I have graduated and moved to Bosch Center for Artificial Intelligence in Renningen near Stuttgart.
Fabio Muratore
Research Interests
Robotics, Machine Learning,
Physics Simulations,
Automatic Control
More Information
Publications Google Scholar Code
Contact Information
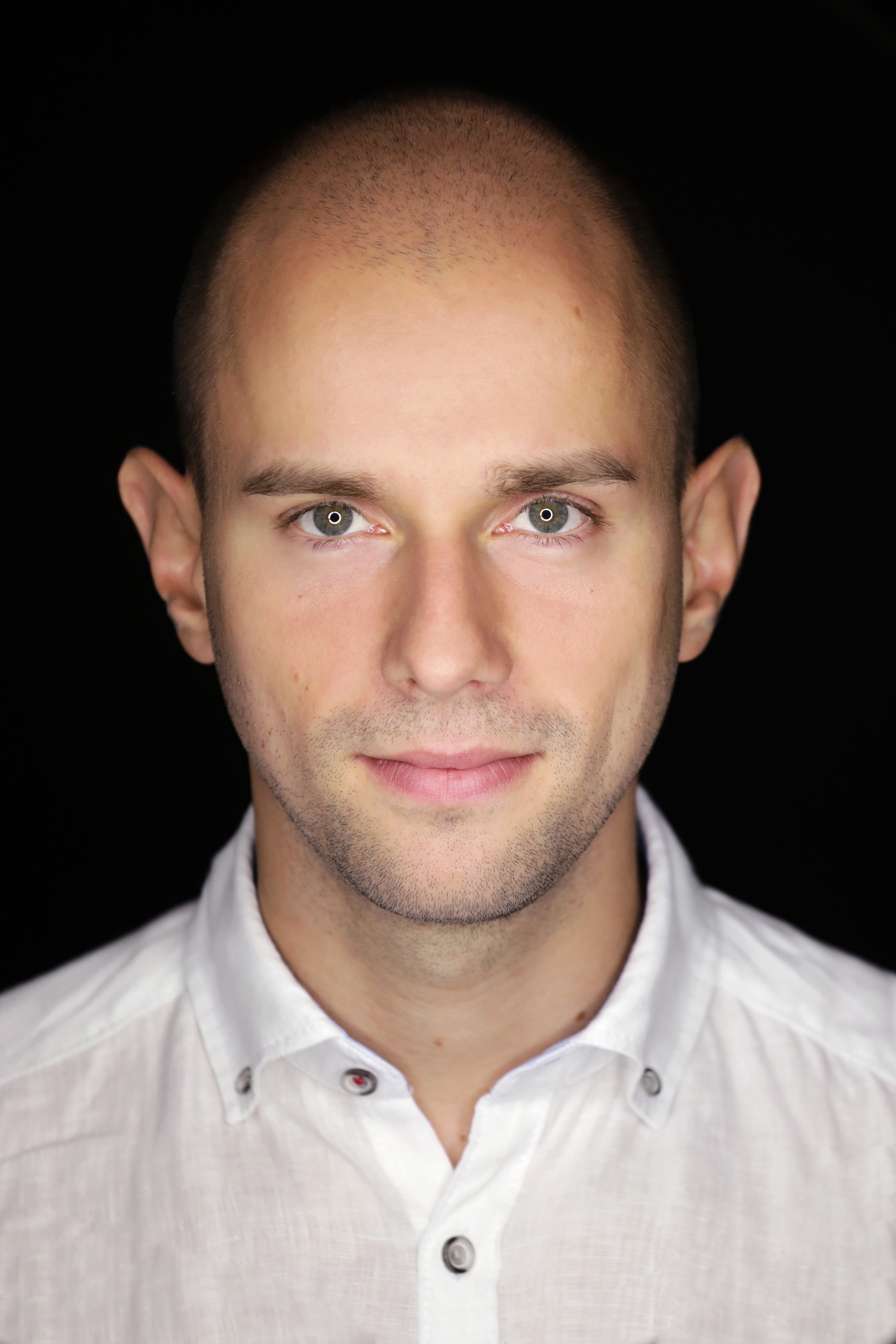
Fabio received his bachelor's degree in mechatronics as well as his masters's degree in mechanical engineering from the Technical University of Munich. During his studies, he focused on automatic control, structural dynamics, and artificial neural networks.
Research Topic
There is a large consent both in academia as well as in industry that physical human-robot interaction is attributed a large potential for future robotic applications. While being a generic technology, applications emerge increasingly in the factory domain, particularly in the production and assembly processes.
Learning concepts for manipulation tasks is, however still rather academic as it is, imposing a number of assumptions on the underlying problem, and requiring scientists to produce the results. In particular, learning of force-based manipulation is mainly realized using kinesthetic teaching, necessitating expensive and specialized hardware as well as an expert.
