I am now a postdoctoral research associate at the Oxford Robotics Institute. For my details see my personal page.
Joe Watson
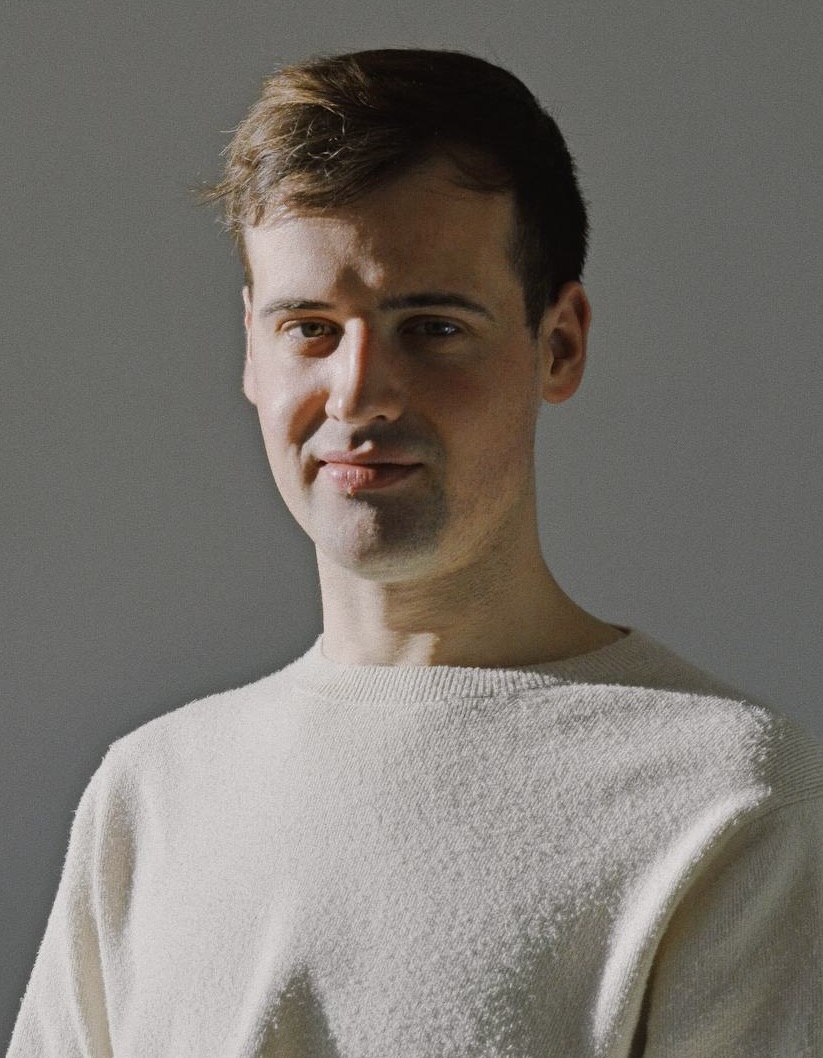
Research Interests
- Statistical methods for learning control
- Bayesian methods and approximate inference
- Differentiable physics and robotic priors
Affiliation
TU Darmstadt, Intelligent Autonomous Systems, Computer Science Department
Contact
joseph.watson(at)dfki(dot)de
joe.watson@robot-learning.de
Room 2.1.16, Building S4|14, DFKI, SAIROL, Mornewegstraße 30, 64293 Darmstadt
Joe joined the Intelligent Autonomous Systems Group at TU Darmstadt as a Ph.D. researcher in December 2018. He studied Information & Computer Engineering at the University of Cambridge, where he received his BA and MEng. His Master’s thesis “Vision-Based Learning for Robotic Grasping”, which investigated the use of Convolutional Neural Networks for real-world grasp prediction, was undertaken at the Bio-Inspired Robotics Lab (BIRL) under the supervision of Dr Fumiya Iida. For two years, Joe worked at CMR Surgical (previously Cambridge Medical Robotics), a medical device startup. He worked extensively on the control and signal processing stack of the manipulators of Versius, a bespoke robotic platform for laparoscopic surgery, which to date has performed over 10,000 surgical procedures globally.
