Currently Available Theses Topics
We offer these current topics directly for Bachelor and Master students at TU Darmstadt who can feel free to DIRECTLY contact the thesis advisor if you are interested in one of these topics. Excellent external students from another university may be accepted but are required to first email Jan Peters before contacting any other lab member for a thesis topic. Note that we cannot provide funding for any of these theses projects.
We highly recommend that you do either our robotics and machine learning lectures (Robot Learning, Statistical Machine Learning) or our colleagues (Grundlagen der Robotik, Probabilistic Graphical Models and/or Deep Learning). Furthermore, we strongly recommend that you take both Robot Learning: Integrated Project, Part 1 (Literature Review and Simulation Studies) and Part 2 (Evaluation and Submission to a Conference) before doing a thesis with us.
In addition, we are usually happy to devise new topics on request to suit the abilities of excellent students. Please DIRECTLY contact the thesis advisor if you are interested in one of these topics. When you contact the advisor, it would be nice if you could mention (1) WHY you are interested in the topic (dreams, parts of the problem, etc), and (2) WHAT makes you special for the projects (e.g., class work, project experience, special programming or math skills, prior work, etc.). Supplementary materials (CV, grades, etc) are highly appreciated. Of course, such materials are not mandatory but they help the advisor to see whether the topic is too easy, just about right or too hard for you.
Only contact *ONE* potential advisor at the same time! If you contact a second one without first concluding discussions with the first advisor (i.e., decide for or against the thesis with her or him), we may not consider you at all. Only if you are super excited for at most two topics send an email to both supervisors, so that the supervisors are aware of the additional interest.
FOR FB16+FB18 STUDENTS: Students from other depts at TU Darmstadt (e.g., ME, EE, IST), you need an additional formal supervisor who officially issues the topic. Please do not try to arrange your home dept advisor by yourself but let the supervising IAS member get in touch with that person instead. Multiple professors from other depts have complained that they were asked to co-supervise before getting contacted by our advising lab member.
NEW THESES START HERE
Neural-Symbolic Reasoning for Safe Robot Manipulation
Scope: Master Thesis
Advisor: Puze Liu / Zihan Ye
Added: 2025-03-20
Start: ASAP
Topic: Despite their powerful representational ability, deep neural networks (DNNs) suffer from a fundamental limitation: the “black-box” nature of DNN makes it difficult to guarantee that the outputs are always reasonable and reliable. This lack of transparency raises significant concerns, particularly in safety-critical applications such as robotics, where erroneous predictions or unexpected behaviors can lead to hazardous consequences. The “hallucinations” problem from the generative model severely impedes the adoption of AI in domains that demand high levels of reliability, safety, and interpretability.
In contrast, formal logic-based reasoning provides a structured, transparent, and interpretable framework for decision-making, making it an attractive approach for ensuring safety in AI-driven applications. By integrating deep learning with formal reasoning, a neural-symbolic reasoning framework offers significant advantages: the expressive power and adaptability of neural networks combined with the rigor, interpretable symbolic logic.
In this thesis, we will investigate the neural-symbolic reasoning framework to address safety challenges in robotics. Specifically, we will explore scalable differentiable reasoning techniques to construct safety constraints and develop robust policies that ensure safety for robotic tasks.
Interested students can apply by sending an email to puze.liu@dfki.de.
Requirements
- Strong programming skills in Python
- Basic knowledge of robotics, machine learning, and optimization
- Experience with deep learning libraries (e.g., PyTorch)
- Knowledge on neural symbolic models is a plus
Thesis objectives
- Investigate how to translate symbolic safety specifications into logic-based representation.
- Explore the possibility of training a neural-symbolic reasoner as safety constraints
- Investigate how to integrate the trained safety prediction model into planning, control and RL.
Narrowing the Sim2Real Gap with Differentiable Tactile Simulation
Scope: Bachelor/Master's thesis
Advisor: Guillaume Duret
Added: 2025-01-13
Start: ASAP

Topic: Sim2Real (simulation-to-real transfer) is critical in robotic learning due to the high demand for data required to train effective models. Simulations provide a controlled and cost-effective means to generate data, reducing the risks and challenges associated with training in real-world environments. However, the inherent discrepancy between simulated and real-world conditions, known as the _sim2real gap_, presents a significant challenge. Minimizing this gap is essential to ensure the transferability of models from simulation to real-world applications. This thesis will investigate methods to refine and adapt simulations to closely replicate the specific characteristics of real tactile sensors, such as the GelSight Mini. The research will focus on leveraging advanced simulation techniques, including differentiable rendering, to optimize simulator parameters automatically. These properties may include camera distortion, sensor positioning, lighting conditions, and color rendering, ensuring high fidelity with the real sensor. By systematically exploring and comparing approaches for fine-tuning simulation environments, this work aims to enhance the accuracy of simulated data and improve the robustness of robotic learning models in real-world settings. The findings will contribute to reducing the sim2real gap and advancing the applicability of tactile sensing in robotics.
Interested students can apply by sending an email to guillaume.duret@ec-lyon.fr, attaching the following documents:
- Curriculum Vitae (CV)
- A motivation letter explaining the reasons for applying to this thesis and outlining academic and career objectives
Requirements
- Strong programming skills in Python
- Basic knowledge of robotics, machine learning, and optimization
- Experience with deep learning libraries (e.g., PyTorch)
- Experience with rendering and computer graphics is a plus
Thesis objectives
- Use differentiable rendering libraries [1] in the context of visual-tactile sensors such as the GelSight mini and demonstrate their ability to produce realistic rendering simulated properties.
- Compare the methods in terms of ease of use and quality with the existing state-of-the-art methods [2] in different contexts.
- Demonstrate the effectiveness of the developed method in a robotic manipulation task
References
[1] https://mitsuba.readthedocs.io/en/stable/src/inverse_rendering/pytorch_mitsuba_interoperability.html
[2] Si, Z., & Yuan, W. (2021). Taxim: An Example-based Simulation Model for GelSight Tactile Sensors. _IEEE Robotics and Automation Letters, PP_, 1-1.
Human-Robot Partner Juggling
Scope: Master's thesis
Advisor: Kai Ploeger, Alap Kshirsagar, Abir Chowdhury
Added: 2025-01-09
Start: ASAP
Topic:
Toss juggling is one of the most challenging dynamic skills that humans learn to perform through trial and error. Recently, researchers have successfully demonstrated robotic toss juggling with high-speed robotic manipulators [1-3]. However, existing works focus primarily on solo-robot juggling and do not explore human-robot interaction in this context, except for [4]. This project seeks to develop robot controllers for closed-loop human-robot partner juggling, pushing the boundaries of dynamic shared manipulation tasks. The objectives include designing control and learning algorithms that can synchronize the robot’s actions with those of a human partner for dyadic juggling. The thesis tasks will involve:
- Designing and implementing a robotic perception pipeline to track balls and human partner movements in real time
- Developing closed-loop robot controllers for human-robot partner juggling
- Implementing and evaluating the approach on a barrett WAM robot
Requirements
- Strong programming skills in Python and C++
- Knowledge in control, computer vision, and machine learning
- Prior hands-on robotics experience is a plus
Interested students can apply by sending an e-mail to kai.ploeger@tu-darmstadt.de and alap.kshirsagar@tu-darmstadt.de, attaching the documents mentioned below:
- Curriculum Vitae (CV)
- Motivation letter explaining why you would like to work on this topic and why you are the ideal candidate
References
[1] Ploeger, K., Lutter, M., & Peters, J. (2021, October). High acceleration reinforcement learning for real-world juggling with binary rewards. In Conference on Robot Learning (pp. 642-653). PMLR.