The joint research project Motor Dreaming between IAS and HRI takes a different perspective on the problem. It targets to combine data-driven learning and exploitative learning in an efficient way. As an alternative to building a skill representation exclusively from data, the core idea is to make additional use of generative models that allow an internal simulation of the task, also known as mental rehearsal. This step involves devising physical simulation models from the real situation, and being able to play them through in different variations. Such a mental rehearsal allows incorporating uncertainty, such aiming to increase the robustness of reproduction by learning solutions that can deal with large parameter variations.
Key References
-
- Muratore, F.; Ramos, F.; Turk, G.; Yu, W.; Gienger, M.; Peters, J. (2022). Robot Learning from Randomized Simulations: A Review, Frontiers in Robotics and AI, 9.
-
- Muratore, F.; Gruner, T.; Wiese, F.; Belousov, B.; Gienger, M.; Peters, J. (2021). Neural Posterior Domain Randomization, Conference on Robot Learning (CoRL).
-
- Muratore, F.; Eilers, C.; Gienger, M.; Peters, J. (2021). Data-efficient Domain Randomization with Bayesian Optimization, IEEE Robotics and Automation Letters (RA-L), with Presentation at the IEEE International Conference on Robotics and Automation (ICRA), IEEE.
-
- Muratore, F.; Gienger, M.; Peters, J. (2021). Assessing Transferability from Simulation to Reality for Reinforcement Learning, IEEE Transactions on Pattern Analysis and Machine Intelligence (PAMI), 43, 4, pp.1172-1183, IEEE.
Code
Check out my open-source repository for reinforcement learning from randomized simulations SimRLacra.
In the rare event you need a Python implementation of a backlash model, this might help you.
Videos
Neural Posterior Domain Randomization (NPDR)
Bayesian Domain Randomization (BayRn)
Simulation-Based Policy Optimization with Transferability Assessment (SPOTA)
Sim-to-real evaluation transfer of a policy learned with of SPOTA on the Ball-Balancer and Cart-Pole platform
Spotlight talk at CoRL 2018
Evaluation of SPOTA, EPOpt, TRPO, and LQR policies in Vortex varying selected physics parameters of the simulation
Cross-evaluation of SPOTA, EPOpt, TRPO, and LQR policies trained in Vortex and in Bullet then tested in both
(Blog) Posts
I wrote a very easy-to-follow blog post on quantifying the transferability of sim-to-real control policies at sim2realai.github.io.
You can also find a short how-to on creating beautiful figures for your papers with Inkscape and Latex here.
Teaching & Student Supervision
Completed Theses and Projects
Start | Type | In coorperation with | Student(s) | Topic | Document |
---|---|---|---|---|---|
2021 | Master's Thesis | Boris Belousov | Theo Gruner | Wasserstein-Optimal Bayesian System Identification for Domain Randomization | |
2020 | Integrated Project | - | Fabian Damken, Heiko Carrasco | Combining Domain Randomization and Self-Paced Contextual Reinforcement Learning | |
2020 | Integrated Project | - | Julien Brosseit, Benedikt Hahner | Combining Domain Randomization and Policy Destillation | |
2020 | Integrated Project | Boris Belousov | Theo Gruner, Arlene Kühn, Florian Wiese | Likelihood-Free Inference for Domain Randomization | |
2020 | Master's Thesis | Thomas Weisswange | David Rother | Reinforcement Learning in Decentralized Multi-Goal Multi-Agent Settings | |
2020 | Master's Thesis | Claudio Zito | Jonas Eschmann | Partially Unsupervised Deep Meta Reinforcement Learning | |
2019 | Student Project | GCC | 6 groups à 2 students | Parallelizing a Reinforcement Learning Algorithm | |
2019 | Bachelor's Thesis | Benedict Flade | Simon Kohaut | Simultaneous Map Correction and Auto Calibration for Hybrid Localization | |
2019 | Bachelor's Thesis | HRI | Robin Menzenbach | Benchmarking Sim2Real Algorithms on Real-World Platforms | |
2019 | Bachelor's Thesis | Boris Belousov & HRI | Christian Eilers | Bayesian Optimization for Learning from Randomized Simulations | |
2019 | Seminar | - | 3 groups à 3 students | Reinforcement Learning Class | |
2019 | Integrated Project | Boris Belousov | Jonas Eschmann, Robin Menzenbach, Christian Eilers | Underactuated Trajectory-Tracking Control for Long-Exposure Photography (part II) | |
2019 | Master's Thesis | HRI | Markus Lamprecht | Benchmarking Robust Control against Reinforcement Learning Methods on a Robotic Balancing Problem | |
2018 | Integrated Project | Boris Belousov | Jonas Eschmann, Robin Menzenbach, Christian Eilers | Underactuated Trajectory-Tracking Control for Long-Exposure Photography (part I) | |
2018 | Master's Thesis | HRI | Felix Treede | Learning Robust Control Policies from Simulations with Perturbed Parameters |