Supervision
- Msc, Zhiyuan Hu
- Msc, Benedikt Hanher
- Msc, Fabio D’Aquino Hilt (with Joao Carvalho)
- Msc, Jihao Andreas Lin (with Pascal Klink)
- Msc, Thomas Gossard (with Michael Lutter)
- Msc, Yannick Eich (with Hany Abdulsamad)
- Msc, Johannes Silberbauer (with Michael Lutter)
- Msc, Len Williamson
- Bsc, Darya Nikitina
- Bsc, Amin Ali
- Bsc, Fabian Damken
Teaching
Robot Learning teaching assistant (2020 - 2022)
Reviewing
CoRL, NeurIPS, ICML, ICLR, AISTATS, IROS, IEEE RAL, Neurocomputing
Joe was selected as an R:SS Pioneer for robotics research in 2022 and was an intern at Google DeepMind Robotics in 2022-23.
Joe is interested in the duality between entropy-regularized optimization and (pseudo-) Bayesian inference for robot learning, as a means of designing principled methods for complex learning tasks. The application of these techniques range from trajectory optimization, imitation learning and model-free reinforcement learning. The Bayesian methodology also motivate the careful design of models and priors, which has inspired research involving Gaussian processes, differentiable physics simulators and function-space inference techniques.
Key References
Control as Inference
-
- Watson, J.; Peters, J. (2022). Inferring Smooth Control: Monte Carlo Posterior Policy Iteration with Gaussian Processes, Conference on Robot Learning (CoRL).
-
- Watson, J.; Abdulsamad, H.; Peters, J. (2019). Stochastic Optimal Control as Approximate Input Inference, Conference on Robot Learning (CoRL).
-
- Watson, J.; Peters, J. (2021). Advancing Trajectory Optimization with Approximate Inference: Exploration, Covariance Control and Adaptive Risk, American Control Conference (ACC).
-
- Watson, J.; Peters, J.; (2022). Stationary Posterior Policy Iteration with Variational Inference, The Multi-disciplinary Conference on Reinforcement Learning and Decision Making (RLDM).
-
- Watson, J.; Huang, S. H.; Heess, N. (2023). Coherent Soft Imitation Learning, Advances in Neural Information Processing Systems (NeurIPS).
Bayesian Machine Learning
-
- Watson, J.; Lin, J. A.; Klink, P.; Peters, J. (2021). Neural Linear Models with Functional Gaussian Process Priors, 3rd Symposium on Advances in Approximate Bayesian Inference (AABI).
-
- Watson, J.; Lin J. A.; Klink, P.; Pajarinen, J.; Peters, J. (2021). Latent Derivative Bayesian Last Layer Networks, Proceedings of the International Conference on Artificial Intelligence and Statistics (AISTATS).
Inductive Biases and Differentiable Physics
-
- Lutter, M.; Silberbauer, J.; Watson, J.; Peters, J. (2021). Differentiable Physics Models for Real-world Offline Model-based Reinforcement Learning, Proceedings of the IEEE International Conference on Robotics and Automation (ICRA).
-
- Watson, J.; Hanher, B.; Peters, J. (2022). Differentiable Simulators as Gaussian Processes, R:SS Workshop: Differentiable Simulation for Robotics.
-
- Watson, J.; Hahner, B.; Belousov, B.; Peters, J. (2024). Tractable Bayesian Dynamics Priors from Differentiable Physics for Learning and Control, 40th Anniversary of the IEEE International Conference on Robotics and Automation (ICRA@40).
-
- Funk, N.; Schaff, C.; Madan, R.; Yoneda, T.; Urain, J.; Watson, J.; Gordon, E.; Widmaier, F; Bauer, S.; Srinivasa, S.; Bhattacharjee, T.; Walter, M.; Peters, J. (2022). Benchmarking Structured Policies and Policy Optimization for Real-World Dexterous Object Manipulation, IEEE Robotics and Automation Letters (R-AL).