Oleg Arenz
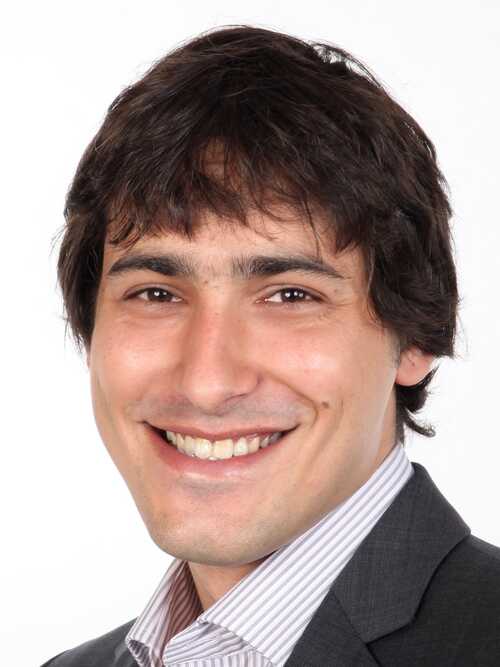
Research Interests
Machine Learning, Robotics, Inverse Reinforcement Learning, Imitation Learning, Grasping and Manipulation, Reinforcement Learning, Variational Inference
Affiliation
TU Darmstadt, Intelligent Autonomous Systems, Computer Science Department
Contact
oleg.arenz@tu-darmstadt.de
Room E226, Building S2|02, TU Darmstadt, FB-Informatik, FG-IAS, Hochschulstr. 10, 64289 Darmstadt
+49-6151-16-20074
Oleg Arenz is a staff scientist at the Intelligent Autonomous Systems group, where he will establish and lead the Laboratory for Humanoid Robotics, a cross-departmental research facility that bundles research in the field of humanoid robotics at TU Darmstadt. He already worked at the IAS as graduate researcher during his PhD (2015-2020), although he was advised by Gerhard Neumann, who went to the University of Lincoln in 2016 and is currently full professor at the Karlsruher Institute of Technology.
During my PhD, I investigated several different learning problems for robotics, namely reinforcement learning, inverse reinforcement learning and variational inference, and showed that they can all be framed as an information projection, which is a particular type of distribution-matching problem. By treating the aforementioned learning problems as different instances of an information projection, we can solve them based on similar insights. For example, we derived an upper bound on the I-Projection objective and used it in combination with an expectation-maximization procedure for variational inference, density estimation as well as non-adversarial imitation learning.
Software
GMMVI: A high-performance and well-documented framework for optimizing Gaussian mixture models for variational inference using natural gradient descent. The framework is quite modular, allowing for different techniques, for example for estimating the natural gradients or selecting the samples for each update. In total 432 different combinations of design choices are supported.
Many kinds of learning problems have been proven to be very useful for robotics and will remain integral parts of increasingly intelligent robots. These learning problems include reinforcement learning, imitation learning, inverse reinforcement learning and various types of supervised, semi-supervised and unsupervised problems. However, in order to develop intelligent robots capable of assisting humans in a variety of different tasks in a changing environment---for example domestic robots---, we need to go beyond these general but limited problem formulations. I think it is time to move to the next layer of abstraction, to devise new mathematically well-specified objectives that encompass and build on the aforementioned learning problems. I argue that it is important to think about the different modules that make up the overall system, how they interact with each other and what goal they optimize together because we need to build on their synergies in order to scale to more complex problem settings.
However, not just we, also the robot itself should devise new abstraction layers. Reasoning about a task on different levels of abstractions is beneficial for many challenging problems in reinforcement learning and inverse reinforcement learning. High-level MDPs have the potential for targeted exploration that enables us to learn better low-level controllers, and their reward functions and policies are more generalizable and less prone to changes in the environment. On the other hand, we need low-level policies to control the robot---especially for highly dynamic and reactive movements---and for representing skills that can be used within different higher-level tasks. Hence, I argue that hierarchical learning will be a key ingredient of future, holistic approaches to robot learning.
Key References
-
- Arenz, O.; Dahlinger, P.; Ye, Z.; Volpp, M.; Neumann, G. (2023). A Unified Perspective on Natural Gradient Variational Inference with Gaussian Mixture Models, Transactions on Machine Learning Research (TMLR).
-
- Arenz, O.; Zhong, M.; Neumann G. (2020). Trust-Region Variational Inference with Gaussian Mixture Models, Journal of Machine Learning Research (JMLR).
-
- Arenz, O.; Neumann, G. (2020). Non-Adversarial Imitation Learning and its Connections to Adversarial Methods, arXiv.
-
- Arenz, O.; Abdulsamad, H.; Neumann, G. (2016). Optimal Control and Inverse Optimal Control by Distribution Matching, Proceedings of the International Conference on Intelligent Robots and Systems (IROS), IEEE.
-
- Arenz, O. (2021). Sample-Efficient I-Projections for Robot Learning, Ph.D. Thesis.
For a complete list of my publications see this complete list.