Projects
We are partners in several projects. Naturally, this list is somewhat incomplete as only major funded projects are being listed.
ARISE (EU; 2024-2028)
ARISE (Advanced AI and RobotIcS for autonomous task pErformance) aims to introduce a combination of perception and control modules around a reconfigurable robotic manipulator that will enable a step change in the level of automation of complex manipulation tasks. ARISE will comprise the key novel technology components that will significantly push the state of the art in terms of automatic task segmentation, human robot interaction and complex manipulation. Within ARISE we will develop next-generation Hierarchical IL and Human Interaction-conditioned task planning.
Contacts: Kay Hansel,Niklas Funk, Jan Peters
LokoAssist (DFG; 2023-2026)
Within the comprehensive LokoAssist project, our focus centers on the development of advanced control mechanisms for robot prostheses. In particular, our branch of the project is dedicated to leveraging machine learning, generative models, and imitation learning techniques to synthesize control signals that result in a natural and visually appealing gait. Recognizing the potential of active movement assistance systems, our research also aims to integrate these technologies into the body schema by identifying diverse movement intentions. Through the application of data-driven learning methods, we are laying the groundwork for generating motor behavior that aligns intuitively with users' expectations. Specifically, our work focuses on the development of models that draw from data obtained through user demonstrations, allowing for the creation of control signals that enhance the adaptability, comfort, and natural appearance of the gait. These initiatives align with the broader mission of maximizing the effectiveness of assistance systems.
Project Website: https://www.tu-darmstadt.de/lokoassist
Contacts: Michael Drolet, Jan Peters
INTENTION (NCN-DFG; 2023-2026)
The INTENTION project is a collaboration between the Poznan University of Technology and the Technische Universität Darmstadt. This project aims to fill the gap between human-made systems and their biological counterparts. The goal of this project is to innovate in terms of perception, control, and learning to provide a solid basis for physical intelligence. We aim at the quadruped robot that can perceive and perform agile locomotion in unstructured, confined, and dynamic environments, switch between different modes of locomotion, and improve performance over time. The use of non-visual sensing is at the core of our research as our target is to sense through actuators using force-transparent motors and proprioception. These will allow our robot to go beyond human sensing and utilize non-visual data for interpretation and decision making. To increase the physical intelligence of the robot we consider a design with a flexible spine. Up to now, this feature is common in biological creatures but is rarely considered in legged robots. We want to exploit this extra degree of freedom to increase the robot’s agility and sensing capabilities by fully exploiting whole-body contacts, allowing the platform to face challenging environments.
Project Website: http://intention.robot-learning.net
Contacts: Nico Bohlinger, Davide Tateo, Jan Peters
IntuitivePhsyics (DFG; 2023-2026)
Within the DFG funded project "Informed Exploration in Reinforcement Learning via Intuitive Physics Model Reasoning" we investigate how we can leveraging high-level dynamic models, specifically intuitive physics models (IPMs), to enhance the adaptability of robots in real-world scenarios. The primary goal is to explore how these models can autonomously discover and learn critical environmental characteristics, contributing to more effective exploration in RL. By creating abstract and adaptable representations of the environment, our research aims to improve the generalization of RL across various environmental factors. The project's evaluation emphasizes critical research questions concerning the utility of high-level dynamic models for informed exploration and the potential for enhancing the practical deployment of autonomous robots in dynamic real-world environments.
Contacts: Oleg Arenz, Jan Peters
ETL4Balance (BMBF; 2023-2026)
In the context of cloud-based data logistics, ETL (Extract, Transform, Load) systems encounter challenges related to variable demands and operational disruptions. Traditional methods necessitate frequent human intervention, limiting continuous functionality. The ETL4Balance project, a collaborative effort between TU Darmstadt and Deepshore with BMBF support, aims to address these issues. By leveraging reinforcement learning, the project seeks to replace conventional ETL system operations with AI-driven, autonomous load and capacity management. This approach is designed to enable ETL systems to proactively allocate resources, and respond to real-time disruptions, contributing to the evolution of more efficient and self-regulated data logistics in virtual infrastructures.
Contacts: Oleg Arenz, Jan Peters
3rd Wave of AI (HMWK; 2021-2025)
Within 3AI, we aim at developing the next generation of AI systems. Pushing the limits of hybrid and neuro-symbolic AI, these AI systems will acquire human-like communication and thinking abilities, recognize and classify new situations, and adapt to them autonomously. Through 3AI, the systemic and algorithmic foundations of what we call “Systems AI” will be developed. Akin to Systems Biology, interactions of different AI building blocks will be mathematically and algorithmically correctly captured, understood, and used, and new methods of system design, e.g., software engineering or data management for Systems AI, will be explored.