[2] Ploeger, K., & Peters, J. (2022, October). Controlling the cascade: Kinematic planning for n-ball toss juggling. In 2022 IEEE/RSJ International Conference on Intelligent Robots and Systems (IROS) (pp. 1139-1144). IEEE.
[3] Andreu, M. G., Ploeger, K., & Peters, J. (2024, October). Beyond the Cascade: Juggling Vanilla Siteswap Patterns. In 2024 IEEE/RSJ International Conference on Intelligent Robots and Systems (IROS) (pp. 2928-2934). IEEE.
[4] Kober, J., Glisson, M., & Mistry, M. (2012, November). Playing catch and juggling with a humanoid robot. In 2012 12th IEEE-RAS International Conference on Humanoid Robots (Humanoids 2012) (pp. 875-881). IEEE.
Safe and stable imitation learning for dexterous manipulation skills
Scope: Master's thesis
Advisor: Cristiana Mirandade Farias, Davide Tateo
Added: 2024-11-25
Start: ASAP
Topic:
Learned stable vector fields are one of the state-of-the-art techniques for imitation learning in robotics and one of the few methodologies with theoretical guarantees of achieving the nominal motion. With these techniques, it is possible to learn, from a few demonstrations, robust trajectories reaching stable points or limit cycle attractors. However, despite their theoretical guarantees and learning robustness, often more modern approaches based on diffusion are preferred, as their greater flexibility allows them to solve more complex tasks. In this thesis, we aim to prove that these methodologies can actually scale to solve complex tasks if integrated with state-of-the-art grasping techniques. Starting from an already existing codebase, we aim at developing a structured approach able to solve complex dexterous manipulation tasks from few demonstrations and maintaining the theoretical guarantees. Furthermore, we will integrate already existing and develop novel methodologies to ensure safety and workspace limits, allowing for environment-aware motion generation. This will be enabled through object-centric grasping algortihms that can ensure the execution of task-aware grasps which are informed from the demonstrations.
Interested students can apply by sending an e-mail to cristiana.farias@tu-darmstadt.de and davide.tateo@tu-darmstadt.de, attaching the documents mentioned below:
- Curriculum Vitae (CV);
- A motivation letter explaining the reason for applying for this thesis and academic/career objectives.
Requirements
- Good Python programming skills
- Basic Knowledge of Robotics and Machine Learning
- Experience with deep learning libraries (Torch)
- Experience with Mujoco is a plus
Thesis objectives
- Develop a simulator framework in MuJoCo, starting from the existing codebase and material
- Integrate dexterous grasping and safety approaches into an existing codebase for a stable vector field
- Deploy the policy on the Darias robot, a bimanual manipulator with dexterous hands
- Optionally, extend the approach to use flow matching, instead of simple normalizing flows
References
[1] Urain, J., Tateo, D., & Peters, J. (2022). Learning stable vector fields on lie groups. IEEE Robotics and Automation Letters, 7(4), 12569-12576.
[2] Urain, J., Ginesi, M., Tateo, D., & Peters, J. (2020, October). Imitationflow: Learning deep stable stochastic dynamic systems by normalizing flows. In 2020 IEEE/RSJ International Conference on Intelligent Robots and Systems (IROS) (pp. 5231-5237). IEEE.
[3] De Farias, C., Tamadazte, B., Adjigble, M., Stolkin, R., & Marturi, N. (2024). Task-informed grasping of partially observed objects. IEEE Robotics and Automation Letters. Vol. 9, no. 10, pp. 8394-8401.
Dynamic Tiles for Deep Reinforcement Learning
Scope: Master's thesis
Advisor: Ahmed Hendawy, Davide Tateo, Carlo D'Eramo
Added: 2024-11-21
Start: ASAP
Topic:
Linear approximators in Reinforcement Learning are well-studied and come with an in-depth theoretical analysis. However, linear methods require defining a set of features of the state to be used by the linear approximation. Unfortunately, the feature construction process is a particularly problematic and challenging task. Deep Reinforcement learning methods have been introduced to mitigate the feature construction problem: these methods do not require handcrafted features, as features are extracted automatically by the network during learning, using gradient descent techniques.
In simple reinforcement learning tasks, however, it is possible to use tile coding as features: Tiles are simply a convenient discretization of the state space that allows us to easily control the generalization capabilities of the linear approximator. The objective of this thesis is to design a novel algorithm for automatic feature extraction that generates a set of features similar to tile coding, but that can arbitrarily partition the state space and deal with arbitrary complex state space, such as images. The idea is to combine the feature extraction problem directly with Linear Reinforcement Learning methods, defining an algorithm that is able both to have the theoretical guarantees and good convergence properties of these methods and the flexibility of Deep Learning approaches.
Interested students can apply by sending an e-mail to ahmed.hendawy@tu-darmstadt.de and attaching the documents mentioned below:
- Curriculum Vitae (CV);
- A motivation letter explaining the reason for applying for this thesis and academic/career objectives.
Minimum knowledge
- Good Python programming skills;
- Basic knowledge of Reinforcement Learning.
Preferred knowledge
- Knowledge of the PyTorch library;
- Knowledge of the Atari environments (ale-py library).
- Knowledge of the MushroomRL library.
Accepted candidate will
- Define a generalization of tile coding working with an arbitrary input set (including images);
- Design a learning algorithm to adapt the tiles using data of interaction with the environment;
- Combine feature learning with standard linear methods for Reinforcement Learning;
- Verify the novel methodology in simple continuous state and discrete actions environments;
- (Optionally) Extend the experimental analysis to the Atari environment setting.
