Lisa Kempf
Research Interests
Human-Robot Interaction, Machine Learning, Interactive Robot Skill Learning
Affiliations
Contact
lisa.kempf@robot-learning.de
Room E225, Building S2|02, TU Darmstadt, FB-Informatik, FG-IAS, Hochschulstr. 10, 64289 Darmstadt
+49-6151-16-20073
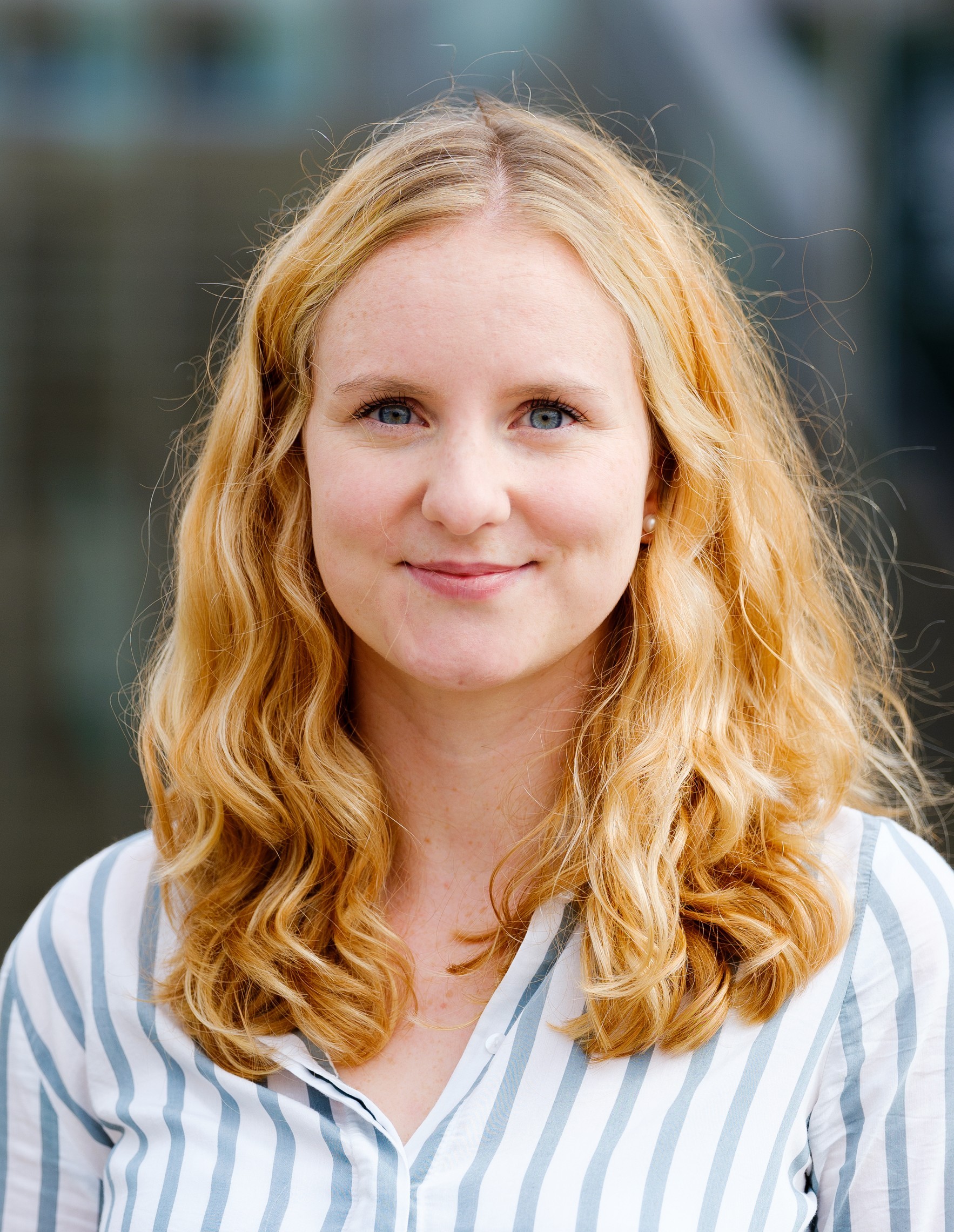
Lisa Kempf joined the Intelligent Autonomous Systems Group at TU Darmstadt as a Ph.D. student in January 2021 and is part of the interdisciplinary research group IKIDA, which started in October 2020. She is interested in the development of future interactive AI systems and how to exploit partially erroneous human input and feedback.