Contacts: Daniel Palenicek, Theo Gruner, Jan Peters
The Adaptive Mind (HMWK; 2021-2025)
The Adaptive Mind One of the most critical challenges facing any organism is to maintain stability in the face of a dynamic and uncertain world. At the same time, successful behavior also rests crucially on our ability to adapt when circumstances fundamentally change. These two conflicting demands present a major dilemma: to determine when variability is noise that must be suppressed, and when it is signal that requires adjusting our behavior to the ‘new normal’. Understanding how we resolve this Stability-Transition dilemma is central to understanding the adaptive mind and is one of the most significant open questions in science. It occurs at every level of human thought and behavior—from low-level sensory adaptation to long-term changes when practicing advanced skills. Resolving the stability-transition dilemma is crucial to survival: it defines our success, and its failure may cause mental disorder. In The Adaptive Mind, we combine rigorous behavioral research methods and theories from experimental psychology, with the unique patient-oriented insights of psychiatry and clinical psychology and the power of quantitative analysis and computational modeling by artificial intelligence. Our goal is to observe empirically, describe quantitatively, and model computationally how the human mind continually adapts in an ever-changing and often unpredictable world. By examining stability and transition, we seek to characterize one of the canonical computations of the human mind—adaptation—that links basic sensory and motor processes with high-level aspects of cognition and behavior across the entire lifespan, in both healthy individuals and those suffering from paradigmatic mental disorders. To do so, we will create a collaborative research program with five tightly interwoven Key Areas (Dynamics, Context, Interaction, Skills and Disorder), and a Data Hub for mining and sharing data, to investigate the adaptive mind as it performs complex natural behaviors. Only by integrating insights and methods from empirical researchers, theoreticians and clinicians can we develop a comprehensive understanding of the adaptive mind.
Contacts: Alap Kshirsagar, Jan Peters
WHITEBOX (LOEWE; 2021-2025)
Until a few years ago, intelligent systems such as robots and digital voice assistants had to be tailored towards narrow and specific tasks and contexts. Such systems needed to be programmed and fine tuned by experts. But, recent developments in artificial intelligence have led to a paradigm shift: instead of explicitly representing knowledge about all information processing steps at time of development, machines are endowed with the ability to learn. With the help of machine learning it is possible to leverage large amounts of data samples, which hopefully transfer to new situations via pattern matching. Groundbreaking achievements in performance have been obtained over the last years with deep neural networks, whose functionality is inspired by the structure of the human brain. A large number of artificial neurons interconnected and organized in layers process input data under large computational costs. Although experts understand the inner working of such systems, as they have designed the learning algorithms, often they are not able to explain or predict the system’s intelligent behavior due to its complexity. Such systems end up as blackboxes raising the question of how such systems’ decisions can be understood and trusted. Our basic hypothesis is that explaining an artificial intelligence system may not be fundamentally different from the task of explaining intelligent goal-directed behavior in humans. Behavior of a biological agent is also based on the information processing of a large number of neurons within brains and acquired experience. But, an explanation based on a complete wiring diagram of the brain and all its interactions with its environment may not provide an understandable explanation. Instead, explanations of intelligent behavior need to reside at a computationally more abstract level: they need to be cognitive explanations. Such explanations are developed in computational cognitive science. Thus, WhiteBox aims at transforming blackbox models into developing whitebox models through cognitive explanations that are interpretable and understandable. Following our basic assumption, we will systematically develop and compare whitebox and blackbox models for artificial intelligence and human behavior. In order to quantify the differences between these models, we will not only develop novel blackbox and whitebox models, but also generate methods for the quantitative and interpretable comparison between these models. Particularly, we will develop new methodologies to generate explanations automatically by means of AI. As an example, deep blackbox models comprise deep neural networks whereas whitebox models can be probabilistic generative models with explicit and interpretable latent variables. Application of these techniques to intelligent goal directed human behavior will provide better computational explanations of human intelligent behavior as well as allow to transfer human level behavior to machines.
Contacts: Kai Ploeger, Jan Peters
SKILLS4ROBOTS (2015-2021; ERC Starting Grant)
The goal of SKILLS4ROBOTS is to develop an autonomous skill learning system that enables humanoid robots to acquire and improve a rich set of motor skills. This robot skill learning system will allow scaling of motor abilities up to fully anthropomorphic robots while overcoming the current limitations of skill learning systems to only few degrees of freedom. To achieve this goal, it will decompose complex motor skills into simpler elemental movements - called movement primitives - that serve as building blocks for the higher-level movement strategy and the resulting architecture will be able to address arbitrary, highly complex tasks -- up to robot table tennis for a humanoid robot. Learned primitives will be superimposed, sequenced and blended. For example, a game of robot table tennis can be represented using different stroke movement primitives, such as a forehand stroke, a backhand stroke or a smash, as well as locomotion primitives for foot placement for maintaining balance by shifting the center of mass of the robot.
