João Carvalho
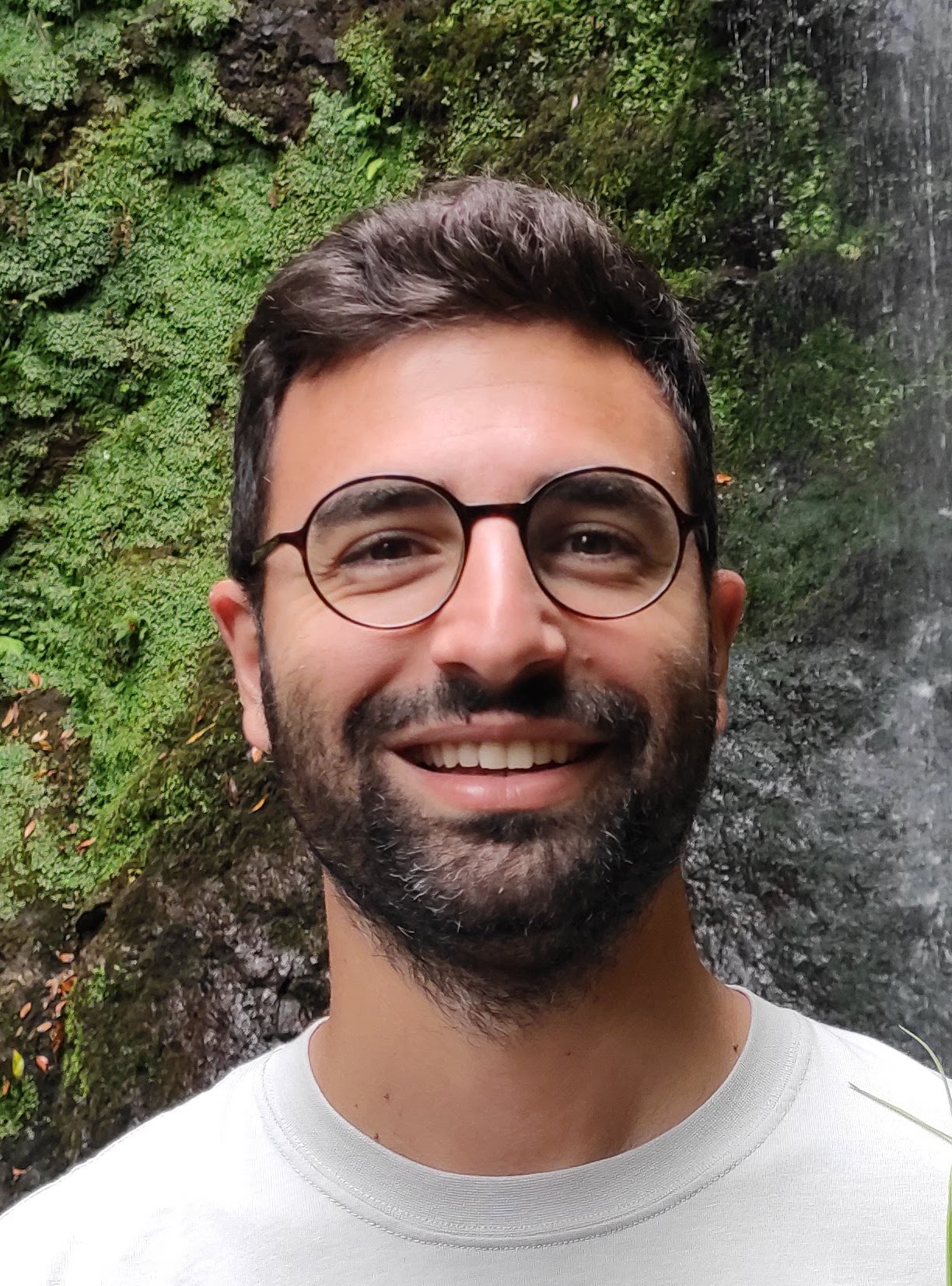
Research Interests
- Robotic Manipulation
- Learning Motion Planning
- Reinforcement Learning for Robotics
Affiliation
TU Darmstadt, Intelligent Autonomous Systems, Computer Science Department
Contact
joao@robot-learning.de (preferred)
joao.correia_carvalho@tu-darmstadt.de
Room E325, Building S2|02, TU Darmstadt, FB-Informatik, FG-IAS, Hochschulstr. 10, 64289 Darmstadt
+49-6151-16-25372
João is a Postdoctoral Researcher at the Intelligent Autonomous Systems group.
Previously, he obtained his Ph.D. degree from TU Darmstadt, a M.Sc. degree in Computer Science from the Albert-Ludwigs-Universität Freiburg, and completed a Master's degree in Electrical and Computer Engineering from the Instituto Superior Técnico of the University of Lisbon.
His master's thesis was written under the supervision of Samuele Tosatto and explored an approach to obtain an off-policy gradient with higher sample efficiency.
He has worked on several research projects, such as KoBo34 and IKIDA.
His research interests are developing machine learning and reinforcement learning algorithms for robot manipulation.
These include methods that leverage generative models for motion planning and grasping, reinforcement learning methods to solve contact-rich tasks like insertions, or improving policy gradient methods with variance reduction techniques.
Publications
- Funk, N.; Urain, J.; Carvalho, J.; Prasad, V.; Chalvatzaki, G.; Peters, J. (submitted). ActionFlow: Equivariant, Accurate, and Efficient Policies with Spatially Symmetric Flow Matching.