Before joining the Autonomous Systems Labs, Lisa Kempf completed her Bachelor's and Master's degree in Psychology in IT at the Technische Universitaet Darmstadt. Her thesis "Learning to segment human sequential behavior to detect the intention for interaction." was written under supervision of Susanne Trick and Constantin Rothkopf.
Currently, Lisa's research focuses on the development of interactive AI systems taking into account human uncertainty and erroneous or incomplete human input. In particular, her research topics include AI-based detection of human uncertainty, e.g. from behavioral features such as gestures, facial expressions, speech, and response times and how this uncertainty can be processed in interactive reinforcement learning algorithms. In addition, she is recently exploring robot task learning from few and potentially incomplete human demonstrations.
Publications
-
- Scherf, L.; Gasche, L. A.; Chemangui, E.; Koert, D. (2024). Are You Sure? - Multi-Modal Human Decision Uncertainty Detection in Human-Robot Interaction, 2024 ACM/IEEE International Conference on Human-Robot Interaction (HRI ’24).
-
- Scherf, L.; Schmidt, A.; Pal, S.; Koert, D. (2023). Interactively learning behavior trees from imperfect human demonstrations, Frontiers in Robotics and AI, 10.
-
- Gassen, M.; Metzler, F.; Prescher, E.; Prasad, V.; Scherf, L. Kaiser, F.; Koert, D. (2023). I^3: Interactive Iterative Improvement for Few-Shot Action Segmentation, 32th IEEE International Conference on Robot & Human Interactive Communication (RO-MAN).
-
- Scherf, L.; Turan, C.; Koert, D. (2022). Learning from Unreliable Human Action Advice in Interactive Reinforcement Learning, 2022 IEEE-RAS 21st International Conference on Humanoid Robots (Humanoids).
-
- Scherf, L. (2021). Learning to segment human sequential behavior to detect the intention for interaction, Master Thesis.
-
- Scherf, L.; Kirchbuchner, F.; Wilmsdorff, J. V.; Fu, B.; Braun, A.; Kuijper, A. (2018). Step by Step: Early Detection of Diseases Using an Intelligent Floor, European Conference on Ambient Intelligence, pp.131-146, Springer Cham.
Student Supervision
- MSc Thesis, "Learning Action Conditions from Human Demonstrations", Fröhlich, K. (2023)
- MSc Thesis, "Detecting Human Uncertainty from Multimodal Behavioral Data in a Task with Decision Uncertainty", Gasche, L. (2023)
- BSc Thesis, "Quantifying Policy Uncertainty for Interactive Reinforcement Learning with Unreliable Human Action Advice", Maurer, C. (2023)
- BSc Thesis, "Detecting Human Uncertainty from Multimodal Behavioral Data in a Task with Perceptual Ambiguity", Chemangui, E. (2023)
- BSc Thesis, "Comparing and Personalizing Human Following Behaviors for Mobile Ground Robots", Woortman, N. (2022)
- Integrated Project, "Interactive Semi-Supervised Action Segmentation", Gassen, M., Prescher, E., Metzler, F. (2023)
- Integrated Project, "Multimodal Attention for Natural Human-Robot Interaction", Tatalovic, A., Jehn, M., Vadgama, D. (2022)
- Integrated Project, "Interactively Learning Behavior Trees from Videos", Schmidt, A., Dannenberg, N. (2022)
- Integrated Project, "Learning Behavior Trees from Videos", Heeg, J., Schmidt, A., Worring, A. (2021)