The resulting decomposition into building blocks is not only inherent to many motor tasks but also highly scalable and will be exploited by our learning system. Four recent breakthroughs in our research will make this project possible due to successes on the representation of the parametric probabilistic representations of the elementary movements, on probabilistic imitation learning, on relative entropy policy search-based reinforcement learning and on the modular organization of the representation.
These breakthroughs will allow create a general, autonomous skill learning system that can learn many different skills in the exact same framework without changing a single line of programmed code.
Contacts: Jan Peters, Boris Belousov, Hany Abdulsamad
CHIRON (2021-2023; ANR-DFG-JSPS)
To make possible such an embodied tele-operated robotic system for dexterous manipulation, thus without any assumption about the object to be manipulated and the operating environment, the CHIRON project features the unique innovations simultaneously on robotics, computer vision and machine learning. Specifically, the CHIRON project will make use of a dual-arm robot and aims to bring breakthroughs in the following research topics: compliant grippers with tactile feedback, deep understanding of the scene, reinforcement learning-based shared robot control, intuitive and effective haptic interface for the embodiment of the dual-arm robot, and the last and not the least few shot learning given the very limited amount of data, e.g., trials that the envisaged system can afford with the physical ability limited human operator, for the training of the deep scene analysis and shared robot control so that the embodied dual-arm robot easily to adapt to novel operator for complex never seen before object manipulation in rapidly changing environments. As such, the CHIRON project fits perfectly the objectives of this trilateral call for proposals on AI, specifically in “advancing the state of the art in AI in order to accomplish complex tasks”; and “allowing high-level interactions with human users” and contributing in core AI technologies. The key components required by the CHIRON project are covered with the unique symbiosis of the respective world class expertise of each partner. Prof.Hasegawa’s group from Japan brings its unique expertise in assistive robotics with embodiment for augmented human physical skills, e.g., extra robotic thumb, intelligent cane for elderly, exoskeleton. Prof. Peters’ team from Germany is providing their well known rich experience in robotc manipulation, including tactile sensing, robotic tele-operation, learning by demonstration, and reinforcement learning. Prof. Chen’s group from France is bringing their confirmed expertise in computer vision and machine learning for deep understanding of the scene for object manipulation.
Contacts: Kay Hansel, Jan Peters
METRIC4IMITATION (2021-2024; DFG Project)
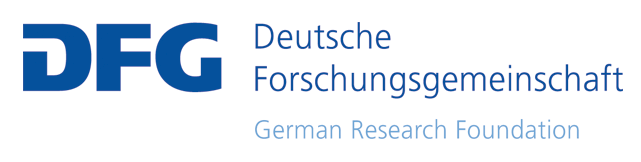
aspect - with behavior, it perceives in another agent (the expert or demonstrator) given that the two agents have different kinematics and dynamics (body morphology, degrees of freedom, constraints, joints, and actuators, torque limits), or in other words, cover different state spaces?
The goal of this project is to use a metric understanding of embodiment to improve robotic motor skills through expert observations. We aim to shed light on important fundamental research questions on the (i) role of learner’s embodiment in statistical imitation learning, (ii) how the correspondence problem can be formalized properly, (iii) how the behavior transferability vs task complexity dilemma can be resolved, and (iv) how to develop new statistical deep imitation learning algorithms based on these insights. We will evaluate these methodological advances by performing imitation learning between robots having different embodiments for goal-conditioned tasks such as manipulations and unconditional tasks such as dancing. The derived algorithms have to deal with embodiment mismatch to facilitate imitation learning.
Contacts: An Thai Le, Jan Peters
ARISTOTLE (2021-2023; ANR-BMBF)
The Aristotle project aims to develop an AI-empowered general-purpose robotic system for dexterous bi-manual manipulation utilizing multimodal information from visual and tactile sensors. This will be achieved by combining novel representations with skill learning methods for learning to manipulate complex objects of different physical properties, as well as for abstracting and decomposing challenging bi-manipulation tasks into internal simpler ones. As humans, we rely heavily on bi-manual manipulation in our everyday life. A service robot needs this capacity to be able to evolve in our unconstrained environment (e.g., houses, factories, hospitals) and to perform tasks efficiently even when confronted with unknown objects or unfamiliar situations. The target application domain for the Aristotle project is maintenance and repair, an area that requires bi-manual manipulation by the essence of the tasks (e.g., opening cans, assembling parts, screwing light bulbs).