Parallel MPC for Diffusion Policy Learning
Scope: Master thesis
Advisor: Firas Al-Hafez, An Thai Le
Added: 2024-11-13
Start: ASAP
Topic:
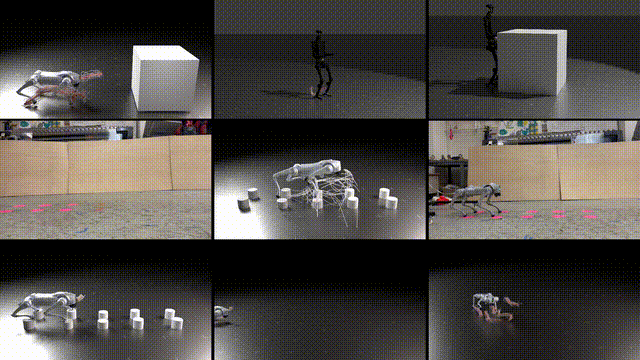
Model Predictive Control (MPC) has proven to be a powerful tool for many robotic applications; however, using MPC with a single dynamics model often leads to mismatches in real-world scenarios. Recently, Diffusion Policies have demonstrated significant real-world success, though their training typically requires extensive data. With advancements in parallel simulation, we aim to explore MPC as an effective expert data generator to create large-scale datasets that can be distilled into Diffusion Policies. Our focus will be on whole-body control for humanoid robots. If results show promise in simulation, we will highly encourage real-world implementation.The thesis tasks will involve:
- literature review
- implementation and evaluation of different parallel MPC pipelines (build on prior work)
- implementation and evaluation of different diffusion policy pipelines for distillation
Requirements
- Very strong Python programming skills
- Knowledge in Robotics and Machine Learning
- Experience with deep learning libraries (Jax, Torch)
- Experience with Mujoco is a plus
Interested students can apply by sending an e-mail to firas.al-hafez@tu-darmstadt.de and attaching the documents mentioned below:
- Curriculum Vitae
- Motivation letter explaining why you would like to work on this topic and why you are the perfect candidate
References
[1] Xue et al., Full-Order Sampling-Based MPC for Torque-Level Locomotion Control via Diffusion-Style Annealing
Active Exploration for Bimanual Grasping
Scope: Master thesis
Advisor: Cristiana Mirandade Farias, Alap Kshirsagar, Tim Schneider
Added: 2024-10-29
Start: ASAP
Topic:
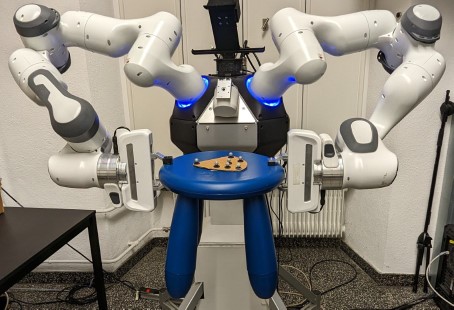
Grasping unknown objects is a challenging task in robotics, particularly when only partial sensory information is available. One solution for this problem is to employ active exploration techniques that integrate vision and tactile sensory modalities to guide the exploratory process over such objects. While previous works have tackled active exploration for object understanding [1] and grasp optimization [2, 3] with a single arm on static scenes, bimanual grasp exploration remains a complex and relatively underexplored challenge. In particular, for larger or irregularly shaped objects, bimanual grasping offers several advantages, as it allows the robot to leverage both hands for added stability. This project seeks to study active exploration algorithms and employ them to find optimal bimanual grasps of larger objects. The thesis tasks will involve:
- Literature review on active exploration for grasping
- Implementation of an active exploration algorithm for improved bimanual-grasp stability
- Evaluation of the approach in simulation and on a physical robot platform.
Requirements
- Strong Python programming skills
- Knowledge in Robotics and Machine Learning
- Experience with deep learning libraries and/or computer vision is a plus
Interested students can apply by sending an e-mail to cristiana.farias@tu-darmstadt.de and attaching the documents mentioned below:
- Curriculum Vitae
- Motivation letter explaining why you would like to work on this topic and why you are the perfect candidate
References
[1] Z. Yi et al., "Active tactile object exploration with Gaussian processes," 2016 IEEE/RSJ International Conference on Intelligent Robots and Systems (IROS), Daejeon, Korea (South), 2016, pp. 4925-4930, doi: 10.1109/IROS.2016.7759723.
[2] C. de Farias, N. Marturi, R. Stolkin and Y. Bekiroglu, "Simultaneous Tactile Exploration and Grasp Refinement for Unknown Objects," in IEEE Robotics and Automation Letters, vol. 6, no. 2, pp. 3349-3356, April 2021, doi: 10.1109/LRA.2021.3063074.
[3] J. Nogueira, R. Martinez-Cantin, A. Bernardino and L. Jamone, "Unscented Bayesian optimization for safe robot grasping," 2016 IEEE/RSJ International Conference on Intelligent Robots and Systems (IROS), Daejeon, Korea (South), 2016, pp. 1967-1972, doi: 10.1109/IROS.2016.7759310.
Visual Key Point Extraction and Mesh Reconstruction of Deformable Objects
Scope: Master thesis
Advisor: Berk Gueler and Alap Kshirsagar
Added: 2024-10-22
Start: ASAP
Topic:
Understanding and manipulating deformable objects is a critical challenge in robotics and simulation, as these objects often exhibit complex, non-linear behavior. This thesis focuses on extracting key points from visual feedback (RGB or RGB-D) of deformable objects and reconstructing them using graph neural networks (GNNs). The goal is to develop a method to parse key points from visual data, encode this data in a latent space, and then reconstruct the object’s mesh structure in simulation using the extracted key points. The proposed method would enable efficient encoding-decoding processes, potentially improving the handling and manipulation of deformable objects in real-world scenarios.
The tasks involved in this thesis include:
- Development of a simulation environment for deformable object manipulation and visualization.
- Design and implementation of key point extraction methods using visual feedback (RGB/RGB-D).
- Application of graph neural networks for encoding key points into a latent space.
- Reconstruction of object meshes in the simulation using the extracted latent space representations.
- Evaluation of the proposed method through comparison with existing approaches in terms of accuracy and computational efficiency.
To apply, please send an email with a letter of motivation and your CV to berk.gueler@tu-darmstadt.de and alap.kshirsagar@tu-darmstadt.de
Desired Qualifications
- Strong Python programming skills
- Experience with deep learning frameworks (PyTorch/TensorFlow).
- Prior experience with graph neural networks or gaussian splatting is preferred.
- Optional: Familiarity with simulation frameworks (e.g., PyBullet, MuJoCo, Unity) or 3D modeling libraries.
References
[1] A. Choi, R. Jing, A. Sabelhaus, et al."DisMech: A Discrete Differential Geometry-Based Physical Simulator for Soft Robots and Structures." IEEE Robotics and Automation, 2024
[2] Jad Abou-Chakra, Krishan Rana, Feras Dayoub, Niko Sünderhauf "Physically Embodied Gaussian Splatting: A Realtime Correctable World Model for Robotics" arXiv preprint arXiv:1609.02907 (2024).
[3] Wang, Yue, et al. "Dynamic graph CNN for learning on point clouds." ACM Transactions on Graphics 38.5 (2019): 1-12.
Robot Learning for Dynamic Motor Skills: A Case Study with Paper Planes
Scope: Master thesis
Advisor: Alap Kshirsagar and Kai Ploeger
Added: 2024-09-05
Start: ASAP
Topic:
Developing dynamic motor skills in robots is crucial for various complex manipulation tasks. This thesis explores robot learning for dynamic motor skills using the challenging task of accurately throwing paper planes at targets. Throwing a paper plane involves both gross and fine motor skills, making it an interesting problem for robot learning. The goal is to enable robots to learn this task autonomously, adapting to various paper-plane properties and environmental conditions. We will explore reinforcement learning [1, 2, 3] and domain randomization for this purpose. The thesis tasks will involve:
- Development of a simulation environment for a robotic paper plane-throwing scenario
- Design and implementation of a reinforcement learning pipeline for accurate paper plane throwing
- Evaluation of the approach in simulation and on a physical robot platform
To apply, please send an email with a letter of motivation and your CV to alap.kshirsagar@tu-darmstadt.de and kai.ploeger@tu-darmstadt.de
Desired Qualifications
- Strong Python programming skills
- Prior experience with simulation frameworks (MuJoCo/Gym)
- Optional: Prior experience with Robot Operating System
References
[1] Obayashi, Chihiro, Tomoya Tamei, and Tomohiro Shibata. "Assist-as-needed robotic trainer based on reinforcement learning and its application to dart-throwing." *Neural Networks* 53 (2014): 52-60.
