Locomotion 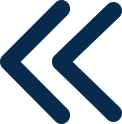
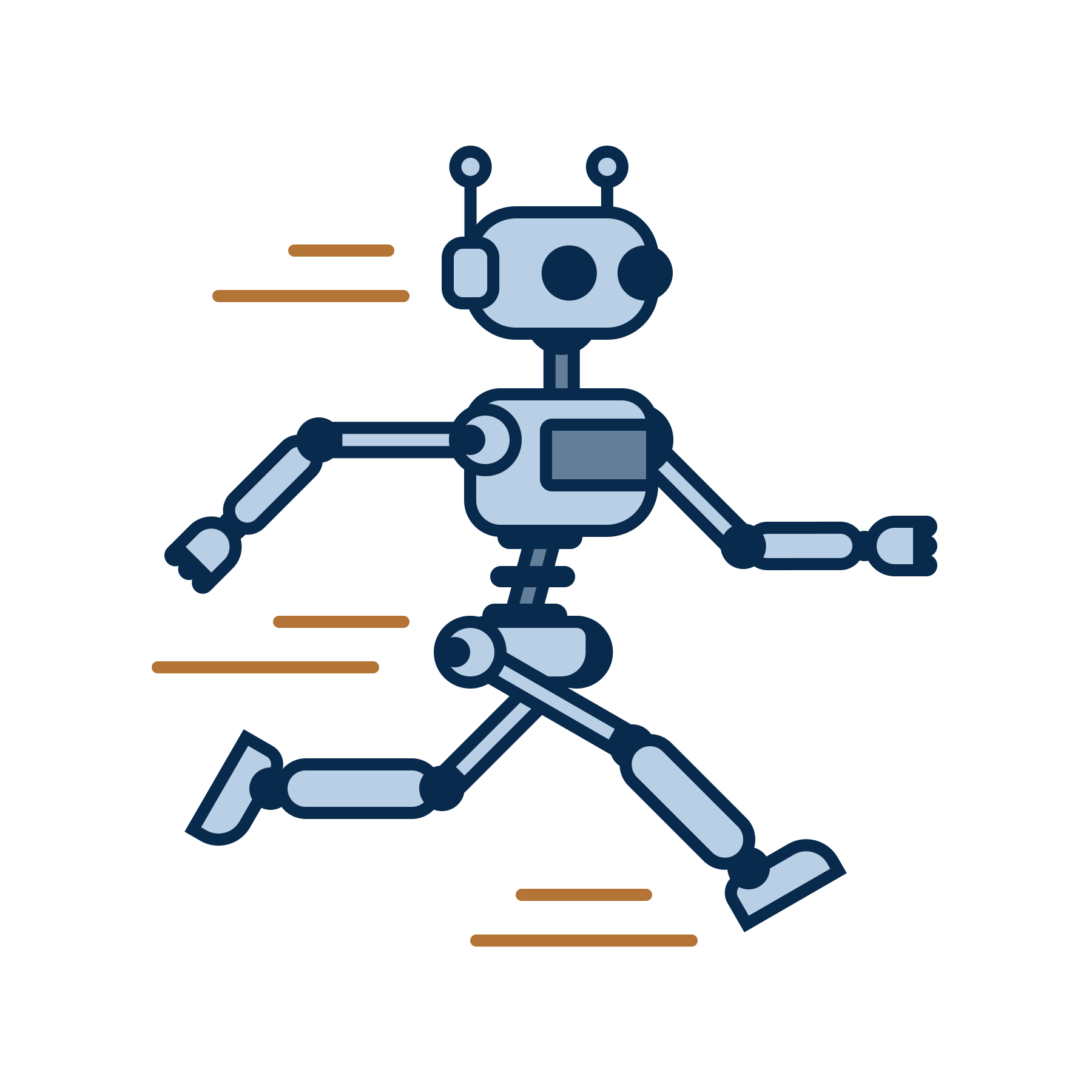
Locomotion is one of the fundamental skills in robotics. Legged robots can navigate in complex terrains and overcome complex obstacles, allowing the deployment of robotics platforms in real-world environments.
Our research encompasses different types of platforms such as humanoids, quadruped, and bioinspired robots. To learn useful robot gaits, we investigate many different techniques, including reinforcement learning, imitation learning, inverse reinforcement learning, or more structured approaches.