Contacts: Tim Schneider, Jan Peters
DeepWalking (2021-2024; DFG Project)
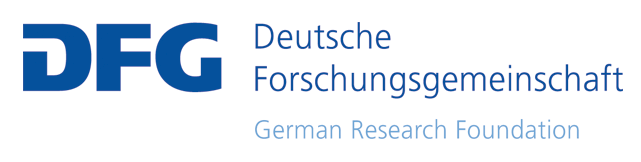
A dynamic model capable of generating rich human walking behaviors, including responses to unexpected perturbations, provides a powerful framework for developing state-of-the-art control schemes for human assistive devices (e.g. exosuits), prostheses, and bipedal robots. Developing such a model is challenging as it requires capturing the complexities of human neuromuscular gait control, which is not well understood. Within the DeepWalking Project, we will use inverse reinforcement learning and deep neural networks to deduce and understand the human's underlying reward signal, and optimize the latter to extract the required sensory-motor mappings to generate human-like locomotion behaviors at kinematic, kinetic, and muscle levels. This sensory-motor mapping not only allows us to generate and understand human locomotion in simulation but also enables research in controlling exoskeletons.
Contacts: Firas Al-Hafez, Jan Peters
AICO - Artificial Intelligence In Construction (2020-2024; Industry Project)
Architecture and the building industry face enormous challenges against climate change, resource scarcity, and lack of skilled labor. The building industry consumes approximately 40% of global resources and energy, 50% of global waste comes from construction. Productivity in the building sector has been stagnating for 30 years. These challenges are now being approached by computational design, digital fabrication, and robotic assembly. Digitally prefabricated modular construction systems are a promising way to improve construction. Computational design tools and digital fabrication allow for mass customization and circular re-use, which is essential for a versatile and livable built environment. However, the robotic assembly of those modules on site, under messy and unpredictable circumstances, is still an unsolved problem.
The AICO project faces this challenge and aims to develop efficient learning-based approaches for modular assembly of architectural structures. One of the key challenges is the integration of visual, tactile, and other sensing modalities within the motor skill learning loop. Therefore, the project studies different parameterizations of control policies, including combined task and motion planning within a single learnable graph neural network. The developed methods should enable robots at construction sites to perform assembly tasks that involve contact-rich manipulation of modules that vary in material, scale, weight, and function. This project is a collaboration with Nexplore.
Contacts: Niklas Funk, Jan Peters
ROBOLEAP (2018-2021; DFG Project)
The goal of the ROBOLEAP (Robot learning to perceive, plan, and act under uncertainty) project is to develop reinforcement learning methods that allow robots to operate in unstructured partially observable real world environments found in household robotics, adaptive manufacturing, elderly care, handling dangerous materials, or even disaster scenarios such as Fukujima. Robots that can operate in such complex environments need data-driven reinforcement learning methods that can take uncertainty due to partial observability into account. To make reinforcement learning in partially observable robotic tasks feasible we will develop new memory representations which allow us to efficiently reuse experience with different kinds of policies. To enable long-term action selection, we will improve exploration and value propagation over long horizons: under partial observability the robot needs to execute information gathering actions which requires uncovering and propagating values over long horizons during policy optimization. Moreover, in partially observable settings the problem of assigning values to actions is amplified. To solve this problem we will give the robot additional side information during learning.
We will evaluate these methodological advances by endowing a real robot with the ability to play Mikado, a task that exhibits all the main difficulties connected to partial observability. The robot has to deal with occlusions and partial information. It has to proactively test physical properties of Mikado sticks and integrate this knowledge into its manipulation skills to remove sticks from the heap.
Contacts: Joni Pajarinen, Tuan Dam, Pascal Klink
SHAREWORK (2018-2022; EU H2020 RIA)
SHAREWORK‘s main objective is to endow an industrial work environment of the necessary »intelligence« and methods for the effective adoption of Human Robot Collaboration (HRC) with not fences, providing a system capable of understanding the environment and human actions through knowledge and sensors, future state predictions and with the ability to make a robot act accordingly while human safety is guaranteed and the human-related barriers are overcome. SHAREWORK will develop the needed technology for facing the new production paradigm compiling the necessary developments in a set of modular hardware, software and procedures to face different HRC applications in a systematic and effective way. A knowledge base (KB) to include system »know-how« data as well as real-time environment information is developed. An environment run-time perception and cognition updates this KB with object detection, human tracking and task identification. A human-aware dynamic task planning system will react based on previous knowledge and environment status by reassigning tasks and/or reconfiguring robot control. This data will allow robot intelligent motion planners to control robots while safety is ensured by a continuous ergonomics and risk assessment module to face a safetyproductivity trade-off. A multimodal human-robot communication system will provide interfaces for bidirectional communication between operator and robot. Finally, methods for overcoming human-related barriers and data reliability and security concerning the entire framework are applied for a successful integration in the industry. SHAREWORK technology will be demonstrated in four different industrial cases: for railway, automotive, mechanical machining and equipment goods sectors. The usability of the developed HRC solutions in different industrial sectors and company sizes will increase productivity, flexibility, and reduce human stress, to support the workers and to strengthen European industry.