[2] Zeng, Andy, et al. "Tossingbot: Learning to throw arbitrary objects with residual physics." *IEEE Transactions on Robotics* 36.4 (2020): 1307-1319.
[3] Kang, Yeong-Gyun, and Cheol-Soo Lee. "Deep Reinforcement Learning of Ball Throwing Robot's Policy Prediction." *The Journal of Korea Robotics Society* 15.4 (2020): 398-403.
Autonomous Robotic Knot Untangling
Scope: Master thesis
Advisor: Berk Gueler and Kay Hansel
Added: 2024-10-10
Start: ASAP
Topic:
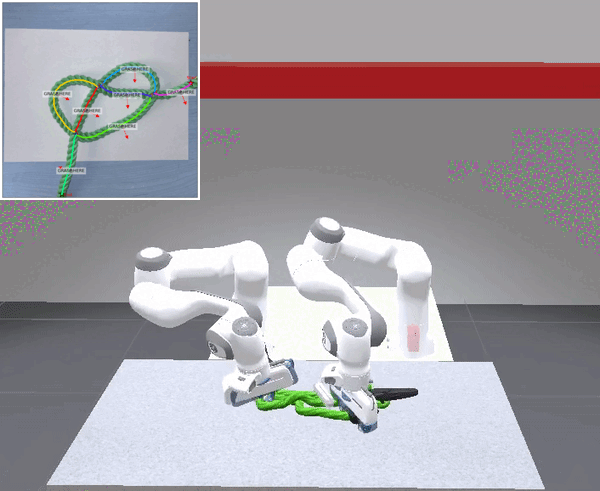
Untangling deformable linear objects (DLOs) such as ropes and cables is a challenging task in robotics due to their complex dynamics and sensitivity to external forces. In real-world applications like surgical procedures, cable harness assembly, and household chores, autonomous DLO manipulation could be highly beneficial. However, estimating their state and planning effective manipulation strategies remain difficult. Recent advancements in computer vision have improved DLO tracing and modeling, with systems like RT-DLO enhancing detection through advanced image segmentation and cable tracing. However, these methods still struggle with occlusions and complex knots. As the field of computer vision continues to evolve, incorporating machine learning models to better estimate rope states and manipulation actions is becoming more feasible. For instance, HANDLOOM [2] and SGTM2.0 [3] combine both manipulation (predefined primitive movements) and cable tracing algorithms to perform DLO manipulation. However, there is significant room for improvement, especially in developing faster solutions capable of handling dynamic, unforeseen situations and a wide variety of DLO manipulations.
This thesis aims to develop a fully autonomous system for rope untangling, leveraging visual recognition and data-driven DLO manipulation techniques, and evaluating it in both simulated and real-world environments.
Requirements
- Strong Python programming skills
- Knowledge in Machine Learning / Supervised Learning
- Experience with deep learning libraries is a plus
Interested students can apply by sending an e-mail to berk.gueler@ias.tu-darmstadt.de and attaching the documents mentioned below:
- Curriculum Vitae
- Motivation letter explaining why you would like to work on this topic and why you are the perfect candidate
References
[1] A. Caporali, K. Galassi, B. L. Zagar, R. Zanella, G. Palli, and A. C. Knoll, “RT-DLO: Real-time deformable linear objects instance segmentation,” IEEE Transactions on Industrial Informatics, vol. 19, no. 11, pp. 11333–11342, 2023
[2] K. Shivakumar, V. Viswanath, A. Gu, Y. Avigal, J. Kerr, J. Ichnowski, R. Cheng, T. Kollar, and K. Goldberg, “SGTM 2.0: Autonomously Untangling Long Cables using Interactive Perception,” Sept. 2022
[3] V. Viswanath, K. Shivakumar, M. Parulekar, J. Ajmera, J. Kerr, J. Ichnowski, R. Cheng, T. Kollar, and K. Goldberg, “HANDLOOM: Learned Tracing of One-Dimensional Objects for Inspection and Manipulation,” 2023
Blending Deep Generative Models using Stochastic Optimization
Scope: Master thesis
Advisor: Kay Hansel and Niklas Funk
Added: 2024-07-26
Start: ASAP
Topic: This Master Thesis aims to explore the blending of deep generative models using stochastic optimization techniques [1], focusing on reactive motion generation for robotics. The research will encompass the training of deep generative models, such as Score-based [2], or Flow-based models, specifically utilizing the JAX framework for efficient computation. A significant part of the thesis will involve deriving a mixture of experts algorithm, which leverages these trained generative models in combination with other manually specified objectives to enhance the performance of the motion generator. This integration aims to create more adaptive and responsive robotic behaviors in dynamic environments, offering a substantial advancement over existing methods.
Requirements
- Strong Python programming skills
- Knowledge in Machine Learning
- Experience with deep learning libraries and JAX is a plus
Interested students can apply by sending an e-mail to kay.hansel@tu-darmstadt.de and attaching the documents mentioned below:
- Curriculum Vitae
- Motivation letter explaining why you would like to work on this topic and why you are the perfect candidate
References
[1] Hansel, K.; Urain, J.; Peters, J.; Chalvatzaki, G. (2023). Hierarchical Policy Blending as Inference for Reactive Robot Control, 2023 IEEE International Conference on Robotics and Automation (ICRA), IEEE.
[2] Urain, J.; Funk, N.; Peters, J.; Chalvatzaki G (2023). SE(3)-DiffusionFields: Learning smooth cost functions for joint grasp and motion optimization through diffusion, International Conference on Robotics and Automation (ICRA).
Self-supervised learning of a visual object-centric representation for robotic manipulation
Scope: External Master Thesis 🇫🇷
This master thesis will be conducted with our French partners at Ecole Centrale de Lyon. Possibility of ERASMUS scholarship.
