Daniel Palenicek
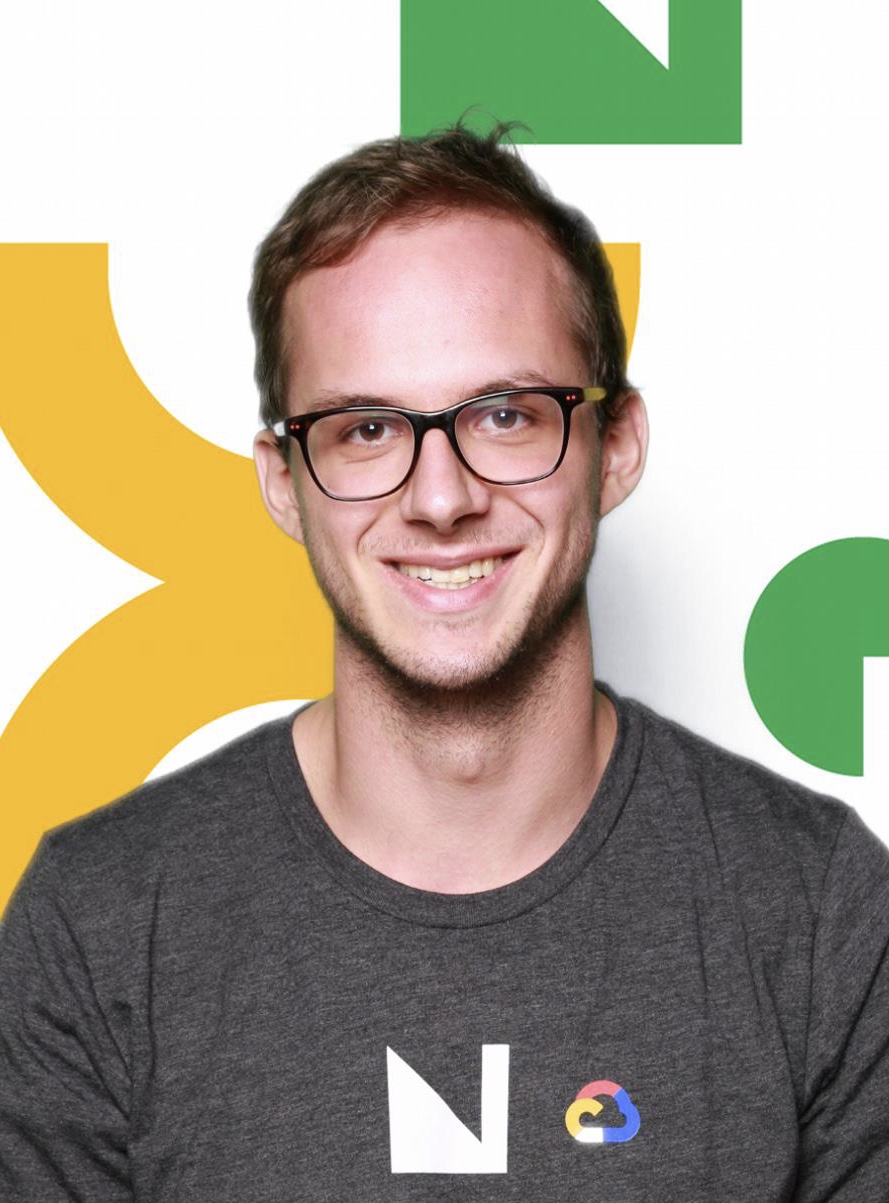
Research Interests
- Reinforcement Learning
- Model-based Reinforcement Learning
- Machine Learning
Affiliations
- TU Darmstadt, Intelligent Autonomous Systems, Computer Science Department
- Hessian Centre for Artificial Intelligence
Contact Information
daniel.palenicek@tu-darmstadt.de
Room E304, Building S2|02, TU Darmstadt, FB-Informatik, FG-IAS, Hochschulstr. 10, 64289 Darmstadt
+49-6151-16-25387
Reviewing
NeurIPS, ICLR, CoRL, RLC (Senior Reviewer), IROS, and various ML & Robotics workshops.
Teaching Assistant
Lecture | Years |
CE and Robotics | SS 2022, WS 2022/23 |
Statistical Machine Learning | SS 2023, WS 2023/24 |
Probabilistic Methods in CS | WS 2024/25 |
Robot Learning Integrated Project 1 & 2 | SS 2025 |
Daniel joined the Intelligent Autonomous System lab on October 1st, 2021 as a Ph.D. student. He is part of the 3AI project with Hessian.AI. In his research, Daniel focuses on increasing sample efficiency of model-based reinforcement learning algorithms by studying the impact which model-errors have on the learning.
