An Thai Le
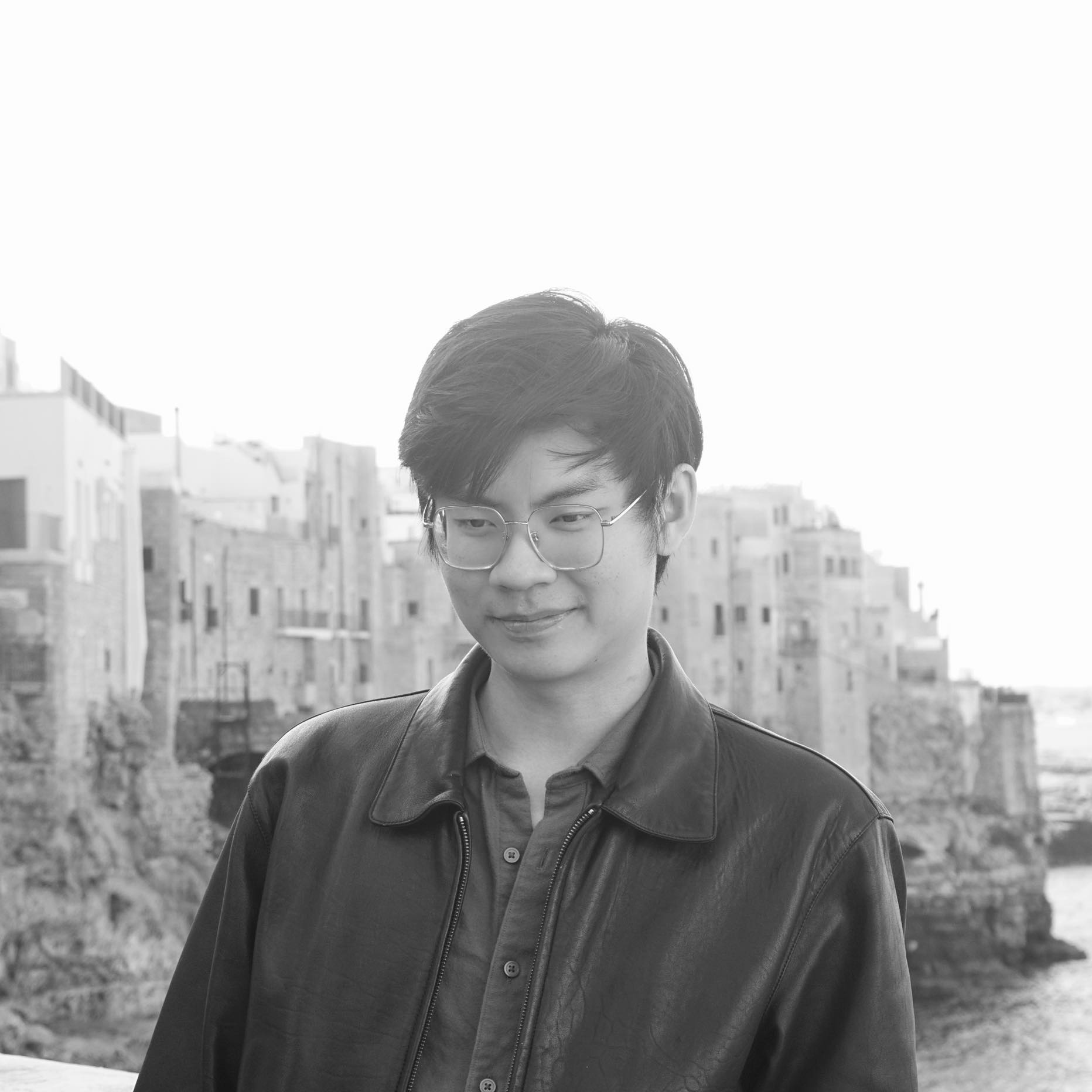
Research Interests
- Scaling robot planning
- Learning to plan
- Optimal Transport theory and gradient flows
Affiliation
TU Darmstadt, Intelligent Autonomous Systems, Computer Science Department
Contact
an.le@tu-darmstadt.de
Room D202, Building S2|02, TU Darmstadt, FB-Informatik, FG-IAS, Hochschulstr. 10, 64289 Darmstadt
+49-6151-16-20073
An Thai Le joined the Intelligent Autonomous System lab on November 1st, 2021, as a Ph.D. student. Currently, he is working to scale robotics learning and planning methods with batch optimization. In particular, he aims to scale planning methods to long-horizon, high-dimensional state-space, number of plans, and number of agents. Batch planning methods are crucial for robotics since they could discover many homotopy classes in the multi-objective problems, thereby exhibiting robustness to bad local minima. Furthermore, these batch methods can serve as a strong oracle for collecting datasets or striving to discover a global optimal solution for robotics skill execution.
Reviewing
IROS, ICRA, R:SS, RLC, CoRL, NeurIPS, ICML, AAAI, IEEE RA-L, IEEE T-RO, and various Robotics & ML workshops.