- Carvalho, J.; Le, A.T.; Jahr, P. ; Sun, Q. ; Urain, J.; Koert, D.; Peters, J. (submitted). Grasp Diffusion Network: Learning Grasp Generators from Partial Point Clouds with Diffusion Models in SO(3)xR3, Submitted to the IEEE Robotics and Automation Letters (R-AL).
- Palenicek, D.; Lutter, M.; Carvalho, J.; Dennert, D.; Ahmad, F.; Peters, J. (submitted). Diminishing Return of Value Expansion Methods, Submitted to the IEEE Transaction on Pattern Analysis and Machine Intelligence (PAMI).
- Carvalho, J.; Le, A.T.; Kicki, P. ; Koert, D.; Peters, J. (submitted). Motion Planning Diffusion: Learning and Adapting Robot Motion Planning with Diffusion Models, Submitted to the IEEE Transactions on Robotics (T-Ro).
- Le, A. T.; Nguyen, K.; Vu, M.N.; Carvalho, J.; Peters, J. (submitted). Model Tensor Planning, Transactions on Machine Learning Research (TMLR).
- Le, A. T.; Hansel, K.; Carvalho, J.; Watson, J.; Urain, J.; Biess, A.; Chalvatzaki, G.; Peters, J. (2025). Global Tensor Motion Planning, IEEE Robotics and Automation Letters (RA-L), 10, 7, pp.7302-7309.
- Carvalho, J.; Le, A.; Jahr, P. ; Sun, Q. ; Urain, J.; Koert, D.; Peters, J. (2025). Grasp Diffusion Network: Learning Grasp Generators from Partial Point Clouds with Diffusion Models in SO(3)×R3, German Robotics Conference (GRC).
- Carvalho, J. (2025). Enhancing Robot Manipulation Skills through Learning, PhD Thesis.
- Le, A. T.; Nguyen, K.; Vu, M.N.; Carvalho, J.; Peters, J. (2025). Model Tensor Planning, ICRA @ RoboARCH: Robotics Acceleration with Computing Hardware and Systems.
- Le, A. T.; Hansel, K.; Carvalho, J.; Urain, J.; Biess, A.; Chalvatzaki, G.; Peters, J. (2024). Global Tensor Motion Planning, CoRL 2024 Workshop on Differentiable Optimization Everywhere.
- Funk, N.; Urain, J.; Carvalho, J.; Prasad, V.; Chalvatzaki, G.; Peters, J. (2024). ACTIONFLOW: Equivariant, Accurate, and Efficient Manipulation Policies with Flow Matching, CoRL 2024 Workshop on Mastering Robot Manipulation in a World of Abundant Data.
- Funk, N.; Urain, J.; Carvalho, J.; Prasad, V.; Chalvatzaki, G.; Peters, J. (2024). ActionFlow: Efficient, Accurate, and Fast Policies with Spatially Symmetric Flow Matching, R:SS workshop: Structural Priors as Inductive Biases for Learning Robot Dynamics.
- Palenicek, D.; Lutter, M.; Carvalho, J.; Peters, J. (2023). Diminishing Return of Value Expansion Methods in Model-Based Reinforcement Learning, International Conference on Learning Representations (ICLR).
- Carvalho, J.; Le, A. T.; Baierl, M.; Koert, D.; Peters, J. (2023). Motion Planning Diffusion: Learning and Planning of Robot Motions with Diffusion Models, IEEE/RSJ International Conference on Intelligent Robots and Systems (IROS).
- Tosatto, S.; Carvalho, J.; Peters, J. (2022). Batch Reinforcement Learning with a Nonparametric Off-Policy Policy Gradient, IEEE Transactions on Pattern Analysis and Machine Intelligence (PAMI), 44, 10, pp.5996--6010.
- Carvalho, J.; Peters, J. (2022). An Analysis of Measure-Valued Derivatives for Policy Gradients, Multi-disciplinary Conference on Reinforcement Learning and Decision Making (RLDM).
- Carvalho, J.; Koert, D.; Daniv, M.; Peters, J. (2022). Adapting Object-Centric Probabilistic Movement Primitives with Residual Reinforcement Learning, 2022 IEEE-RAS 21st International Conference on Humanoid Robots (Humanoids).
- Vorndamme, J.; Carvalho, J.; Laha, R.; Koert, D.; Figueredo, L.; Peters, J.; Haddadin, S. (2022). Integrated Bi-Manual Motion Generation and Control shaped for Probabilistic Movement Primitives, 2022 IEEE-RAS 21st International Conference on Humanoid Robots (Humanoids).
- Carvalho, J.; Baierl, M; Urain, J; Peters, J. (2022). Conditioned Score-Based Models for Learning Collision-Free Trajectory Generation, NeurIPS 2022 Workshop on Score-Based Methods.
- Carvalho, J., Tateo, D., Muratore, F., Peters, J. (2021). An Empirical Analysis of Measure-Valued Derivatives for Policy Gradients, International Joint Conference on Neural Networks (IJCNN).
- Tosatto, S.; Carvalho, J.; Abdulsamad, H.; Peters, J. (2020). A Nonparametric Off-Policy Policy Gradient, Proceedings of the 23rd International Conference on Artificial Intelligence and Statistics (AISTATS).
- Carvalho, J.A.C. (2019). Nonparametric Off-Policy Policy Gradient, Master Thesis.
Supervised Theses and Projects
Teaching Assistant
Lecture | Years |
Computational Engineering and Robotics | SS 2020, SS 2021 |
Robot Learning | WS 2020 |
Robot Learning Integrated Project | SS 2022 |