Before starting his Ph.D., Daniel completed his Bachelor's degree and Master's degree in Wirtschaftsinformatik at the Technische Universität Darmstadt. He wrote his Master's thesis entitled "Dyna-Style Model-Based Reinforcement Learning with Value Expansion" in the Computer Science Department under the supervision of Michael Lutter and Jan Peters. During his studies, Daniel further did two research internships, at the Bosch Center for AI and at Huawei Noah’s Ark Lab London.
Publications
- Palenicek, D.; Lutter, M.; Carvalho, J.; Dennert, D.; Ahmad, F.; Peters, J. (submitted). Diminishing Return of Value Expansion Methods, Submitted to the IEEE Transaction on Pattern Analysis and Machine Intelligence (PAMI).
- Palenicek, D.; Vogt, F.; Watson, J.; Peters, J. (submitted). Scaling Off-Policy Reinforcement Learning with Batch and Weight Normalization, Under review.
- Vincent, T.; Palenicek, D.; Belousov, B.; Peters, J.; D'Eramo, C. (2025). Iterated Q-Network: Beyond One-Step Bellman Updates in Deep Reinforcement Learning, Transactions on Machine Learning Research (TMLR).
- Toelle, M.; Gruner, T.; Palenicek, D.; Schneider, T. Guenster, J.; Watson, J.; Tateo, D.; Liu, P.; Peters, J. (2025). Towards Safe Robot Foundation Models using Inductive Biases, SafeVLM Workshop @ IEEE International Conference on Robotics and Automation (ICRA), [Spotlight].
- Palenicek, D.; Vogt, F.; Peters, J. (2025). Scaling CrossQ with Weight Normalization, Multi-disciplinary Conference on Reinforcement Learning and Decision Making (RLDM).
- Lenz, J.; Pfenning, I.; Gruner, T.; Palenicek, D.; Schneider, T.; Peters, J. (2025). Exploring the Role of Vision and Touch in Reinforcement Learning for Dexterous Insertion Tasks, Multi-disciplinary Conference on Reinforcement Learning and Decision Making (RLDM).
- Celik, O.; Li, Z.; Blessing, D.; Li, G.; Palenicek, D.; Peters, J.; Chalvatzaki, G.; Neumann, G. (2025). Diffusion-Based Maximum Entropy Reinforcement Learning, 7th Robot Learning Workshop: Towards Robots with Human-Level Abilities.
- Scherer, C. F.; Tölle, M.; Gruner, T.; Palenicek, D.; Schneider, T.; Schramowski, P.; Belousov, B.; Peters, J. (2025). AllmAN: A German Vision-Language-Action Model, German Robotics Conference (GRC).
- Toelle, M.; Gruner, T.; Palenicek, D.; Guenster, J.; Liu, P.; Watson, J.; Tateo, D.; Peters, J. (2025). Towards Safe Robot Foundation Models, German Robotics Conference (GRC).
- Bohlinger, N.; Kinzel, J.; Palenicek, D.; Antczak, L.; Peters, J. (2025). Gait in Eight: Efficient On-Robot Learning for Omnidirectional Quadruped Locomotion, International Conference on Intelligent Robots and Systems (IROS).
- Celik, O.; Li, Z.; Blessing, D.; Li, G.; Palenicek, D.; Peters, J.; Chalvatzaki, G.; Neumann, G. (2025). DIME: Diffusion-Based Maximum Entropy Reinforcement Learning, International Conference on Machine Learning (ICML).
- Celik, O.; Li, Z.; Blessing, D.; Li, G.; Palenicek, D.; Peters, J.; Chalvatzaki, G.; Neumann, G. (2025). DIME: Diffusion-Based Maximum Entropy Reinforcement Learning, EXAIT Workshop at International Conference on Machine Learning (ICML).
- Bhatt, A.; Palenicek, D.; Belousov, B.; Argus, M.; Amiranashvili, A.; Brox, T.; Peters, J. (2024). CrossQ: Batch Normalization in Deep Reinforcement Learning for Greater Sample Efficiency and Simplicity, International Conference on Learning Representations (ICLR), [Spotlight].
- Palenicek, D.; Gruner, T.; Schneider, T.; Böhm, A.; Lenz, J.; Pfenning, I. and Krämer, E.; Peters, J. (2024). Learning Tactile Insertion in the Real World, IEEE ICRA 2024 Workshop on Robot Embodiment through Visuo-Tactile Perception.
