Kay Pompetzki (fmr Hansel)
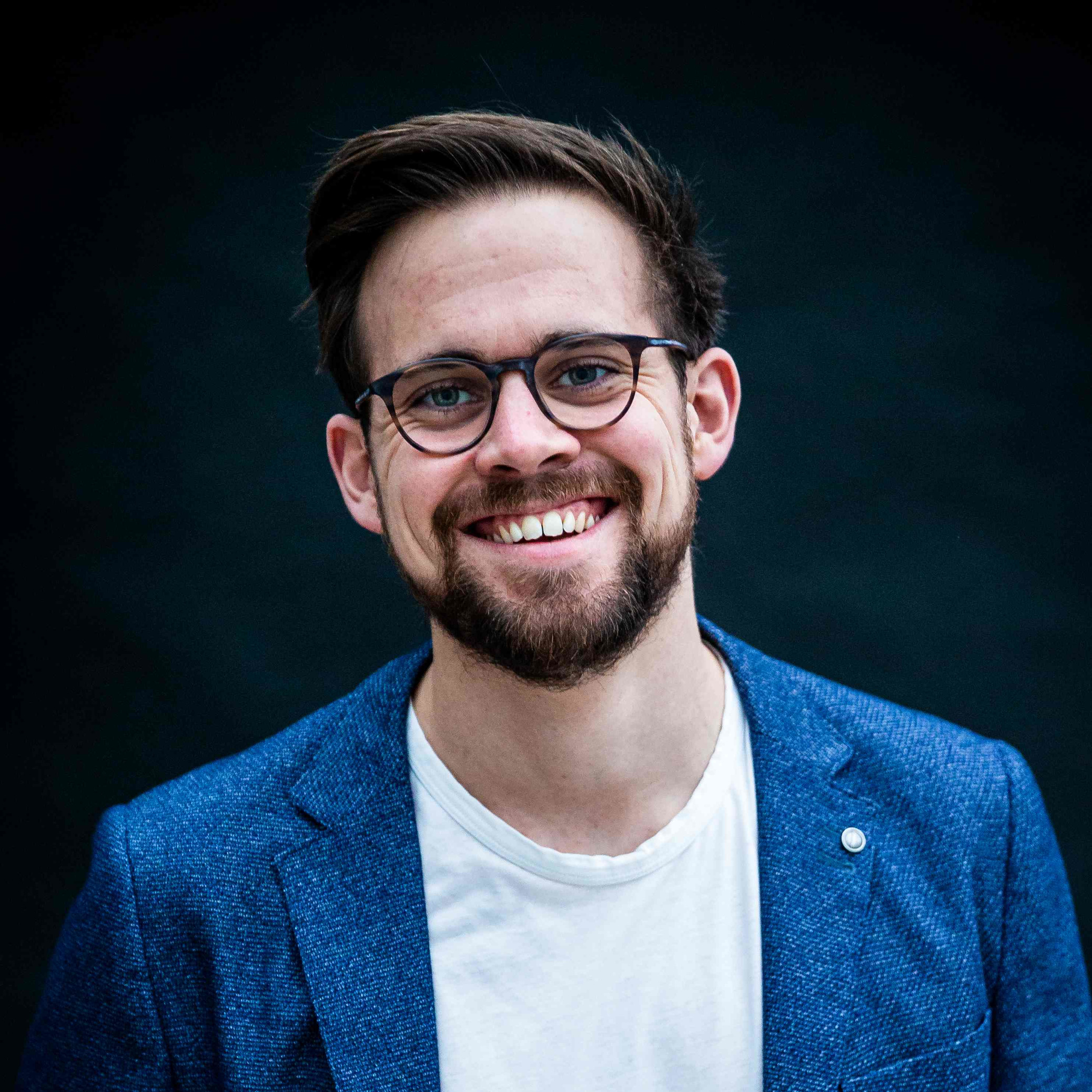
Research Interests
Robot Learning, Machine Learning, Reinforcement Learning, Robotics, Optimal Control, Telerobotics, Human-Robot Interaction
Affiliation
TU Darmstadt, Intelligent Autonomous Systems, Computer Science Department
Contact
kay@robot-learning.de
kay.pompetzki@tu-darmstadt.de
Room D202, Building S2|02, TU Darmstadt, FB-Informatik, FG-IAS, Hochschulstr. 10, 64289 Darmstadt
+49-6151-16-25385
Kay joined the Intelligent Autonomous Systems (IAS) Lab as a Ph.D. student in May 2021. He is interested in experimenting and researching with robots, exploring new concepts in artificial intelligence such as machine learning, reinforcement learning or deep learning, and thereby discovering interdisciplinary connections between various academic disciplines.
Before starting his Ph.D., Kay completed his Bachelor's degree in Applied Mathematics at the RheinMain University of Applied Sciences and his Master's degree in Autonomous Systems at the TU Darmstadt. His thesis, "Probabilistic Dynamic Mode Primitives", was written under the supervision of Svenja Stark and Hany Abdulsamad.