Advisor: Alexandre Chapin, Liming Chen, Emmanuel Dellandrea
Added: 2024-07-15
Start: ASAP
Topic:
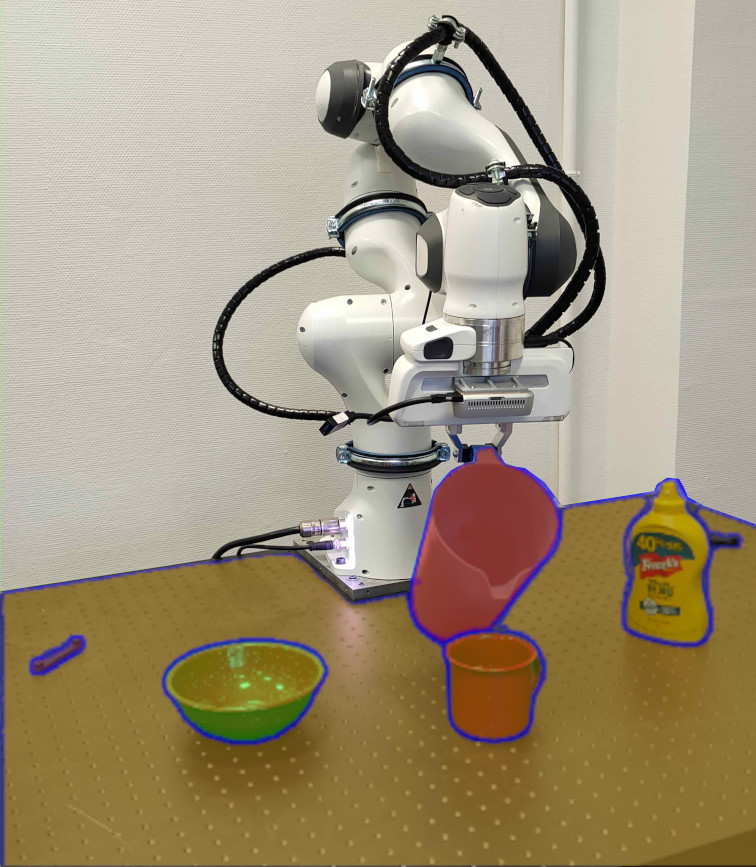
Vision-based learning for robotic manipulation often relies on holistic visual scene representations, where the environment is depicted as a single vector. This method is suboptimal for handling diverse scenes and objects in unconstrained environments. Better representations can improve generalization and data efficiency in robotic learning [1]. Inspired by human perception, object-centric representation has been developed to represent environments with multiple vectors, each corresponding to an object's properties [2]. However, these methods mainly use synthetic datasets [3, 4, 5] and struggle with real-world scenarios [6]. With advances in self-supervised learning for vision models [7, 8], which show promise for object discovery, we propose pre-training an object-centric representation using self-supervised methods to scale to real-world scenarios. This thesis will focus on: Developing and training an object-centric self-supervised model on a real-world dataset. Pre-training the model on a real-world robotic dataset. Applying the pre-trained model to visual-based robotic manipulation tasks.
Interested students can apply by sending the required documents to alexandre.chapin@ec-lyon.fr and attaching the required documents mentioned below.
Requirements
- Strong Python programming skills
- Experience with the Pytorch library
Preferred Qualifications
- Prior experience in Computer Vision and/or Robotics is preferred
- Use of distributed environment for learning of models (SLURM)
- Knowledge on recent self-supervised learning methods for vision [7, 8]
Required Documents
- Curriculum Vitae
- Motivation letter explaining why you would like to work on this topic and why you are the perfect candidate
References
[1] O. Kroemer et al. “A review of robot learning for manipulation: Challenges, representations, and algorithms” (2019)
[2] F. Locatello et al. “Object-centric learning with Slot Attention” (2020)
[3] G. Singh et al. “Illiterate DALL-E learns to compose” (2021)
[4] T. Kipf et al. “Conditional object-centric learning from video” (2022)
[5] G. Singh et al. “Simple Unsupervised Object-Centric Learning for Complex and Naturalistic Videos” (2022)
[6] Z. Wu et al. “SlotDiffusion: Object-Centric Generative Modeling with Diffusion Models” (2023)
[7] M. Caron et al. “Emerging Properties in Self-Supervised Vision Transformers” (2021)
[8] O. J. Hénaff et al. “Object discovery and representation networks” (2022)
Walk your network: investigating neural network’s location in Q-learning methods.
Scope: Master thesis
Advisor: Theo Vincent
Start: Flexible
Topic:
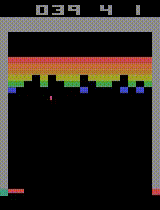
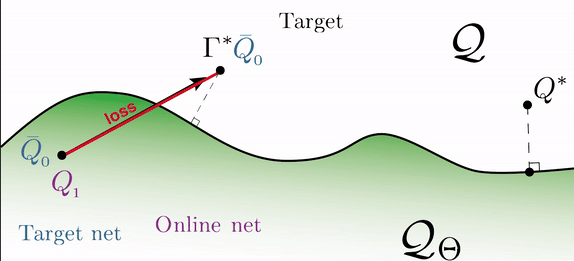
Q-learning methods are at the heart of Reinforcement Learning. They have been shown to outperform humans on some complex tasks such as playing video games [1]. In robotics, where the action space is in most cases continuous, actor-critic methods are relying on Q-learning methods to learn the critic [2]. Although Q-learning methods have been extensively studied in the past, little focus has been placed on the way the online neural network is exploring the space of Q functions. Most approaches focus on crafting a loss that would make the agent learn better policies [3]. Here, we offer a thesis that focuses on the position of the online Q neural network in the space of Q functions. The student will first investigate this idea on simple problems before comparing the performance to strong baselines such as DQN or REM [1, 4] on Atari games. Depending on the result, the student might as well get into MuJoCo and compare the results with SAC [2]. The student will be welcome to propose some ideas as well.
Highly motivated students can apply by sending an email to theovincentjourdat@gmail.com. Please attach your CV, a grade sheet and clearly state why you are interested in this topic. Students who have followed the Reinforcement Learning or Robot Learning course will be prioritized.
Requirements
- Strong Python programming skills
- Knowledge in Reinforcement Learning
- Experience with deep learning libraries is a plus
References
[1] Mnih, Volodymyr, et al. "Human-level control through deep reinforcement learning." nature 518.7540 (2015): 529-533.
[2] Haarnoja, Tuomas, et al. "Soft actor-critic: Off-policy maximum entropy deep reinforcement learning with a stochastic actor." International conference on machine learning. PMLR, 2018.
[3] Hessel, Matteo, et al. "Rainbow: Combining improvements in deep reinforcement learning." Proceedings of the AAAI conference on artificial intelligence. Vol. 32. No. 1. 2018.
[4] Agarwal, R., Schuurmans, D. & Norouzi, M.. (2020). An Optimistic Perspective on Offline Reinforcement Learning International Conference on Machine Learning (ICML).
Co-optimizing Hand and Action for Robotic Grasping of Deformable objects
Scope: Master thesis
Advisor: Alap Kshirsagar, Guillaume Duret
Added: 2024-01-15
Start: ASAP
Topic:
The current standard approach to robotic manipulation involves distinct stages of manipulator design and control. However, the interdependence of a robot gripper's morphology and control suggests that jointly optimizing these aspects can significantly enhance performance. Existing methods for such a co-optimization [1] are limited to rigid objects whereas manipulation of deformable objects is critical for several real-world applications such as food handling and robotic surgery.
This project aims to advance deformable object manipulation by co-optimizing robot gripper morphology and control policies. The project will involve utilizing existing simulation environments for deformable object manipulation [2] and implementing a method to jointly optimize gripper morphology and grasp policies within the simulation.