- Palenicek, D.; Gruner, T.; Schneider, T.; Böhm, A.; Lenz, J.; Pfenning, I. and Krämer, E.; Peters, J. (2024). Learning Tactile Insertion in the Real World, 40th Anniversary of the IEEE International Conference on Robotics and Automation (ICRA@40).
- Bhatt, A.; Palenicek, D.; Belousov, B.; Argus, M.; Amiranashvili, A.; Brox, T.; Peters, J. (2024). CrossQ: Batch Normalization in Deep Reinforcement Learning for Greater Sample Efficiency and Simplicity, European Workshop on Reinforcement Learning (EWRL).
- Lenz, J.; Gruner, T.; Palenicek, D.; Schneider, T.; Pfenning, I.; Peters J. (2024). Analysing the Interplay of Vision and Touch for Dexterous Insertion Tasks, CoRL 2024 Workshop on Learning Robot Fine and Dexterous Manipulation: Perception and Control.
- Palenicek, D.; Lutter, M.; Carvalho, J.; Peters, J. (2023). Diminishing Return of Value Expansion Methods in Model-Based Reinforcement Learning, International Conference on Learning Representations (ICLR).
- Gruner, T.; Belousov, B.; Muratore, F.; Palenicek, D.; Peters, J. (2023). Pseudo-Likelihood Inference, Advances in Neural Information Processing Systems (NIPS / NeurIPS).
- Cowen-Rivers, A.I.; Palenicek, D.; Moens, V.; Abdullah, M.A.; Sootla, A.; Wang, J.; Bou-Ammar, H. (2022). SAMBA: safe model-based & active reinforcement learning, Machine Learning.
- Palenicek, D.; Lutter, M., Peters, J. (2022). Revisiting Model-based Value Expansion, Multi-disciplinary Conference on Reinforcement Learning and Decision Making (RLDM).
- Palenicek, D. (2021). A Survey on Constraining Policy Updates Using the KL Divergence, Reinforcement Learning Algorithms: Analysis and Applications, pp.49-57.
- Zhou, M.; ...; Palenicek, D; ... (2020). SMARTS: Scalable Multi-Agent Reinforcement Learning Training School for Autonomous Driving, Conference on Robot Learning (CoRL), Best System Paper Award.
Talks and Interviews
- Sample Efficiency in Deep RL: Quo Vadis? @ BeNeRL Seminar Series (2024)
- CrossQ: Batch Normalization in Deep Reinforcement Learning for Greater Sample Efficiency and Simplicity @ Machine Learning and AI Academy (2024)
- AI and its faces, Daniel Palenicek & Theo Gruner, PhD students @hessian.AI (2023)
- Slurm-tastic Adventures on the IAS Cluster: A Roboticist Guide to Using the IAS Cluster with Slurm and Docker @ IWIALS (2023)
Supervised Theses and Projects
Thesis/Project | Topic | Student(s) | Together with |
M.Sc. Thesis | Investigating bottlenecks of CrossQ's sample efficiency | Vogt F. | |
M.Sc. Thesis | On-robot Deep Reinforcement Learning for Quadruped Locomotion | Kinzel J. | Nico Bohlinger |
B.Sc. Thesis | Diminishing Return of Value Expansion Methods in Offline Model-Based Reinforcement Learning | Dennert D. | |
B.Sc. Thesis | Diminishing Return of Value Expansion Methods in Discrete Model-Based Reinforcement Learning | Ahmad F. | |
RL:IP.SS24 | Training Large Scale Robot Transformer Models | Scherer C. | Tim Schneider & Theo Gruner & Maximilian Tölle |
RL:IP.SS24 | XXX: eXploring X-Embodiment with RT-X | Jacobs T. | Tim Schneider & Theo Gruner & Maximilian Tölle |
RL:IP.SS24 | Unveiling the Unseen: Tactile Perception and Reinforcement Learning in the Real World | Böhm A., Krämer E. | Tim Schneider & Theo Gruner |
RL:IP.WS23/24 | XXX: eXploring X-Embodiment with RT-X | Dennert D., Scherer C., Ahmad F. | Tim Schneider & Theo Gruner & Maximilian Tölle |
RL:IP.WS23/24 | XXX: eXploring X-Embodiment with RT-X | Jacobs T. | Tim Schneider & Theo Gruner & Maximilian Tölle |
RL:IP.WS23/24 | Unveiling the Unseen: Tactile Perception and Reinforcement Learning in the Real World | Böhm A., Pfenning I., Lenz J. | Tim Schneider & Theo Gruner |
RL:IP.SS23 | Latent Tactile Representations for Model-based RL | Krämer E. | Tim Schneider & Theo Gruner |