Teaching Assistant
- Reinforcement Learning (SS 2022)
- Statistical Machine Learning (SS 2023, WS 2023/2024)
Before his Ph.D., An Thai Le worked on forceful imitation learning during the internship at Bosch AI. He also worked on task and motion planning for human-robot collaboration settings. During his master's research, he was fortunate to be advised by Dr. Meng Guo and Dr. Jim Mainprice.
Recent Publications
Optimal Transport In Robotics
-
- Le, A. T.; Chalvatzaki, G.; Biess, A.; Peters, J. (2023). Accelerating Motion Planning via Optimal Transport, Advances in Neural Information Processing Systems (NIPS / NeurIPS).
- Le, A. T.; Chalvatzaki, G.; Biess, A.; Peters, J. (2023). Accelerating Motion Planning via Optimal Transport, IROS 2023 Workshop on Differentiable Probabilistic Robotics: Emerging Perspectives on Robot Learning, [Oral].
- Le, A. T.; Chalvatzaki, G.; Biess, A.; Peters, J. (2023). Accelerating Motion Planning via Optimal Transport, NeurIPS 2023 Workshop Optimal Transport and Machine Learning, [Oral].
-
- Le, A. T.; Hansel, K.; Peters, J.; Chalvatzaki, G. (2023). Hierarchical Policy Blending As Optimal Transport, 5th Annual Learning for Dynamics & Control Conference (L4DC), PMLR.
Optimal Transport In ML
-
- Nguyen, D.M.H.; Lukashina, N.; Nguyen, N.; Le, A.T.; Nguyen, T.T.; Ho, N.; Peters, J.; Sonntag, D.; Zaverkin, V.; Niepert, M. (2024). Structure-Aware E(3)-Invariant Molecular Conformer Aggregation Networks, Proceedings of the International Conference on Machine Learning (ICML).
Generative Modeling For Imitation Learning And Motion Planning
-
- Carvalho, J.; Le, A. T.; Baierl, M.; Koert, D.; Peters, J. (2023). Motion Planning Diffusion: Learning and Planning of Robot Motions with Diffusion Models, IEEE/RSJ International Conference on Intelligent Robots and Systems (IROS).
-
- Urain, J.; Le, A. T.; Lambert, A.; Chalvatzaki, G.; Boots, B.; Peters, J. (2022). Learning Implicit Priors for Motion Optimization, IEEE/RSJ International Conference on Intelligent Robots and Systems (IROS).
- Le, A. T.; Urain, J.; Lambert, A.; Chalvatzaki, G.; Boots, B.; Peters, J. (2022). Learning Implicit Priors for Motion Optimization, RSS 2022 Workshop on Implicit Representations for Robotic Manipulation.
-
- Le, A. T.; Guo M.; Duijkeren, N.; Rozo, L.; Krug, R.; Kupcsik, A.G.; Buerger, M. (2021). Learning forceful manipulation skills from multi-modal human demonstrations, IEEE/RSJ International Conference on Intelligent Robots and Systems (IROS).
Supervised Theses and Projects
Year | Type | Together with | Student(s) | Topic | Document |
---|---|---|---|---|---|
2022 | Project | Junning Huang | Lu Liu, Yuheng Ouyang, Jiahui Shi | Benchmark Neural Lyapunov Control Algorithms on Pendulum | |
2022 | Project | Junning Huang | Chao Jin, Liyuan Xiang, Peng Yan | Hybrid Motion-Force Optimization | |
2022 | Master Thesis | Ali Younes, Georgia Chalvatzaki | Daljeet Nandha | Leveraging Large Language Models For Autonomous Task Planning | |
2023 | Master Thesis | Joao Carvalho, Julen Urain De Jesus | Mark Baierl | Score-based Planning Networks for Robot Motion Planning | |
2023 | Master Thesis | Kay Hansel, Jan Peters, Georgia Chalvatzaki | Alper Gece | Leveraging Structured-Graph Correspondence in Imitation Learning | |
2023 | Master Thesis | Kay Hansel, Jan Peters | Marius Zoeller | Enhancing Smoothness in Policy Blending with Gaussian Processes | [Ongoing] |
2023 | Master Thesis | Ali Younes, Georgia Chalvatzaki | Caio Freitas | Graph Correspondence Diffusion For Imitation Learning | |
2023 | Master Thesis | Georgia Chalvatzaki | Denis Andric | Learning Symplectic Manifold Of Dynamical Systems | [Ongoing] |
2023 | Master Thesis | Georgia Chalvatzaki | Nico Nonnengiesser | Graph Neural Network For Robotics Control | [Ongoing] |
2024 | Master Thesis | Joao Carvalho | Qiao Sun | Geometry-Aware Diffusion Models for Robotics | [Ongoing] |
2024 | Master Thesis | Kay Hansel | Sebastian Zach | T.B.D. | [Ongoing] |