Multi Embodiment Locomotion 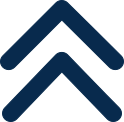
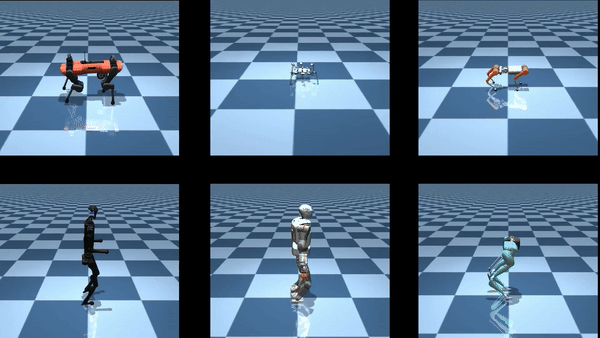
One Policy to Run Them All: an End-to-end Learning Approach to Multi-Embodiment Locomotion. While there exists a wide variety of legged platforms such as quadruped, humanoids, and hexapods, the field is still missing a single learning framework that can control all these different embodiments easily and effectively and possibly transfer, zero or few-shot, to unseen robot embodiments. To close this gap, we introduce URMA, the Unified Robot Morphology Architecture. Our framework brings the end-to-end Multi-Task Reinforcement Learning approach to the realm of legged robots, enabling the learned policy to control any type of robot morphology. The key idea of our method is to allow the network to learn an abstract locomotion controller that can be seamlessly shared between embodiments thanks to our morphology-agnostic encoders and decoders. This flexible architecture can be seen as a first step in building a foundation model for legged robot locomotion. Our experiments show that URMA can learn a locomotion policy on multiple embodiments that can be easily transferred to unseen robot platforms in simulation and the real world.
- Bohlinger, N.; Czechmanowski, G.; Krupka, M.; Kicki, P.; Walas, K.; Peters, J.; Tateo, D. (2024). One Policy to Run Them All: an End-to-end Learning Approach to Multi-Embodiment Locomotion, Conference on Robot Learning (CoRL).
- Bohlinger, N.; Czechmanowski, G.; Krupka, M.; Kicki, P.; Walas, K.; Peters, J.; Tateo, D. (2024). One Policy to Run Them All: an End-to-end Learning Approach to Multi-Embodiment Locomotion, CoRL 2024 Workshop on Morphology-Aware Policy and Design Learning Workshop.
Biomechanics 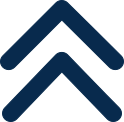
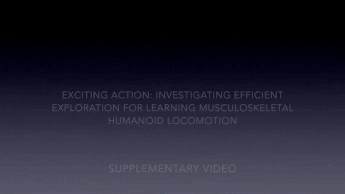
Exciting Action: Investigating Efficient Exploration for Learning Musculoskeletal Humanoid Locomotion. Learning a locomotion controller for a musculoskeletal system is challenging due to over-actuation and high-dimensional action space. While many reinforcement learning methods attempt to address this issue, they often struggle to learn human-like gaits because of the complexity involved in engineering an effective reward function. In this paper, we demonstrate that adversarial imitation learning can address this issue by analyzing key problems and providing solutions using both current literature and novel techniques. We validate our methodology by learning walking and running gaits on a simulated humanoid model with 16 degrees of freedom and 92 Muscle-Tendon Units, achieving natural-looking gaits with only a few demonstrations.
- Geiss, H.J.; Al-Hafez, F.; Seyfarth, A.; Peters, J.; Tateo, D. (2024). Exciting Action: Investigating Efficient Exploration for Learning Musculoskeletal Humanoid Locomotion, IEEE-RAS International Conference on Humanoid Robots (Humanoids).
Benchmark 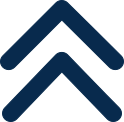
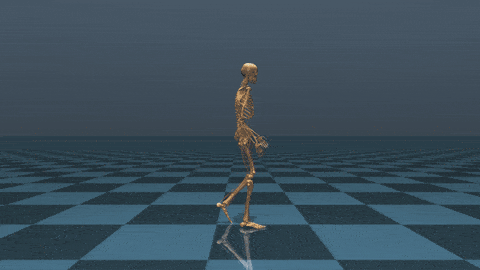
LocoMuJoCo: A Comprehensive Imitation Learning Benchmark for Locomotion. Imitation Learning (IL) holds great promise for enabling agile locomotion in embodied agents. However, many existing locomotion benchmarks primarily focus on simplified toy tasks, often failing to capture the complexity of real-world scenarios and steering research toward unrealistic domains. To advance research in IL for locomotion, we present a novel benchmark designed to facilitate rigorous evaluation and comparison of IL algorithms. This benchmark encompasses a diverse set of environments, including quadrupeds, bipeds, and musculoskeletal human models, each accompanied by comprehensive datasets, such as real noisy motion capture data, ground truth expert data, and ground truth sub-optimal data, enabling evaluation across a spectrum of difficulty levels. To increase the robustness of learned agents, we provide an easy interface for dynamics randomization and offer a wide range of partially observable tasks to train agents across different embodiments. Finally, we provide handcrafted metrics for each task and ship our benchmark with state-of-the-art baseline algorithms to ease evaluation and enable fast benchmarking.
- Al-Hafez, F.; Zhao, G.; Peters, J.; Tateo, D. (2023). LocoMuJoCo: A Comprehensive Imitation Learning Benchmark for Locomotion, Robot Learning Workshop, Conference on Neural Information Processing Systems (NeurIPS).