I am now an Assistant Professor at the University of Innsbruck. Please see my new homepage at https://samueletosatto.com ...
Samuele Tosatto
Research Interests
Robotics, Machine Learning, Reinforcement Learning, Partial Observability
More Information
CV All Publications Google Scholar
Contact Information
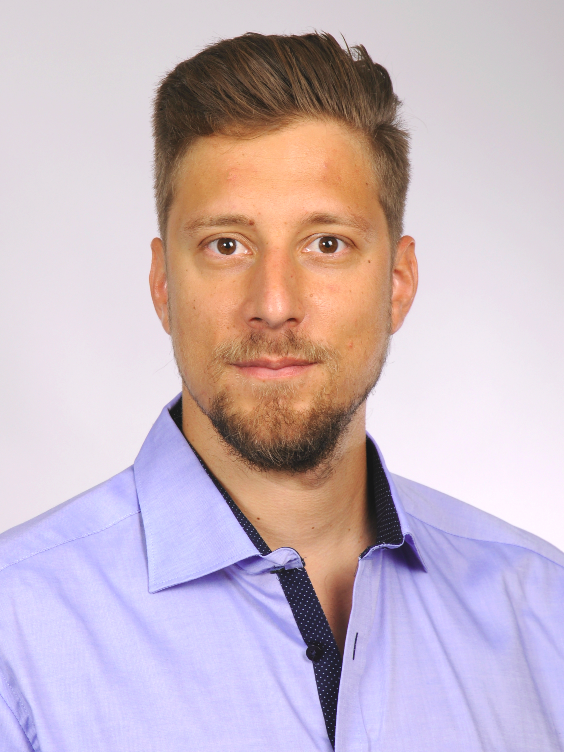
Samuele received his bachelor degree as well as his master degree in software engineering from Polytechnic University of Milan. During his studies he focused on machine learning and more in particular in reinforcement learning.
His thesis, entitled “Boosted Fitted Q-Iteration", was written under the supervision of Prof. Marcello Restelli PhD Matteo Pirotta and Ing Carlo D'Eramo.
Research Topic
In the recent years we saw an important technology advancement in the field of artificial intelligent, mainly devoted to improvements in the field of machine learning, which has become nowadays a very active field both in the perspective of research and industry. This technological improvement had an impact in the way we program robots: instead of an ad-hoc programming to accomplish a given task, it is now preferred to make the robot learn how to solve such task. This former approach has a lot of benefits: it relives the programmer to implement difficult solutions, it could find a very optimal way to solve the task, just to mention a few. Reinforcement leanring is a machine learning technique which aims to solve sequential decision problems by learning. Despite the recent advances in the field, especially in solving games and simulated environment, often the direct application of reinforcement learning to real-robotic task is unsatisfactory and leads to poor results.
The main reason is that online methods are very sample inefficient, meaning they require an unreasonable amount of interaction with the environment, which is often intractable for real systems. Moreover, to enable safety, we want to use a different policy than the optimization one.
Off-policy techniques tries to overcome the issues presented, but the current state of the art often fails to provide a true off-policy estimation. Samuele worked out an analytical off-policy estimation of the gradient which results to be more accurate than the current state off-the art.
Samuele also focused his research on the problem of exploration under sparse reward, so to obtain a sample-efficient way to explore with a low-informative reward signal.
He is focusing now to bring his findings closer to real application and robotics.
Research Interests
Reinforcement Learning, Off-Policy Gradient Estimation, Exploration, Risk-Awareness
Key References
-
- Tosatto, S.; Carvalho, J.; Peters, J. (2022). Batch Reinforcement Learning with a Nonparametric Off-Policy Policy Gradient, IEEE Transactions on Pattern Analysis and Machine Intelligence (PAMI), 44, 10, pp.5996--6010.
- Tosatto, S.; Carvalho, J.; Abdulsamad, H.; Peters, J. (2020). A Nonparametric Off-Policy Policy Gradient, Proceedings of the 23rd International Conference on Artificial Intelligence and Statistics (AISTATS).
-
- Tosatto, S.; D'Eramo, C.; Pajarinen, J.; Restelli, M.; Peters, J. (2019). Exploration Driven By an Optimistic Bellman Equation, Proceedings of the International Joint Conference on Neural Networks (IJCNN).
- Tosatto, S.; D'Eramo, C.; Pajarinen, J.; Restelli, M.; Peters, J. (2018). Technical Report: Exploration Driven by an Optimistic Bellman Equation.
-
- Tosatto, S.; Pirotta, M.; D'Eramo, C; Restelli, M. (2017). Boosted Fitted Q-Iteration, Proceedings of the International Conference of Machine Learning (ICML).