Required Qualification:
- Strong Python programming skills
- Familiarity with deep learning libraries such as PyTorch or Tensorflow
Preferred Qualification:
- Attendance of the lectures "Statistical Machine Learning", "Computational Engineering and Robotics" and "Robot Learning"
Application Requirements:
- Curriculum Vitae
- Motivation letter explaining why you would like to work on this topic and why you are the perfect candidate
Interested students can apply by sending an e-mail to alap.kshirsagar@tu-darmstadt.de and attaching the required documents mentioned above.
References:
[1] Xu, Jie, et al. "An End-to-End Differentiable Framework for Contact-Aware Robot Design." Robotics: Science & Systems. 2021.
[2] Huang, Isabella, et al. "DefGraspNets: Grasp Planning on 3D Fields with Graph Neural Nets." arXiv preprint arXiv:2303.16138 (2023).
Geometry-Aware Diffusion Models for Robotics
Scope: Master thesis
Advisor: Joao Carvalho, An Thai Le
Added: 2023-11-20
Start: ASAP
Topic:
Diffusion-based generative models have shown increasing performance in image generation [1], and, more recently, in reinforcement learning and robotics.
E.g., in motion planning [2], grasping [3], imitation learning [4], and offline reinforcement learning [5].
Two important properties of diffusion models that we are interested in exploring are their ability to encode multimodal demonstrations and combine gradients of multiple costs (as in optimization-based motion planning).
In this thesis, you will work on developing an imitation learning algorithm using diffusion models for robotic manipulation tasks, such as the ones in [2, 3, 4], but taking into account the geometry of the task space.
If this sounds interesting, please send an email to joao@robot-learning.de and an@robot-learning.de, and possibly attach your CV, highlighting the relevant courses you took in robotics and machine learning.
What's in it for you:
- You get to work on an exciting topic at the intersection of deep-learning and robotics
- We will supervise you closely throughout your thesis
- Depending on the results, we will aim for an international conference publication
Requirements:
- Be motivated -- we will support you a lot, but we expect you to contribute a lot too
- Robotics knowledge
- Experience setting up deep learning pipelines -- from data collection, architecture design, training, and evaluation
- PyTorch -- especially experience writing good parallelizable code (i.e., runs fast in the GPU)
References:
[1] https://arxiv.org/abs/2112.10752
[2] https://arxiv.org/abs/2308.01557
[3] https://arxiv.org/abs/2209.03855
[4] https://arxiv.org/abs/2303.04137
[5] https://arxiv.org/abs/2205.09991
Learning Latent Representations for Embodied Agents
Scope: Master Thesis
Advisor: Michael Drolet, Oleg Arenz
Added: 2023-11-05
Start: ASAP
Topic:
Learning from Demonstration [1] and Policy Search [2] are fundamental approaches in training robot policies, but there's a critical challenge: choosing the right expert. Typically, experts are expected to operate within the same state space and dynamics as the robot to facilitate learning within a specific environment. While this approach can yield desirable results, it limits us to a single expert, often overlooking valuable insights from experts with different dynamics and actuators. This is where your thesis steps in.. Our goal is harness the collective power of experts with diverse embodiments. We seek to create a more comprehensive representation of prior experiences by identifying common attributes in a lower-dimensional latent space. You will play a crucial role in discovering efficient encoding and decoding techniques for this latent space, ensuring it can be applied to various robot platforms. Your work will involve mastering dimensionality reduction, imitation learning, and the transfer of robot skills. While prior experience in these areas is a bonus, we welcome anyone with a passion for robotics and sufficient qualifications as described below.
Interested students can apply by sending an E-Mail to michael.drolet@tu-darmstadt.de and attaching the required documents mentioned below.
Required Qualification:
- Strong Python programming skills
- Experience with TensorFlow/PyTorch
- Familiarity with core Machine Learning topics
Preferred Qualification:
- Experience programming/controlling robots (either simulated or real world)
- Knowledgeable about different robot platforms (quadrupeds and bipedal robots)
Application Requirements:
- Resume / CV
- Cover letter explaining why this topic fits you well and why you are an ideal candidate
References:
[1] Ho and Ermon. "Generative adversarial imitation learning"
[2] Arenz, et al. "Efficient Gradient-Free Variational Inference using Policy Search"
Timing is Key: CPGs for regularizing Quadruped Gaits learned with DRL
Scope: Master thesis
Advisor: Nico Bohlinger, Davide Tateo
Added: 2023-10-20
Start: ASAP
Topic:
Current model-free Deep Reinforcement Learning (DRL) approaches for quadruped locomotion can learn highly agile locomotion (like parkour [1]), while being far more flexible than model-based or optimal control approaches. But to achieve a natural-looking gait they rely on complex reward functions with up to 12 or more reward terms and heavy tuning of their coefficients. This tuning is quite fragile and often has to be redone for different robots or new environments.
To tackle this problem we want to utilize Central Pattern Generators (CPGs), which can generate timings for ground contacts for the four feet. The policy gets rewarded for complying with the contact patterns of the CPGs. This leads to a straightforward way of regularizing and steering the policy to a natural gait without posing too strong restrictions on it. We first want to manually find fitting CPG parameters for different gait velocities and later move to learning those parameters in an end-to-end fashion.
Highly motivated students can apply by sending an E-Mail to nico.bohlinger@tu-darmstadt.de and attaching the required documents mentioned below.
Minimum Qualification:
- Good Python programming skills
- Basic knowledge of the PyTorch library
- Basic knowledge of Reinforcement Learning
Preferred Qualification:
- Good knowledge of the PyTorch library
- Basic knowledge of the MuJoCo simulator
Application Requirements:
- Curriculum Vitae
- Motivation letter explaining why you would like to work on this topic and why you are the perfect candidate
References:
[1] Cheng, Xuxin, et al. "Extreme Parkour with Legged Robots."
Damage-aware Reinforcement Learning for Deformable and Fragile Objects
Scope: Master thesis
Advisor: Guillaume Duret, Tim Schneider
Added: 2023-10-16
Start: ASAP
Topic:
Dealing with soft or fragile objects introduces a host of challenges that surpass traditional rigid object manipulation, such as deformability and the risk of damage.
Tasks in which we have to deal with such objects include, for example, squeezing a mustard bottle or picking up fragile fruits without causing damage.
In this thesis we will tackle this problem with model-based reinforcement learning by using existing models to predict stress and deformability of objects.
Goal of this thesis will be the development and application of a model-based reinforcement learning method on real robots.
Your tasks will include:
1. Setting up a simulation environment for deformable object manipulation
2. Utilizing existing models for stress and deformability prediction[1]
3. Implementing a reinforcement learning method to work in simulation and, if possible, on the real robot methods.
If you are interested in this thesis topic and believe you possess the necessary skills and qualifications, please submit your application, including a resume and a brief motivation letter explaining your interest and relevant experience. Please send your application to guillaume.duret@ec-lyon.fr.
