I have graduated and moved to Bosch Center for Artificial Intelligence in Renningen near Stuttgart.
Christian Daniel
Research Interests
Motor Control & Learning, Robotics, Machine Learning, Biomimetic Systems.
More Information
Curriculum Vitae Publications Google Citations DBLP
Contact Information
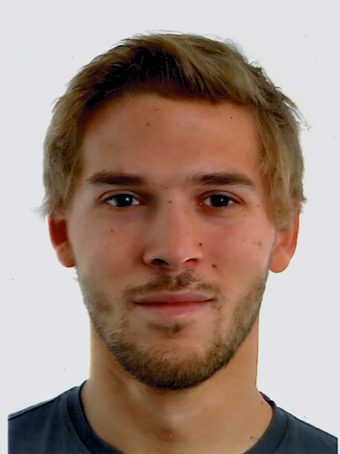
Christian Daniel joined the institute for Intelligent Autonomous Systems in August 2011 as a Masters student. He was born and raised in Frankfurt/ Main in Germany where he also went to school and completed his civil service duties.
Before writing his master thesis at the IAS, he received his bachelor of science from TU Darmstadt in the field of computational fluid dynamics. He then proceeded to leave Darmstadt for one year, during which he studied at EPFL in Lausanne, Switzerland. There, he focussed away from the field of computational fluid dynamics in favor of the field of robotics in general and artificial intelligence in particular. After finishing the official school year at EPFL he got the opportunity to stay on as a research assistant at EPFL's LASA lab, working with Aude Billard and Dan Grollman. Back in Germany, he went on to specialize in the field of AI and became a Master's student at the IAS lab. Recently, his thesis at IAS has won the Datenlotsenpreis 2013 for the best Master's thesis in Computer Science. After his Master's thesis, Christian became a Ph.D. student at the IAS lab.
Christian's research interests specialize on the field of skill and transfer learning, how robot learning compares to human learning and what makes humans 'intelligent'. These are very basic questions on the way to true artificial intelligence, that still have to be covered. While skill learning has been around for some time, there still remains a lot to be done. Transfer learning, on the other hand is an area of research that still is very much unexplored.
Key References
-
(conference version received the IROS CoTeSys Cognitive Robotics Best Paper Award while being both the IROS 2012 Best Paper Award Finalist and the IROS 2012 Best Student Paper Award Finalist.)- Daniel, C.; Neumann, G.; Kroemer, O.; Peters, J. (2016). Hierarchical Relative Entropy Policy Search, Journal of Machine Learning Research (JMLR), 17, pp.1-50.
-
- Daniel, C.; Kroemer, O.; Viering, M.; Metz, J.; Peters, J. (2015). Active Reward Learning with a Novel Acquisition Function, Autonomous Robots (AURO), 39, pp.389-405.
-
- Daniel, C.; Taylor, J.; Nowozin, S. (2016). Learning Step Size Controllers for Robust Neural Network Training, National Conference of the American Association for Artificial Intelligence (AAAI).
-
(received the Best Student Paper Award of ECMLPKDD 2016 sponsored by the Machine Learning journal)- Daniel, C.; van Hoof, H.; Peters, J.; Neumann, G. (2016). Probabilistic Inference for Determining Options in Reinforcement Learning, Machine Learning (MLJ), 104, 2-3, pp.337-357.
For all publications please see my Publication Page