Learning BCIs for Robotics-based Rehabilitation
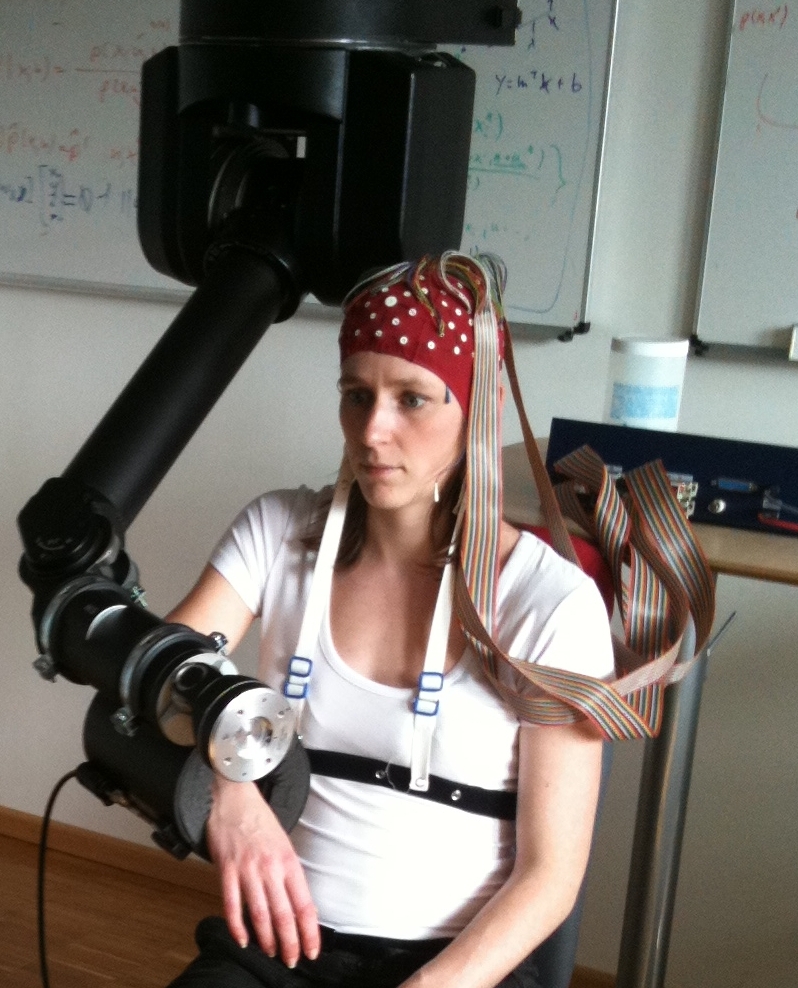
Stroke is a leading cause of long-term motor disability among adults. Current rehabilitative interventions often do not help patients with severe motor impairment. Significant functional recovery after a year is rare despite novel interventional approaches for application in the chronic stage such as bilateral arm training or constraint-induced movement therapy. Brain-Computer Interfaces (BCIs) in combination with robot-assisted physical therapy may play an important role for neurorehabilitation of patients suffering severe hemiparetic syndromes caused by stroke, as proposed in [3]. Reinforcement of the patient own arm movements using a robot arm has been effective for moderately impaired stroke patients, but this is not a feasible option for severely affected stroke patients who are not capable of arm movement at all. In this scenario, we assume that brain signal based reinforcement of the patient’s intent to move his/her own arm using a robot arm may have a positive effect in recovering his mobility, as it is likely to result in increased cortical plasticity due to Hebbian-type learning rules.
In such a scenario, we aim for instantaneous haptic feedback, on-line decoding with high accuracy and specificity when decoding brain regions. Working with a stroke patient, we have shown in [2] how ECoG enables on-line decoding with high accuracy and high specificity. Further work that build on the idea of specificity is under progress.
A key aspect is how to reestablish the disrupted sensorimotor feedback loop. However, it has been an open question to date how artificially closing the sensorimotor feedback loop influences the decoding performance of a BCI. We answer this issue in [1] by studying six healthy subjects, and present empirical evidence that instantaneous haptic feedback helps on-line decoding of arm movement intention.
Contact persons: Manuel Gomez Rodriguez, Moritz Grosse-Wentrup, Jan Peters
References
-
- Gomez Rodriguez, M.; Peters, J.; Hill, J.; Schoelkopf, B.; Gharabaghi, A.; Grosse-Wentrup, M. (2010). Closing the Sensorimotor Loop: Haptic Feedback Facilitates Decoding of Arm Movement Imagery, Proceedings of the IEEE International Conference on Systems, Man, and Cybernetics (Workshop on Brain-Machine Interfaces).
-
- Gomez Rodriguez, M.; Grosse Wentrup, M.; Peters, J.; Naros, G.; Hill, J.; Gharabaghi, A.; Schoelkopf, B. (2010). Epidural ECoG Online Decoding of Arm Movement Intention in Hemiparesis, 1st ICPR Workshop on Brain Decoding: Pattern Recognition Challenges in Neuroimaging.
-
- Gomez Rodriguez, M.; Peters, J.; Hill, J.; Gharabaghi, A.; Schoelkopf, B.; Grosse-Wentrup, M. (2010). BCI and robotics framework for stroke rehabilitation, Proceedings of the 4th International BCI Meeting, May 31 - June 4, 2010. Asilomar, CA, USA.