Reviewing
IEEE IROS, IEEE ICRA, CoRL, RSS, IEEE RA-L, and various Robotics & ML workshops.
Lecturer
Lecture | Institution | Years |
Physical AI | Matsuo-Iwasawa Lab @UTokyo | SS 2025 |
Teaching Assistant
Lecture | Years |
Probabilistic Methods for CS | WS 2024/25 |
Robot Learning Integrated Project 1 & 2 | SS 2024 |
Robot Learning | WS 2022/2023, SS 2023 |
Computational Engineering and Robotics | SS 2022, WS 2022/2023 |
During his academic research, Kay had the invaluable opportunity to visit the Intelligent Robotics and Biomechatronics Laboratory led by Prof. Hasegawa as a visiting scholar from May 2023 to July 2023. Part of the Department of Micro-Nano Mechanical Science and Engineering at Nagoya University, the lab is renowned for its fundamental and applied studies on mechatronics technologies for advanced human assistance systems. In November 2024, Kay joined the German delegation for the Junior Expert Exchange (JEX) program, engaging in high-level discussions on robotics and AI policy with Japanese ministries and academic institutions. Building on these experiences, he served as lecturer in the Physical AI lecture series, sharing insights on machine learning in robotics at the Matsuo-Iwasawa Lab of the University of Tokyo in spring 2025.
Recent Publications
Robot Control and Motion Planning
-
- Le, A. T.; Hansel, K.; Carvalho, J.; Watson, J.; Urain, J.; Biess, A.; Chalvatzaki, G.; Peters, J. (2025). Global Tensor Motion Planning, IEEE Robotics and Automation Letters (RA-L), 10, 7, pp.7302-7309.
- Le, A. T.; Hansel, K.; Carvalho, J.; Urain, J.; Biess, A.; Chalvatzaki, G.; Peters, J. (2024). Global Tensor Motion Planning, CoRL 2024 Workshop on Differentiable Optimization Everywhere.
-
- Hansel, K.; Urain, J.; Peters, J.; Chalvatzaki, G. (2023). Hierarchical Policy Blending as Inference for Reactive Robot Control, 2023 IEEE International Conference on Robotics and Automation (ICRA), IEEE.
-
- Le, A. T.; Hansel, K.; Peters, J.; Chalvatzaki, G. (2023). Hierarchical Policy Blending As Optimal Transport, 5th Annual Learning for Dynamics & Control Conference (L4DC), PMLR.
Goal Inference
-
- Pompetzki, K.; Le, A. T.; Gruner, T.; Watson, J.; Chalvatzaki, G.; Peters, J. (2025). Geometrically-Aware Goal Inference: Leveraging Motion Planning as Inference, German Robotics Conference (GRC).
- Pompetzki, K.; Le, A. T.; Gruner, T.; Watson, J.; Chalvatzaki, G.; Peters, J. (2025). Geometrically-Aware Goal Inference: Leveraging Motion Planning as Inference, Multi-disciplinary Conference on Reinforcement Learning and Decision Making (RLDM).
Telerobotics
-
--- Best Paper Award Finalist ---- Zhu, Y.; Nazirjonov, S.; Jiang, B.; Colan, J.; Aoyama, T.; Hasegawa, Y.; Belousov, B.; Hansel, K.; Peters, J. (2023). Visual Tactile Sensor Based Force Estimation for Position-Force Teleoperation, IEEE International Conference on Cyborg and Bionic Systems (CBS), pp.49-52.
-
--- Best Paper Award ---- Chen, Q.; Zhu, Y.; Hansel, Kay.; Aoyama, T.; Hasegawa, Y. (2023). Human Preferences and Robot Constraints Aware Shared Control for Smooth Follower Motion Execution, IEEE International Symposium on Micro-NanoMechatronics and Human Science (MHS), IEEE.
-
- Becker, N.; Gattung, E.; Hansel, K.; Schneider, T.; Zhu, Y.; Hasegawa, Y.; Peters, J. (2024). Integrating Visuo-tactile Sensing with Haptic Feedback for Teleoperated Robot Manipulation, IEEE ICRA 2024 Workshop on Robot Embodiment through Visuo-Tactile Perception.
-
- Becker, N.; Sovailo, K.; Zhu, C.; Gattung, E.; Hansel, K.; Schneider, T.; Zhu, Y.; Hasegawa, Y.; Peters, J. (2024). Integrating and Evaluating Visuo-tactile Sensing with Haptic Feedback for Teleoperated Robot Manipulation, 40th Anniversary of the IEEE International Conference on Robotics and Automation (ICRA@40).
-
- Moyen, S. B.; Krohn, R.; Lueth, S.; Pompetzki, K.; Chalvatzaki, G. (2025). Intuitive User Interfaces for Mobile Manipulation, German Robotics Conference (GRC).
Robot Learning
-
- Hansel, K.; Moos, J.; Abdulsamad, H.; Stark, S.; Clever, D.; Peters, J. (2022). Robust Reinforcement Learning: A Review of Foundations and Recent Advances, Machine Learning and Knowledge Extraction (MAKE), 4, 1, pp.276--315, MDPI.