Required Qualification:
- Enthusiasm for and experience in robotics, machine learning, and simulation
- Strong programming skills in Python
- Familiarity with deep learning libraries such as PyTorch or Tensorflow
Desired Qualification:
- Attendance of the lectures "Statistical Machine Learning", "Computational Engineering and Robotics" and (optionally) "Robot Learning"
References:
[1] Huang, I., Narang, Y., Bajcsy, R., Ramos, F., Hermans, T., & Fox, D. (2023). DefGraspNets: Grasp Planning on 3D Fields with Graph Neural Nets. arXiv preprint arXiv:2303.16138.
Geometry-Aware Diffusion Models for Robotics
Scope: Master thesis
Advisor: Joao Carvalho, An Thai Le
Added: 2023-10-09
Start: ASAP
Topic:
Diffusion-based generative models have shown increasing performance in image generation [1], and, more recently, in reinforcement learning and robotics.
E.g., in motion planning [2], grasping [3], imitation learning [4], and offline reinforcement learning [5].
Two important properties of diffusion models that we are interested in exploring are their ability to encode multimodal demonstrations and combine gradients of multiple costs (as in optimization-based motion planning).
In this thesis, you will work on developing an imitation learning algorithm using diffusion models for robotic manipulation tasks, such as the ones in [2, 3, 4], but taking into account the geometry of the task space.
If this sounds interesting, please send an email to joao@robot-learning.de and an@robot-learning.de, and possibly attach your CV, highlighting the relevant courses you took in robotics and machine learning.
What's in it for you:
- You get to work on an exciting topic at the intersection of deep-learning and robotics
- We will supervise you closely throughout your thesis
- Depending on the results, we will aim for an international conference publication
Requirements:
- Be extremely motivated -- we will support you a lot, but we expect you to contribute a lot too
- Robotics knowledge
- Experience setting up deep learning pipelines -- from data collection, architecture design, training, and evaluation
- PyTorch -- especially experience writing good parallelizable code (i.e., runs fast in the GPU)
References:
[1] https://arxiv.org/abs/2112.10752
[2] https://arxiv.org/abs/2308.01557
[3] https://arxiv.org/abs/2209.03855
[4] https://arxiv.org/abs/2303.04137
[5] https://arxiv.org/abs/2205.09991
Tactile Sensing for the Real World
Scope: Master thesis
Advisor: Theo Gruner, Daniel Palenicek, and Tim Schneider
Start: ASAP
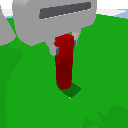
Topic: Tactile sensing is a crucial sensing modality that allows humans to perform dexterous manipulation[1]. In recent years, the development of artificial tactile sensors has made substantial progress, with current models relying on cameras inside the fingertips to extract information about the points of contact [2]. However, robotic tactile sensing is still a largely unsolved topic despite these developments. A central challenge of tactile sensing is the extraction of usable representations of sensor readings, especially since these generally contain an incomplete view of the environment.
Recent model-based reinforcement learning methods like Dreamer [3] leverage latent state-space models to reason about the environment from partial and noisy observations. However, more work has yet to be done to apply such methods to real-world manipulation tasks. Hence, this thesis will explore whether Dreamer can solve challenging real-world manipulation tasks by leveraging tactile information. Initial results suggest that tasks like peg-in-a-hole can indeed be solved with Dreamer in simulation (see figure above), but the applicability of this method in the real world has yet to be shown.
In this work, you will work with state-of-the-art hardware and compute resources on a hot research topic with the option of publishing your work at a scientific conference.
Highly motivated students can apply by sending an email to theo_sunao.gruner@tu-darmstadt.de. Please attach a transcript of records and clearly state your prior experiences and why you are interested in this topic.
Requirements
- Strong Python programming skills
- Ideally experience with deep learning libraries like JAX or PyTorch
- Experience with reinforcement learning is a plus
- Experience with Linux
References
[1] 2S Match Anest2, Roland Johansson Lab (2005), https://www.youtube.com/watch?v=HH6QD0MgqDQ
[2] Gelsight Inc., Gelsight Mini, https://www.gelsight.com/gelsightmini/
[3] Hafner, D., Lillicrap, T., Ba, J., & Norouzi, M. (2019). Dream to control: Learning behaviors by latent imagination. arXiv preprint arXiv:1912.01603.
Deep Learning Meets Teleoperation: Constructing Learnable and Stable Inductive Guidance for Shared Control
Scope: Master thesis
Advisor: Kay Hansel, An Thai Le
Added: 2023-06-14
Start: July / August 2023
Topic:
Teleoperation is one of the biggest challenges in robotics [1]. It allows us to bypass human physical limitations and more rapidly and efficiently distribute skills and hands-on expertise to distant geographic locations where they are needed. However, transferring skills and hands-on expertise to robots in remote, sometimes dangerous environments must meet specific requirements, e.g., high precision and safety. Additional difficulties, such as communication delays and partial observability, complicate the transfer. Therefore, prior work introduced assistive policies that guide the user while execution, also known as shared control [2]. However, most of these policies are task-specific, manually created, or lack properties such as stability.
This work considers policies as learnable inductive guidance for shared control. In particular, we use the class of Riemannian motion policies [3] and consider them as differentiable optimization layers [4]. We analyze (i) if RMPs can be pre-trained by learning from demonstrations [5] or reinforcement learning [6] given a specific context; (ii) and subsequently employed seamlessly for human-guided teleoperation thanks to their physically consistent properties, such as stability [3]. We believe this step eliminates the laborious process of constructing complex policies and leads to improved and generalizable shared control architectures.
Highly motivated students can apply by sending an e-mail expressing your interest to kay.hansel@tu-darmstadt.de and an.le@tu-darmstadt.de, attaching your letter of motivation and possibly your CV.
Requirements:
- Strong Python programming skills
- Experience with deep learning libraries (in particular Pytorch)
- Knowledge in reinforcement learning and/or machine learning
References:
[1] Niemeyer, Günter, et al. "Telerobotics." Springer handbook of robotics (2016);
[2] Selvaggio, Mario, et al. "Autonomy in physical human-robot interaction: A brief survey." IEEE RAL (2021);
[3] Cheng, Ching-An, et al. "RMP flow: A Computational Graph for Automatic Motion Policy Generation." Springer (2020);
[4] Jaquier, Noémie, et al. "Learning to sequence and blend robot skills via differentiable optimization." IEEE RAL (2022);
[5] Mukadam, Mustafa, et al. "Riemannian motion policy fusion through learnable lyapunov function reshaping." CoRL (2020);
[6] Xie, Mandy, et al. "Neural geometric fabrics: Efficiently learning high-dimensional policies from demonstration." CoRL (2023).
Dynamic symphony: Seamless human-robot collaboration through hierarchical policy blending
Scope: Master thesis
Advisor: Kay Hansel, Berk Gueler
Added: 2023-06-14
Start: July / August 2023
Topic:
Teleoperation is one of the biggest challenges in robotics [1]. It allows us to bypass human physical limitations and more rapidly and efficiently distribute skills and hands-on expertise to distant geographic locations where they are needed. However, transferring skills and hands-on expertise to robots in remote, sometimes dangerous environments must meet specific requirements, e.g., high precision and safety. Additional difficulties, such as communication delays and partial observability, complicate the transfer. Therefore, previous work introduced assistive policies that guide the user during execution, also known as shared control, and studied how to arbitrate between user and autonomy [2].
