I have graduated and moved to Boston Dynamics in Cambridge, MA, USA. You can find more up-to-date information on my personal homepage.
Michael Lutter
Research Interests
Machine Learning, Robotics,
Reinforcement Learning,
Deep Learning
More Information
CV Publications Google Scholar
Contact Information
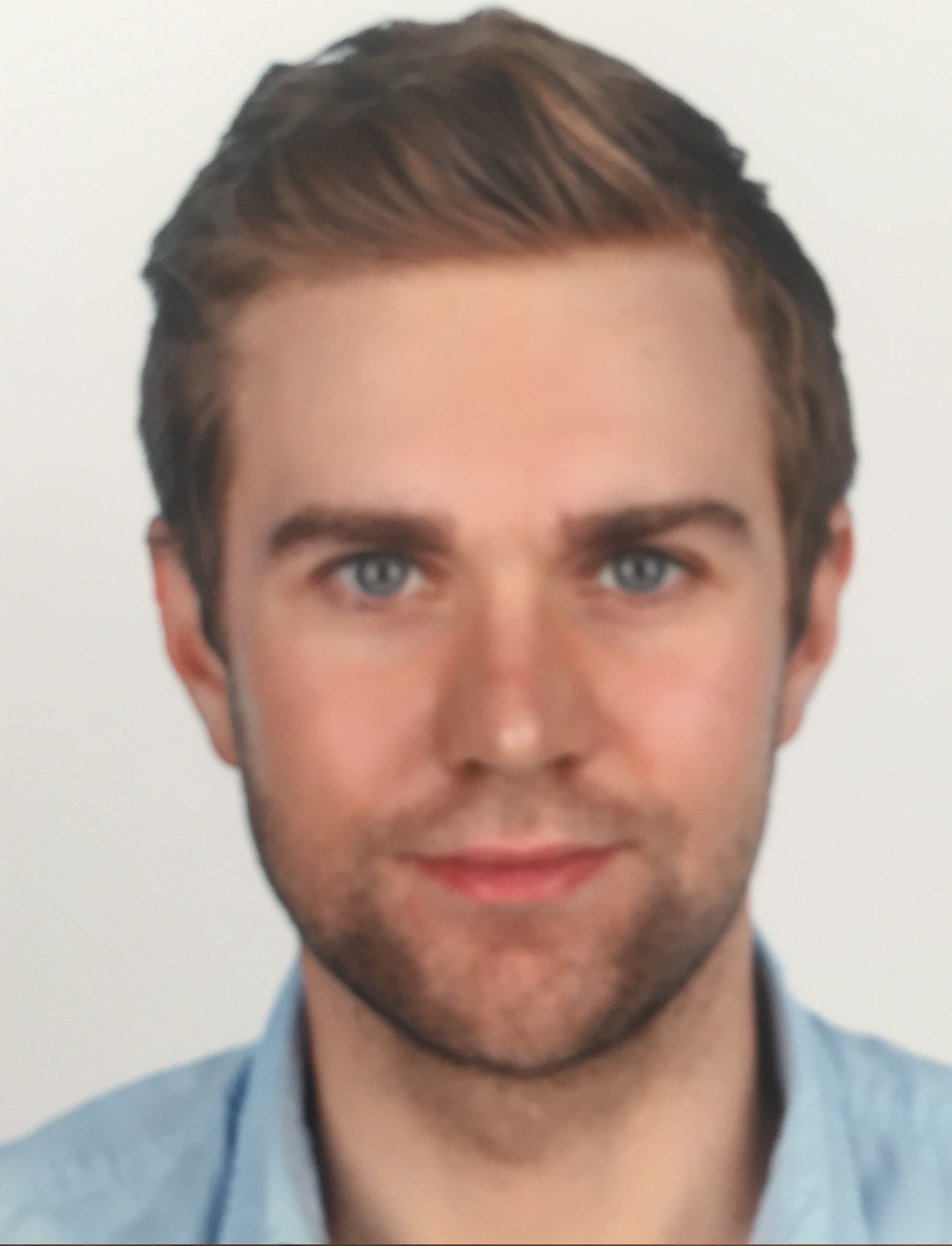
Research Topic
During his Ph.D. Michael works on a joint project with ABB Corporate Research on bringing robot learning to industrial applications. The current assembly automation using robotics is limited by the high cost and long duration of robot programming and the inflexible programs. Hence, robotic automation is only applicable to standardised and high volume manufacturing. However, the trend for highly personalised products requires assembly automation for smaller volumes. With other words, future robots must perform thousand tasks several times rather than a single task thousand times. Robot learning promises the generation of intelligent robots capable of being programmed by workers rather than engineers and capable of transferring knowledge between tasks. Therefore, reducing cost for programming and time-to-deployment, which is a key enabler for robotic automation for low-volume and personalised manufacturing. Within this context, Michael first evaluates the current state of the art of robot learning by demonstrating the current capabilities on a small assembly task. Afterwards, he will focus his research on transferring knowledge between similar domain tasks.
Research Interests
Machine Learning:
Deep Learning, Non-Convex Optimisation, Reinforcement Learning, Structured Learning, Inductive Biases, Safe Exploration, Neuro-Inspired Learning, Neuromorphic Hardware
Robotics:
High-Speed Robotics, Industrial Manipulators, Humanoids, Dexterous Manipulation, Learning for Control, Optimal Control, Robust Control, Motion Representation
Key References
-
- Lutter, M.; Ritter, C.; Peters, J. (2019). Deep Lagrangian Networks: Using Physics as Model Prior for Deep Learning, International Conference on Learning Representations (ICLR).
-
- Lutter, M.; Mannor, S.; Peters, J.; Fox, D.; Garg, A. (2021). Robust Value Iteration for Continuous Control Tasks, Robotics: Science and Systems (RSS).
-
- Lutter, M.; Mannor, S.; Peters, J.; Fox, D.; Garg, A. (2021). Value Iteration in Continuous Actions, States and Time, International Conference on Machine Learning (ICML).
-
- Lutter, M.; Peters, J. (2019). Deep Lagrangian Networks for end-to-end learning of energy-based control for under-actuated systems, International Conference on Intelligent Robots and Systems (IROS).