Contacts: Tianyu Ren, Julen Urain de Jesus
GOAL-Robots (2017-2020; EU H2020 FET)
This project aims to develop a new paradigm to build open-ended learning robots called Goal-based
Openended Autonomous Learning (GOAL). GOAL rests upon two key insights. First, to exhibit an autonomous
open-ended learning process, robots should be able to self-generate goals, and hence tasks to practice.
Second, new learning algorithms can leverage self-generated goals to dramatically accelerate skill learning.
The new paradigm will allow robots to acquire a large repertoire of flexible skills in conditions
unforeseeable at design time with little human intervention, and then to exploit these skills to efficiently
solve new user-defined tasks with no/little additional learning. This innovation will be essential in the design
of future service robots addressing pressing societal needs. The project will develop the GOAL paradigm by
pursuing three main objectives: (1) advance our understanding of how goals are formed and underlie skill
learning in children; (2) develop innovative computational architectures and algorithms supporting (2a) the
self-generation of useful goals based on user/task independent mechanisms such as intrinsic motivations, and
(2b) the use of such goals to efficiently and autonomously build large repertoires of skills; (3) demonstrate
the potential of GOAL with a series of increasingly challenging demonstrators in which robots will
autonomously develop complex skills and use them to solve difficult challenges in real-life scenarios. The
iterdisciplinary project consortium is formed by leading international roboticists, computational modelers,
and developmental psychologists working with complementary approaches. This will allow the project to
greatly advance our understanding of the fundamental principles of open-ended learning and to produce a
breakthrough in the field of autonomous robotics by producing for the first time robots that can
autonomously accumulate complex skills and knowledge in a truly open-ended way.
Contacts: Elmar Rueckert, Daniel Tanneberg, Svenja Stark, Jan Peters
Motor Dreaming (2017-2021; Industry Project)
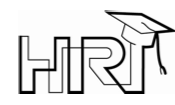
The use of internal physical models allows creating training data without a large number of real-world explorations. Such a reduction of the dependency on real-world samples alleviates one of the key problems of Reinforcement Learning: complex problems require a large number of explorations which rapidly becomes unmanageable when moving to a larger dimensionality. The usage of internal simulations allows creating big data, and creating training input with a large number of variations / parameter distributions. The results opens the door to learning approaches that rely on large amounts of data. This project is a collaboration with Michael Gienger and his team at Honda Research Institute at Offenbach, Germany.
Contacts: Fabio Muratore, Jan Peters
Reinforcement Learning for Robot Manipulation (2017-2020; Industry Project)
Machine learning and artificial intelligence have made important and substantial progress in recent years. By now, they are reaching large scale applications in industrial environments. Such machine learning methods increasingly enable unforeseen development of new applications of technical systems and robots. Many large companies, such as Google, Baidu and Microsoft, are heavily investing into integrating such technology in their products and providing a new, better user experience. While perception tasks -- such as hearing and seeing -- have been particularly difficult for machines for a long time, we have seen an enormous improvement in this field thanks to machine learning in recent years. For example, by using techniques from the field of "deep learning", machines are already reaching and overtaking human performance for specific tasks (e.g., skin cancer recognition).

In this research project, the required work for a Ph.D. thesis in reinforcement learning in the context of industrial robotics is to be pursued. This topic may well change the role of robots in many manufacturing processes. Instead of being manually programmed to carry out the same task millions of times, future robots would be enabled to adapt to hundreds of different tasks autonomously and could become useful for small series production. The proposed research will develop reinforcement learning methods for more complex robot activities enabling as well as interaction with humans. The Ph.D. project is funded by the BOSCH FORSCHUNGSSTIFTUNG (Bosch Research Foundation).
Contacts: Samuele Tosatto, Jan Peters
KoBo34 (2018-2021; BMBF Project)
The overall objective of the KoBo34 project is to contribute to improving the social participation of elderly people and maintaining their independence with a humanoid service robot that will be developed in KoBo34. The determination of useful activities to be supported by robotics technology and the technical design of these options at the cutting edge of today's scientific knowledge and technical possibilities are central to the project, as well as the processing and consideration of the acceptance requirements and the comprehensive evaluation of the Implementation. In close collaboration with the center for cognitive science the IAS research within KoBO34 focusses on "Intentional recognition and interaction learning", which is the (mutual) recognition and coordination of the movement and action intentions of human and robot. Here, "physical" interaction and communication between robot and human, e.g. physical feedback through touch or motion gestures, as well as the haptic, interactive training of complex procedures with non-expert users is an interesting and challenging research aspect.
