We at IAS were deeply shaken when we learned about Linh's tragic accident on June 28, 2019 and we still cannot believe that his bright future was cut short. We will always remember him as a highly intelligent, nice, enthusiastic and helpful undergrad just as much as an outstanding Ph.D. student with a bright future. We will miss this extraordinary smart and pleasant fellow student, colleague and friend.
Hong Linh Thai
Research Interests
Machine Learning, Reinforcement Learning, Bayesian Deep Learning, POMDPs, Information Theoretic Policy Search, Exploration
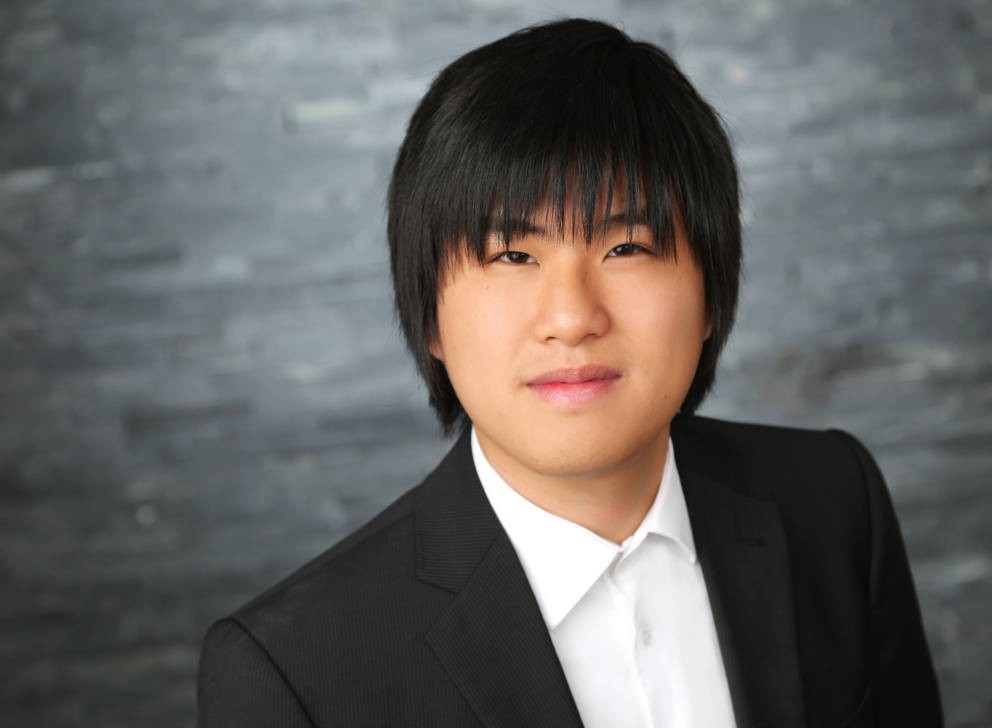
Before his PhD, Linh completed both his Bachelor and Master Degree in Computer Science at the Technische Universität Darmstadt. His Master's Thesis was focused on deep reinforcement learning for partially observable environments and supervised by Gerhard Neumann, Joni Pajarinen and Jan Peters.
Research Interest
In the last few years reinforcement learning, especially deep reinforcement learning, has made a big impact by achieving human level gameplay in the Atari 2600 console games just using images as input and by learning robot control policies end-to-end from images to motor signals - task which were previously intractable for reinforcement learning due to the high dimensionality. Most of these results were obtained on fully observable environments, which is a common assumption in reinforcement learning. However, in the real world this assumption is often violated, since there can be many cases where the full world state cannot be perceived by a robot, e.g. due to occlusions or having only local observations. In these cases, often the agent needs to first gather information about the true environmental state until he his certain enough before he can start exploiting. Therefore, it is necessary to create new deep reinforcement learning methods, which can learn this information gathering process in partially observable environments. Furthermore, one drawback of deep reinforcement learning is sample inefficiency: tons of interactions with the environment are needed to learn a good policy, which can be highly costly in the real world for applications as autonomous driving or robotics. To reduce the number of needed samples model-based reinforcement learning is a promising route for future research. On the back of this we want to be able to use model bias obtain safe exploration and robust policies.