This work focuses on arbitration between the user and assistive policy, i.e., shared autonomy. Various works allow the user to influence the dynamic behavior explicitly and, therefore, could not satisfy stability guarantees [3]. We pursue the idea of formulating arbitration as a trajectory-tracking problem that implicitly considers the user's desired behavior as an objective [4]. Therefore, we extend the work of Hansel et al. [5], who employed probabilistic inference for policy blending in robot motion control. The proposed method corresponds to a sampling-based online planner that superposes reactive policies given a predefined objective. This method enables the user to implicitly influence the behavior without injecting energy into the system, thus satisfying stability properties. We believe this step leads to an alternative view of shared autonomy with an improved and generalizable framework.
Highly motivated students can apply by sending an e-mail expressing your interest to kay.hansel@tu-darmstadt.de or berk.gueler@tu-darmstadt.de, attaching your letter of motivation and possibly your CV.
Requirements:
- Strong Python programming skills
- Experience with deep learning libraries (in particular Pytorch)
- Knowledge in reinforcement learning and/or machine learning
References:
[1] Niemeyer, Günter, et al. "Telerobotics." Springer handbook of robotics (2016);
[2] Selvaggio, Mario, et al. "Autonomy in physical human-robot interaction: A brief survey." IEEE RAL (2021);
[3] Dragan, Anca D., and Siddhartha S. Srinivasa. "A policy-blending formalism for shared control." IJRR (2013);
[4] Javdani, Shervin, et al. "Shared autonomy via hindsight optimization for teleoperation and teaming." IJRR (2018);
[5] Hansel, Kay, et al. "Hierarchical Policy Blending as Inference for Reactive Robot Control." IEEE ICRA (2023).
Feeling the Heat: Igniting Matches via Tactile Sensing and Human Demonstrations
Scope: Master thesis
Advisor: Tim Schneider Niklas Funk
Added: 2023-03-27
Start: April / May 2023
Topic:
Humans heavily rely on tactile sensing for a range of dynamic tasks, such as igniting matches, catching balls, and shuffling cards.
Yet, there still only exist few works that augment robotic systems with a sense of touch.
In this thesis we want to employ tactile sensors for solving the highly dynamic task of igniting matches.
This task has been of great interest to understand the importance of tactile sensing in humans [1] which makes it a great candidate for studying robot tactile sensing.
In this thesis, we want to investigate the effectiveness of vision-based tactile sensors for solving dynamic tasks (igniting matches). Since the whole task is difficult to simulate, we directly collect real-world data to learn policies from the human demonstrations [2,3]. We believe that this work is an important step towards more advanced tactile skills.
Highly motivated students can apply by sending an e-mail expressing your interest to niklas.funk@tu-darmstadt.de and tim.schneider1@tu-darmstadt.de, attaching your letter of motivation and possibly your CV.
Requirements:
- Good knowledge of Python
- Experience with deep learning libraries (in particular Pytorch)
- Prior experience with real robots and Linux is a plus
References:
[1] https://www.youtube.com/watch?v=HH6QD0MgqDQ
[2] Learning Compliant Manipulation through Kinesthetic and Tactile Human-Robot Interaction; Klas Kronander and Aude Billard.
[3] https://www.youtube.com/watch?v=jAtNvfPrKH8
Robotic Tactile Exploratory Procedures for Identifying Object Properties
Scope: Master's thesis
Advisor: Tim Schneider, Alap Kshirsagar
Added: 2022-09-06
Start: ASAP
Topic:
Identifying properties such as shape, deformability, roughness etc. is important for successful manipulation of an object. Humans use specific “exploratory procedures (EPs)” [1] to identify object properties, for example, lateral motion to detect texture and pressure to detect deformability. Our goal is to understand whether these exploratory procedures are optimal for robotic arms equipped with tactile sensors. We specifically focus on three properties and their corresponding EPs: texture (lateral motion), shape (contour following) and deformability (pressure).
Goals of the thesis
- Literature review of robotic EPs for identifying object properties [2,3,4]
- Develop and implement robotic EPs for a Digit tactile sensor
- Compare performance of robotic EPs with human EPs
Desired Qualifications
- Interested in working with real robotic systems
- Python programming skills
Literature
[1] Lederman and Klatzky, “Haptic perception: a tutorial”
[2] Seminara et al., “Active Haptic Perception in Robots: A Review”
[3] Chu et al., “Using robotic exploratory procedures to learn the meaning of haptic adjectives”
[4] Kerzel et al., “Neuro-Robotic Haptic Object Classification by Active Exploration on a Novel Dataset”
Long-Horizon Manipulation Tasks from Visual Imitation Learning (LHMT-VIL): Algorithm
Scope: Master thesis
Advisor: Suman Pal, Ravi Prakash, Vignesh Prasad, Aiswarya Menon
Added: 2022-06-16
Start: Immediately
Topic: The objective of this thesis is to create a method to solve long-horizon robot manipulation tasks from Visual Imitation Learning (VIL). Thus, given a video demonstration of a human performing a long horizon manipulation task such as an assembly sequence, a robot should imitate the identical task by analyzing the video.
The proposed architecture can be broken down into the following sub-tasks:
1. Multi-object 6D pose estimation from video: Identify the object 6D poses in each video frame to generate the object trajectories
2. Action segmentation from video: Classify the action being performed in each video frame
3. High-level task representation learning: Learn the sequence of robotic movement primitives with the associated object poses such that the robot completes the demonstrated task
4. Low-level movement primitives: Create a database of low-level robotic movement primitives which can be sequenced to solve the long-horizon task
Desired Qualification:
1. Strong Python programming skills
2. Prior experience in Computer Vision and/or Robotics is preferred
Long-Horizon Manipulation Tasks from Visual Imitation Learning (LHMT-VIL): Dataset
Scope: Master thesis
Advisor: Suman Pal, Ravi Prakash, Vignesh Prasad, Aiswarya Menon
Added: 2022-06-16
Start: Immediately
Topic: The objective of this thesis is to create a large-scale dataset to solve long-horizon robot manipulation tasks from Visual Imitation Learning (VIL). Thus, given a video demonstration of a human performing a long horizon manipulation task such as an assembly sequence, a robot should imitate the identical task by analyzing the video.
During the project, we will create a large-scale dataset of videos of humans demonstrating industrial assembly sequences. The dataset will contain information of the 6D poses of the objects, the hand and body poses of the human, the action sequences among numerous other features. The dataset will be open-sourced to encourage further research on VIL.
Desired Qualification:
1. Strong Python programming skills
2. Prior experience in Computer Vision and/or Robotics is preferred
[1] F. Sener, et al. "Assembly101: A Large-Scale Multi-View Video Dataset for Understanding Procedural Activities". CVPR 2022.
[2] P. Sharma, et al. "Multiple Interactions Made Easy (MIME) : Large Scale Demonstrations Data for Imitation." CoRL, 2018.