Contacts: Dorothea Koert
LearnRobotS (2015-2018; DFG Project, SPP Autonomous Learning)
The goal of this project is to develop a hierarchical learning system that decomposes complex motor skills into simpler elemental movements, also called movement primitives, that serve as building blocks of our movement strategy. For example, in a tennis game, such primitives can represent different tennis strokes such as a forehand stroke, a backhand stroke or a smash. As we can see, the autonomous decomposition into building blocks is inherent to many motor tasks. In this project, we want to exploit this basic structure for our learning system. To do so, our autonomous learning system has to extract the movement primitives out of observed trajectories, learn to generalize the primitives to different situations and select between, sequence or combine the movement primitives such that complex behavior can be synthesized out of the primitive building blocks. Our autonomous learning system will be applicable to learning from demonstrations as well as subsequent self improvement by reinforcement learning. Learning will take place on several layers of the hierarchy. While on the upper level, the activation policy of different primitives will be learned, the intermediate level of the hierarchy extracts meta-parameters of the primitives and autonomously learns how to adapt these parameters to the current situation. The lowest level of the hierarchy learns the control policies of the single primitives. Learning on all layers as well as the extraction of the structure of the hierarchical policy is aimed to operate with a minimal amount of dependence from a human expert. We will evaluate our autonomous learning framework on a robot table tennis platform, which will give us many insights in the hierarchical structure of complex motor tasks.
Contacts: Riad Akrour, Sebastian Gomez, Gerhard Neumann, Jan Peters
SCARL (2015-2018; DFG Project, SPP Autonomous Learning)
Over the course of the last decade, the framework of reinforcement learning (RL) has developed into a promising tool for learning a large variety of different tasks in robotics. During this timeframe, a lot of progress has been made towards scaling reinforcement learning to high-dimensional systems and solving tasks of increasing complexity. Unfortunately, this scalability has been achieved by using expert knowledge to pre-structure the learning problem in several dimensions. As a consequence, the state-of-the-art methods in robot reinforcement learning generally depend on hand-crafted state representations, pre-structured parametrized policies, well-shaped reward functions and demonstrations by a human expert to aid scaling of the learning algorithm. This large amount of required pre-structuring arguably is in stark contrast to the goal of developing autonomous reinforcement learning systems.
In this project, we want to advance the field by starting with a 'classical' reinforcement learning setting for a challenging robotic task (i.e., tetherball). Solving this task by RL methods will be already a valuable contribution. From there on, we will start to identify the components for which the learning task design still needs engineering experience. In the course of this project, we show how we aim to drive each of these components towards more autonomy while developing highly scalable approaches.
Contacts: Simone Parisi, Christian Daniel, Jan Peters
ROMANS (2015-2018; EU H2020 RIA)
The RoMaNS (Robotic Manipulation for Nuclear Sort and Segregation) project will advance the state of the art in mixed
autonomy for tele-manipulation, to solve a challenging and safety-critical “sort and segregate” industrial problem, driven by
urgent market and societal needs.
Cleaning up the past half century of nuclear waste represents the largest environmental remediation project in the whole of Europe. Nuclear waste must be “sorted and segregated”, so that low-level contaminated waste is placed in low-level storage containers, rather than occupying extremely expensive and resource intensive high-level storage containers and facilities. Many older nuclear sites (>60 years
in UK) contain large numbers of legacy storage containers, some of which have contents of mixed contamination levels, and
sometimes unknown contents.
Several million of these legacy waste containers must now be cut open, investigated, and their contents sorted. This can
only be done remotely using robots, because of the high levels of radioactive material. Current state-of-the-art practice in the
industry, consists of simple tele-operation (e.g. by joystick or teach-pendant). Such an approach is not viable in the long-
term, because it is prohibitively slow for processing the vast quantity of material required.
The project will:
1) Develop novel hardware and software solutions for advanced bi-lateral master-slave tele-operation.
2) Develop advanced autonomy methods for highly adaptive automatic grasping and manipulation actions.
3) Combine autonomy and tele-operation methods using state-of-the-art understanding of mixed initiative planning, variable
autonomy and shared control approaches.
Contacts: Takayuki Osa, Joni Pajarinen, Gregor Gebhardt, Oleg Arenz, Gerhard Neumann, Jan Peters
BIMROB (2014-2017; FiF Project)
The BIMROB project is funded by the Forum for Interdisciplinary Research (FiF) at the Technische Universität Darmstadt (Technical University of Darmstadt). The main focus of this project is the scientific investigation of the interaction between humans and robots when learning movements. Efficient and effective interaction configurations will be determined in order to allow humans and robots to acquire new movements or improve their performance at certain tasks that involve their sensorial and motor capabilities. BIMROB comprises four work packages. The investigation of the joint acquisition/improvement of movements by humans and robots will be developed in the scenario of learning to putt a golf ball. Each of the work packages corresponds to a type of interaction: (1) A human learns a movement from another human. (2) A robot learns a movement from a human. (3) A human learns a movement from a robot. (4) A human and a robot learn a movement together. The results of this project may become important for applications ranging from sport training devices to rehabilitation.
