I have graduated and joined the Honda Research Institute (HRI) as a Senior Scientist.
Daniel Tanneberg
Research Interests
(Biologically-inspired) Machine Learning, (Memory-augmented) Neural Networks, Deep Learning, (Stochastic) Neural Networks, Lifelong-Learning
More Information
Curriculum Vitae Publications ResearchGate Network Google Scholar ORCID Twitter
Contact Information
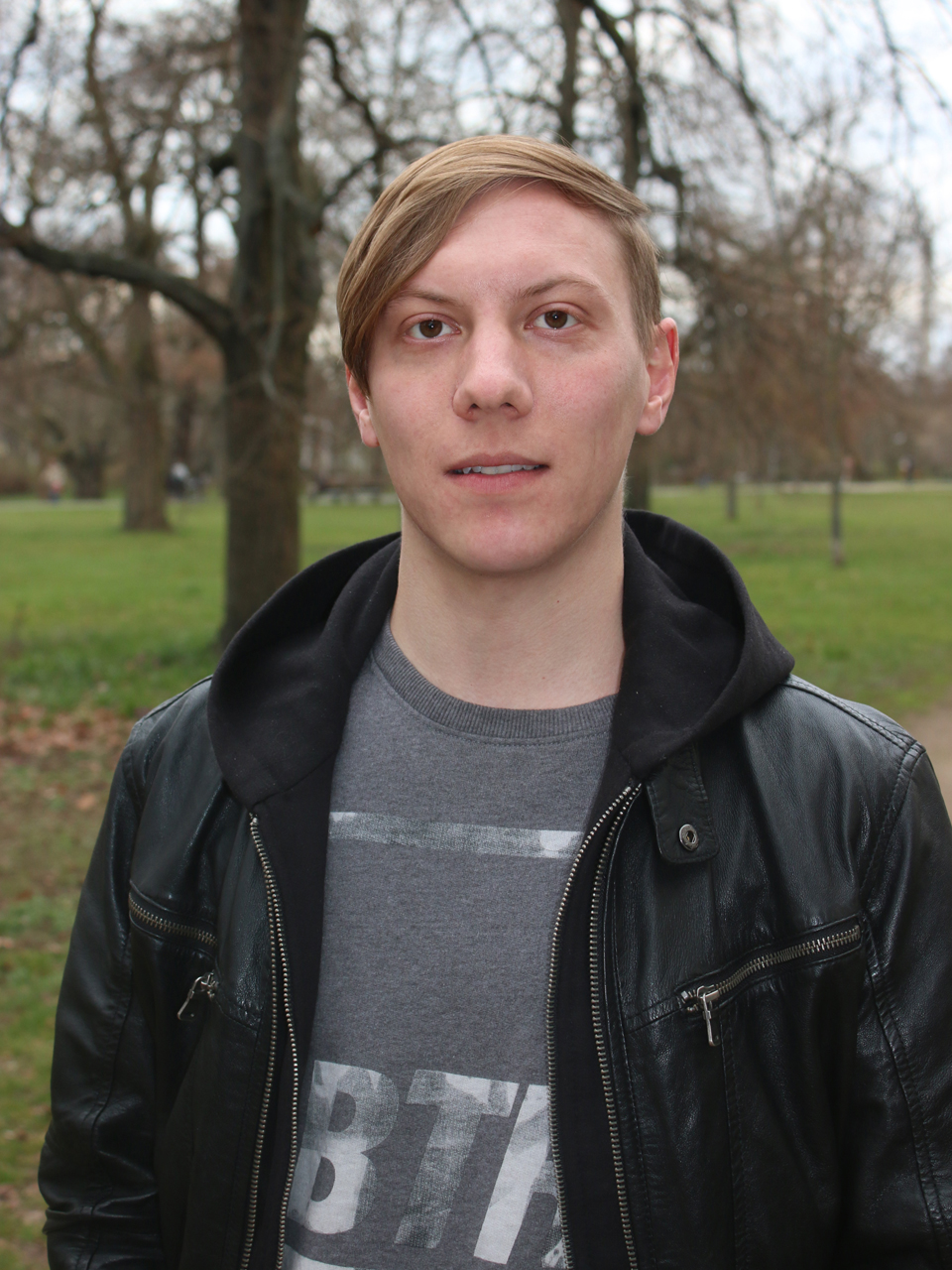
Generally he is interested in the connection between machine learning, robotics and neuroscience. Daniel was also a member of the Athena-Minerva Cybathlon-Team of TU Darmstadt (IAS Group) and the Max Planck Institute for Intelligent Systems, which build a Brain-Computer-Interface system and participated in the BCI-Race at the Cybathlon 2016. The team and the related research aims to contribute to the BCI research that wants to give paralyzed people a way to communicate. Beside that, he was also involved on the TACMAN Project which used tactile-sensing to improve the manipulation abilities and dexterity of robotic hands.
Before starting his Ph.D., Daniel completed both, his Bachelor Degree in Computer Science and his Master Degree in Computer Science (with honors) at the Technische Universitaet Darmstadt. During his master, he focused on machine learning and studied Biological Psychology as a minor to get into learning and information processing in humans. His master thesis entitled “Spiking Neural Networks Solve Robot Planning Problems" was written under the supervision of Elmar Rueckert and Jan Peters and was decorated with the Hanns-Voith-Stiftungspreis Award 2017.
Key References
-
- Tanneberg, D.; Ploeger, K.; Rueckert, E.; Peters, J. (2021). SKID RAW: Skill Discovery from Raw Trajectories, IEEE Robotics and Automation Letters (RA-L).
-
- Tanneberg, D.; Rueckert, E.; Peters, J. (2020). Evolutionary Training and Abstraction Yields Algorithmic Generalization of Neural Computers, Nature Machine Intelligence, 2, 12, pp.753-763.
-
- Tanneberg, D.; Peters, J.; Rueckert, E. (2019). Intrinsic Motivation and Mental Replay enable Efficient Online Adaptation in Stochastic Recurrent Networks, Neural Networks, 109, pp.67-80.
For all publications please see Publications or Google Scholar.