-
- Hansel, K.; Moos, J.; Derstroff, C. (2021). Benchmarking the Natural Gradient in Policy Gradient Methods and Evolution Strategies, Reinforcement Learning Algorithms: Analysis and Applications, pp.69--84, Springer.
-
- Belousov, B.; Abdulsamad H.; Klink, P.; Parisi, S.; Peters, J. (2021). Reinforcement Learning Algorithms: Analysis and Applications, Studies in Computational Intelligence, Springer International Publishing.
-
- Hansel, K. (2021). Probabilistic Dynamic Mode Primitives, Master Thesis.
Others
-
- Watson, J.; Song, C.; Weeger, O.; Gruner, T.; Le, A.T.; Hansel, K.; Headway, A.; Arenz, O.; Trojak, W.; Cranmer, M.; D’Eramo, C.; Bülow, F.; Goyal, T.; Peters, J.; Hoffman, M.W.; (submitted). Machine Learning with Physics Knowledge for Prediction: A Survey, Transactions on Machine Learning Research (TMLR).
-
- Le, A.T.; Pompetzki, K.; Peters, J.; Biess, A. (2025). Kinematics Correspondence As Inexact Graph Matching, German Robotics Conference (GRC).
- Le, A.T.; Pompetzki, K.; Peters, J.; Biess, A. (2025). Kinematics Correspondence As Inexact Graph Matching, Multi-disciplinary Conference on Reinforcement Learning and Decision Making (RLDM).
Supervised Theses and Projects
Year | Thesis/Project | Student(s) | Topic | Together with |
2021 | RL:IP | Gao, Z., Shao, F. | Towards Intelligent Shared-Control with TIAGo++ | Chalvatzaki, G. |
2021 | RL:IP | Langer, M. | Learn to Play Tangram with Graph-based Reinforcement Learning 1 | Funk, N. |
2022 | RL:IP | Zimmermann, M., Langer, M., Marino, D. | Learn to Play Tangram with Graph-based Reinforcement Learning 2 | Funk, N. |
2022 | RL:IP | Zimmermann, M., Zöller, M., Aristakesyan, A. | Learn to Play Tangram with Graph-based Reinforcement Learning 3 | Funk, N. |
2023 | MSc Thesis | Gao, Z. | Hierarchical Contextualization of Movement Primitives | Chalvatzaki, G., Peters, J., Antink, C. H. |
2023 | MSc Thesis | Gece, A. | Leveraging Structured-Graph Correspondence in Imitation Learning | Le, A. T., Chalvatzaki, G. |
2023 | MSc Thesis | Langer, M. | Energy-based Models for 6D Pose Estimation | Funk, N., Peters, J. |
2024 | RL:IP | Gattung, E., Becker, N. | Integrating Visuo-tactile Sensing with Haptic Feedback for Teleoperated Robot Manipulation 1 | Schneider, T. |
2024 | S:HR | Beyer, H. | Controlling Humanoids: A Comprehensive Analysis of Teleoperation Frameworks | |
2024 | MSc Thesis | Cosserat, E. | Refining 6D pose estimation with tactile sensors | Schneider, T., Duret, G., Peters, J., Chen, L. |
2024 | RL:IP | Sun, Y., Huang, Y. | Control Barrier Functions for Assisted Teleoperation 1 | Gueler, B. |
2024 | RL:IP | Becker, N., Sovailo, K., Zhu, C. | Integrating Visuo-tactile Sensing with Haptic Feedback for Teleoperated Robot Manipulation 2 | Schneider, T., Funk, N. |
2025 | RL:IP | Sun, Y., Huang, Y. | Control Barrier Functions for Assisted Teleoperation 2 | Gueler, B. |
2025 | MSc Thesis | Zach, S. | Reactive Motion Generation through Probabilistic Dynamic Graphs | Le, A. T., Peters, J. |
2025 | MSc Thesis | Moyen, S. | User Study of Intuitive Teleoperation Systems for Mobile Manipulation | Krohn, R., Chalvatzaki, G. |
2025 | RL:IP | Rodriguez, J. F. | Blending Deep Generative Models using Stochastic Optimization 1 | Funk, N. |
Ongoing | RL:IP | Zoltan, M., Schönfeld, P., Qian, H. | Motion Planning on Constraint Manifolds | Farias, C. M. |
Ongoing | RL:IP | Neykov, D., Lang, D., Stebich, D., Knaußt, P. | Hierarchical Semantic Segmentation of Human Demonstration Data | Farias, C. M. |
Ongoing | RL:IP | Rodriguez, J. F. | Blending Deep Generative Models using Stochastic Optimization 2 | Funk, N. |
Ongoing | MSc Thesis | Zoller, L. | Generative models for motion planning leveraging control barrier functions | Carvalho, J. |
Ongoing | MSc Thesis | Sun, Y. | A Shared Autonomy Framework for Assisted Teleoperation Using Control Barrier Functions | Gueler, B., Hirt., S., Findeisen, R. |