Contacts: Marco Ewerton, Gerrit Kollegger, Josef Wiemeyer, Jan Peters
TACMAN (2014-2017; EU FP7 STREP)
TACMAN addresses the key problem of developing an information processing and control technology enabling robot hands to exploit tactile sensitivity and thus become as dexterous as human hands. The current availability of the required technology now allows us to considerably advance in-hand manipulation. TACMAN’s goal is to develop fundamentally new approaches which can replace manual labor under inhumane conditions by endowing robots with such tactile manipulation abilities, by transferring insights from human neuroscientific studies into machine learning algorithms.
TACMAN will provide an innovative new technology that is key for bringing industrial manufacturing back to Europe. Consider the case of the iPhone, where most mechanical manipulation of the major components is achieved by manual human
labor under terrible work conditions and not by advanced industrial robots—despite that millions of iPhones are industrially assembled per month. The reason for this absence of appropriate automation is the lack of manipulation skills of current robots.
Commercially available robotic hand-arm systems move more accurately and faster than humans, and their sensors
see more and at a higher precision—even the smallest forces and torques can be detected. Despite these impressive sensori-motor abilities, current robots are terrible at manipulation when compared to humans. Neuro- science provides a clear reason for the superiority of human hands: During manipulation, humans make substantial use of the data from tactile sensors, i.e., the information obtained through the feeling in the human’s fingers. Robot hands are lacking this key ability! Hence, the rationale of TACMAN is that this performance gap in manipulation ability can be filled by (1) making such tactile sensory comprehensible, and (2) use the information provided by such sensors intelligently for behavior generation. TACMAN aims to integrate the most robust available tactile sensors into the control of existing modern robot hands, and, based on this control law, develop tactile sensor-based manipulation solutions. To make this innovation tractable in a three year project, we aim only on recognising and handling objects that are already in the hand. The structure of the project
is designed to allow quick scaling from straightforward, well-captured scenarios employing a single finger to complex multi-fingered manipulation.
Contacts: Elmar Rueckert, Herke van Hoof, Filipe Veiga, Daniel Tanneberg, Jan Peters
Learning Sequential Skills for Robot Manipulation Tasks (2014-2017; Industry Project)
Robot manipulation is commonly conceived as a high-potential future business area due to the numerous potential applications. Among them are factory assembly, medical applications, service robotics, offshore robotics, disaster robot applications and others. This project will create new concepts and techniques for robot learning of manipulation skills from a human teacher. In recent and current work, we are investigating movement representations and learning of simple movements, which we represent in so called Movement Primitives. The particular focus of this joint project with the Honda Research Institute at Offenbach, Germany, is to learn the coordination of such primitives, in order to realize complex sequential and parallel movement behaviour. An illustrative example is the replacement of a light bulb: The robot’s movement skill can be composed of elementary primitives, such as reaching towards the lamp, aligning the fingers with the bulb, grasping the bulb or turning it in the thread. The sequential skill is coordinating these primitives with a flexible arbitration scheme: It needs to maintain the causal order of the primitives (e.g. reach – pre-shape – grasp), while coordinating the timing of primitives that are active in parallel (co-articulation of left and right hand for bi-manual skills). In case of larger disturbances, the skill needs to adapt the sequential flow to account for the changed situation (e.g. pick up bulb if it drops out of the hand). This project is a collaboration with Michael Gienger and his team at Honda Research Institute at Offenbach, Germany.
Contacts: Simon Manschitz, Jan Peters
3rd Hand (2013-2017; EU FP7 STREP)
Robots have been essential for keeping industrial manufacturing in Europe. Most factories have large numbers of robots in a fixed setup and few programs that produce the exact same product hundreds of thousands times. The only common interaction between the robot and the human worker has become the so-called “emergency stop button”. As a result, re-programming robots for new or personalized products has become a key bottleneck for keeping manufacturing jobs in Europe. The core requirement to date has been the production in large numbers or at a high price.
Robot-based small series production requires a major breakthrough in robotics: the development of a new class of semi-autonomous robots that can decrease this cost substantially. Such robots need to be aware of the human worker, alleviating him from the monotonous repetitive tasks while keeping him in the loop where his intelligence makes a substantial difference.
In the 3rd Hand project, we pursue this breakthrough by developing a semi-autonomous robot assistant that acts as a third hand of a human worker. It will be straightforward to instruct even by an untrained layman worker, allow for efficient knowledge transfer between tasks and enable a effective collaboration between a human worker with a robot third hand. The main contributions of this project will be the scientific principles of semi-autonomous human-robot collaboration, a new semi-autonomous robotic system that is able to: i) learn cooperative tasks from demonstration; ii) learn from instruction; and iii) transfer knowledge between tasks and environments. We will demonstrate its efficiency in the collaborative assembly of an IKEA-like shelf where the robot acts as a semi-autonomous 3rd-Hand.
