After graduation, I moved to McGill University to work with Joelle Pineau, Greg Dudek, and Dave Meger. Currently, I am a tenure track assistant prof at University of Amsterdam. Check out my new homepage ...
Herke van Hoof
Research Interests
Reinforcement learning, robotics, active learning and exploration.
More Information
Curriculum Vitae Publications Google Citations DBLP
Contact Information
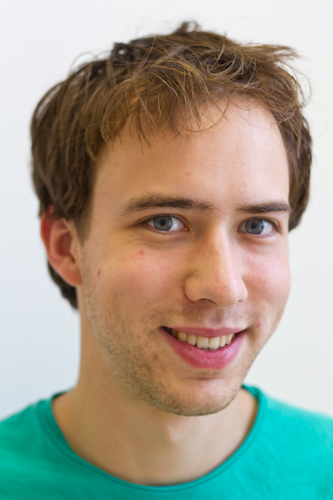
One example of such a task is exploring the objects present in a novel environment. Segmenting objects using passive sensing is inherently limited. By interacting with the environment, the robot can improve its understanding of the different objects that are present. However, interaction is costly. By expressing the uncertainty in the robot's understanding of the world, it becomes possible to select actions based on the information they are expected to yield about the environment, and thus speed up the learning progress.
In another project, we consider reinforcement learning with high-dimensional inputs. Current approaches have usually tried to learn features in a separate step. However, such features cannot be informed by what is relevant for the task at hand. We have taken a complementary approach, where we have developed a non-parametric reinforcement learning method that only depends on the similarity between data-points, independent of the embedding dimensionality.
Before joining IAS, Herke got his bachelor and master degrees in Artificial Intelligence at the University of Groningen in the Netherlands. Within this degree, Herke focused on autonomous systems and perception, writing his bachelor thesis on "Using different methods to attract a robot's attention" under supervision of Gert Kootstra. During his master's, Herke got involved with RoboCup competitions, first in the 3D soccer simulation student team 'The little green BATS'. He proceeded to do an internship at INSERM in Lyon, France, where he participated in a RoboCup@Home team and worked on his master thesis on "Interaction between face detection and learning tracking systems" which explored ways to make different perception subsystems train each other to improve robot performance continuously. This work was done under the supervision of Tijn van der Zant, Peter Ford Dominey, and Marco Wiering.
Research Interests
Bayesian Machine Learning, Reinforcement Learning, Non-parametric methods, Active Learning, Active Perception, Autonomous Exploration
Key References
-
- van Hoof, H.; Neumann, G.; Peters, J. (2017). Non-parametric Policy Search with Limited Information Loss, Journal of Machine Learning Research (JMLR), 18, 73, pp.1-46.
-
- van Hoof, H.; Tanneberg, D.; Peters, J. (2017). Generalized Exploration in Policy Search, Machine Learning (MLJ), 106, 9-10, pp.1705-1724.
-
- van Hoof, H.; Peters, J.; Neumann, G. (2015). Learning of Non-Parametric Control Policies with High-Dimensional State Features, Proceedings of the International Conference on Artificial Intelligence and Statistics (AISTATS).
-
- van Hoof, H.; Kroemer, O; Peters, J. (2014). Probabilistic Segmentation and Targeted Exploration of Objects in Cluttered Environments, IEEE Transactions on Robotics (TRo), 30, 5, pp.1198-1209.
-
- van Hoof, H.; Kroemer, O.;Ben Amor, H.; Peters, J. (2012). Maximally Informative Interaction Learning for Scene Exploration, Proceedings of the International Conference on Robot Systems (IROS).
A full list of my publications can be found on this page.
CV
Herke's CV can be found here.
Research projects
On the topic of information-theoretic action selection in interactive exploration of new scenes, we made a short video:
⚠ (:html5video filename=hoof2014_interactive poster=Team.HerkeVanHoof/hoof2014_interactive.jpg width=640 height=360:)
A detailed description of this research topic is given in our TRo paper:
- van Hoof, H.; Kroemer, O; Peters, J. (2014). Probabilistic Segmentation and Targeted Exploration of Objects in Cluttered Environments, IEEE Transactions on Robotics (TRo), 30, 5, pp.1198-1209.