamad |
Characterization of WAM Dynamics | Kai Ploeger | Dorothea Koert, Michael Lutter |
Deep Reinforcement Learning for playing Starcraft II | Daniel Palenicek, Marcel Hussing, Simon Meister | Filipe Veiga |
Enhancing Exploration in High-Dimensional Environments Δ | Lu Wan, Shuo Zhang | Simone Parisi |
Building a Grasping Testbed | Devron Williams | Oleg Arenz |
Online Dynamic Model Learning | Pascal Klink | Hany Abdulsamad, Alexandros Paraschos |
Spatio-spectral Transfer Learning for Motor Performance Estimation | Karl-Heinz Fiebig | Daniel Tanneberg |
From Robots to Cobots | Michael Burkhardt, Moritz Knaust, Susanne Trick | Dorothea Koert, Marco Ewerton |
Learning Hand-Kinematics | Sumanth Venugopal, Deepak Singh Mohan | Gregor Gebhardt |
Goal-directed reward generation | Alymbek Sadybakasov | Boris Belousov |
Learning Grammars for Sequencing Movement Primitives | Kim Berninger, Sebastian Szelag | Rudolf Lioutikov |
Learning Deep Feature Spaces for Nonparametric Inference | Philipp Becker | Gregor Gebhardt |
Lazy skill learning for cleaning up a table | Lejla Nukovic, Moritz Fuchs | Svenja Stark |
Reinforcement Learning for Gait Learning in Quadrupeds | Kai Ploeger, Zinan Liu | Svenja Stark |
Learning Deep Feature Spaces for Nonparametric Inference | Philipp Becker | Gregor Gebhardt |
Optimal Control for Biped Locomotion | Martin Seiler, Max Kreischer | Hany Abdulsamad |
Semi-Autonomous Tele-Operation | Nick Heppert, Marius, Jeremy Tschirner | Oleg Arenz |
Teaching People how to Write Japanese Characters | David Rother, Jakob Weimar, Lars Lotter | Marco Ewerton |
Local Bayesian Optimization | Dmitry Sorokin | Riad Akrour |
Bayesian Deep Reinforcement Learning -Tools and Methods- | Simon Ramstedt | Simone Parisi |
Controlled Slip for Object Release | Steffen Kuchelmeister, Albert Schotschneider | Filipe Veiga |
Learn intuitive physics from videos | Yunlong Song, Rong Zhi | Boris Belousov |
Learn an Assembling Task with Swarm Robots | Kevin Daun, Marius Schnaubelt | Gregor Gebhardt |
Learning To Sequence Movement Primitives | Christoph Mayer | Christian Daniel |
Learning versatile solutions for Table Tennis | Felix End | Gerhard Neumann Riad Akrour |
Learning to Control Kilo-Bots with a Flashlight | Alexander Hendrich, Daniel Kauth | Gregor Gebhardt |
Playing Badminton with Robots | J. Tang, T. Staschewski, H. Gou | Boris Belousov |
Juggling with Robots | Elvir Sabic, Alexander Wölker | Dorothea Koert |
Learning and control for the bipedal walker FaBi | Manuel Bied, Felix Treede, Felix Pels | Roberto Calandra |
Finding visual kernels | Fide Marten, Dominik Dienlin | Herke van Hoof |
Feature Selection for Tetherball Robot Games | Xuelei Li, Jan Christoph Klie | Simone Parisi |
Inverse Reinforcement Learning of Flocking Behaviour | Maximilian Maag, Robert Pinsler | Oleg Arenz |
Control and Learning for a Bipedal Robot | Felix Treede, Phillip Konow, Manuel Bied | Roberto Calandra |
Perceptual coupling with ProMPs | Johannes Geisler, Emmanuel Stapf | Alexandros Paraschos |
Learning to balance with the iCub | Moritz Nakatenus, Jan Geukes | Roberto Calandra |
Generalizing Models for a Compliant Robot | Mike Smyk | Herke van Hoof |
Learning Minigolf with the BioRob | Florian Brandherm | Marco Ewerton |
iCub Telecontrol | Lars Fritsche, Felix Unverzagt | Roberto Calandra |
REPS for maneuvering in Robocup | Jannick Abbenseth, Nicolai Ommer | Christian Daniel |
Learning Ball on a Beam on the KUKA lightweight arms | Bianca Loew, Daniel Wilberts | Christian Daniel |
Sequencing of DMPs for Task- and Motion Planning | Markus Sigg, Fabian Faller | Rudolf Lioutikov |
Tactile Exploration and Mapping | Thomas Arnreich, Janine Hoelscher | Tucker Hermans |
Multiobjective Reinforcement Learning on Tetherball BioRob | Alexander Blank, Tobias Viernickel | Simone Parisi |
Semi-supervised Active Grasp Learning | Simon Leischnig, Stefan Lüttgen | Oliver Kroemer |
Lecturer
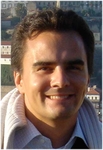
Jan Peters heads the Intelligent Autonomous Systems Lab at the Department of Computer Science at the TU Darmstadt. Jan has studied computer science, electrical, control, mechanical and aerospace engineering. You can find Jan Peters in the Robert-Piloty building in S2 | 02 find in room E314.