Contacts: Oliver Kroemer, Guilherme Maeda, Rudolf Lioutikov, Jan Peters
CoDyCo (2013-2017; EU FP7 STREP)
The CoDyCo project is an EU STREP project centered on "Whole-body Compliant Dynamical Contacts in Cognitive Humanoids". The aim of CoDyCo is to advance the current control and cognitive understanding about robust, goaldirected whole-body motion interaction with multiple contacts. CoDyCo will go beyond traditional
approaches: (1) proposing methodologies for performing coordinated interaction tasks with complex systems; (2) combining planning and compliance to deal with predictable and unpredictable events and contacts; (3) validating theoretical advances in real-world interaction scenarios.
First, CoDyCo will advance the state-of-the-art in the way robots coordinate physical interaction and physical mobility. Traditional industrial applications involve robots with limited mobility. Consequently, interaction (e.g. manipulation) was treated separately from whole-body posture (e.g. balancing), assuming the robot firmly connected to the ground. Foreseen applications involve robots with augmented autonomy and physical mobility. Within this novel context, physical interaction influences stability and balance. To allow robots to surpass barriers between interaction and posture control, CoDyCo will be grounded in principles governing whole-body coordination with contact dynamics. Second, CoDyCo will go beyond traditional approaches in dealing with all perceptual and motor aspects of physical interaction, unpredictability included. Recent developments in compliant actuation and touch sensing allow safe and robust physical interaction from unexpected contact including humans. The next advancement for cognitive robots, however, is the ability not only to cope with unpredictable contact, but also to exploit predictable contact in ways that will assist in goal achievement.
Third, the achievement of the project objectives will be validated in real-world scenarios with the iCub humanoid robot engaged in whole-body goal-directed tasks. The evaluations will show the iCub exploiting rigid supportive contacts, learning to compensate for compliant contacts, and utilizing assistive physical interaction
Contacts: Alexandros Paraschos, Roberto Calandra, Elmar Rueckert, Serena Ivaldi, Jan Peters
CompLACS (2011-2015; EU FP7 STREP)
The CompLACS project is also an EU STREP project which can be described as follows: Cognitive architectures capable of operating autonomously in complex environments will require a constant interaction with this environment (e.g. with multiple users, in the case of web agents) and a high degree of modularity (e.g. user profiling module interacting with text generation modules, or recommendation systems, for example).
Understanding the behavior of complex adaptive systems, where multiple parts are both driven by data and co-adapting, is a key question for the design of real world intelligent cognitive systems, that is "Composing learning systems for Artificial Cognitive Systems" or CompLACS. The project aims to develop key enabling machine learning technologies necessary for building artificial cognitive systems as well as developing a principled method of breaking down cognitive system design into well-specified components that can be matched against specified sub-systems together with guarantees on the behaviour of the resulting composition.
Contacts: Gerhard Neumann, Christian Daniel, Marc Deisenroth, Jan Peters
RILCCA (2012-2013; Industry Project)
The Robot Interaction Learning of Cooperative and Competitive Actions (RILCCA) project is funded by a grant of the Daimler-and-Benz Foundation for Postdocs and Juniorprofessors, and can be described as follows: In this project, we will develop new algorithms that allow anthropomorphic robots to learn how to engage in joint
actions with a human partner in order to learn manipulation tasks.
The focus lies on learning models-of-interaction from
observed data, e.g., from a recorded rapport between two persons. Using optical tracking technology the movements of
a pair of persons are first recorded and then processed using machine learning algorithms. The result is a model of how
each person adapted his or her behavior to the the movements of the respective other. Once a model is learned, it can
be used by a robot to engage in a similar interaction with a human counter part. For example, by observing how two
workmen collaborate on a maintenance task using a motion tracking setup, a robot can learn what actions and responses
are needed to assist in a similar maintenance task.
Contact: Heni Ben Amor
GeRT (2010-2013; EU FP7 STREP)
The GeRT project is an EU STREP project. GeRT stands for Generalizing Robot manipulation Tasks. Its' goal is to enable a robot to autonomously generalize its manipulation skills from known objects to previously unmanipulated objects in order to achieve everyday manipulation tasks. To achieve this aim, GeRT employs a set of demonstration programs for the same abstract task with different objects and varying scene arrangements.
These programs are coded by hand and executed on the robotic system. The results from these example programs form the base for generalizing the planning operators and for learning pre and post conditions of operations. We are part of WP3 and WP4 as well as leader for WP5.
Contacts: Oliver Kroemer, Heni Ben Amor, Jan Peters