Upon graduation, I have joined the Robotics Institute at Carnegie Mellon University where I was a Systems Scientist faculty member. Now I am at Google!
Katharina Muelling
Research Interests
Robotics, Machine Learning, Human Motor Control.
More Information
Curriculum Vitae Publications [Google Citations] [DBLP]
Contact Information
Mail. 10th at 40th, Pittsburgh, PA 15232, USA
kmuelling [at] nrec [dot] ri [dot] cmu [dot] edu
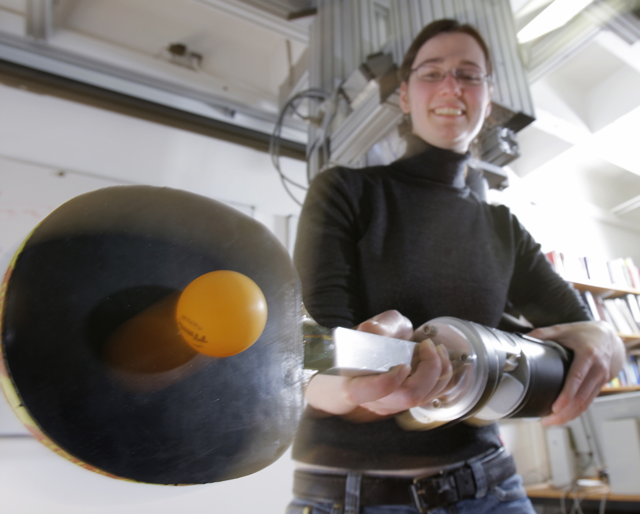
Katharina Muelling has joined the National Robotics Engineering Center at Carnegie Mellon University as a project scientist in October 2013. Before going to Pittsburgh, she did her PhD at the Max-Planck Institute for Intelligent Systems in the departments of Bernahrd Schoelkopf and Stefan Schaal under the supervision of Jan Peters. Please see her curriculum vitae for more biographical information.
During her thesis her work focused on motor control and learning in complex motor tasks such as table tennis. Table tennis is ideal for studying complex motor skills as it requires fast movements, accurate control, adaptation to new parameters and is based on several elemental movements. In this context, she is interested both in human motor control as well as synthetic robotics approaches.
Katharina Muelling was featured in Engadget and the New Scientist. Katharina Muelling can be found on [Google Citations] and [DBLP].
Research Interests: Robotics, Computational Models of Human Motor Control, Robot Learning Architectures, Inverse Reinforcement Learning, Learning by Demonstration, Manipulation and Human-Robot-Interaction
Collaborators: Jens Kober, Oliver Kroemer, Zhikun Wang, Abdeslam Boularias, Betty Mohler, Jan Peters
Key References - More References here!
-
- Muelling, K.; Boularias, A.; Schoelkopf, B.; Peters, J. (2014). Learning Strategies in Table Tennis using Inverse Reinforcement Learning, Biological Cybernetics, 108, 5, pp.603-619.
-
- Muelling, K.; Kober, J.; Kroemer, O.; Peters, J. (2013). Learning to Select and Generalize Striking Movements in Robot Table Tennis, International Journal of Robotics Research (IJRR), 32, 3, pp.263-279.
-
- Muelling, K.; Kober, J.; Peters, J. (2011). A Biomimetic Approach to Robot Table Tennis, Adaptive Behavior Journal, 19, 5.
Projects
Extracting strategic information
Learning a complex task such as table tennis is a challenging problem for both robots and humans. Even after acquiring the necessary motor skills, a strategy is needed to choose where and how to return the ball to the opponent’s court in order to win the game. The goal of this project was to develop a Markov Decision Process (MDP) framework for table tennis, where the reward function models the goal of the task as well as the strategic information. We showed how this reward function can be discovered from demonstrations of table tennis matches using model-free inverse reinforcement learning. The resulting framework allowed us to identify basic elements on which the selection of striking movements is based. The approach was tested on data collected from players with different playing styles and under different playing conditions. The estimated reward function was able to capture expert-specific strategic information that sufficed to distinguish the expert among players with different skill levels as well as different playing styles.
- Muelling, K.; Boularias, A.; Schoelkopf, B.; Peters, J. (2014). Learning Strategies in Table Tennis using Inverse Reinforcement Learning, Biological Cybernetics, 108, 5, pp.603-619.
Towards Learning Robot Table Tennis
Autonomously learning new motor tasks from physical interactions is an important goal for both robotics and machine learning. However, when moving beyond basic skills, most monolithic machine learning approaches fail to scale. For more complex skills, methods that are tailored for the domain of skill learning are needed. In this project, we present a new framework that enables a robot to learn basic cooperative table tennis from demonstration and interaction with a human player. To achieve this goal, we created an initial movement library from kinesthetic teach-in and imitation learning. The movements stored in the movement library can be selected and generalized using the proposed mixture of motor primitives algorithm. As a result, we obtain a task policy that is composed of several motor primitives weighted by their ability to generate successful movements in the given task context. These weights are computed by a gating network and can be updated autonomously.
- Muelling, K.; Kober, J.; Kroemer, O.; Peters, J. (2013). Learning to Select and Generalize Striking Movements in Robot Table Tennis, International Journal of Robotics Research (IJRR), 32, 3, pp.263-279.
Biomimetic Robot Table Tennis Player
Playing table tennis is a difficult motor task that require fast movements, accurate control and adaptation to task parameters. Although human beings see and move slower than most robot systems, they significantly outperform all table tennis robots. One important reason for this higher performance is the human movement generation. In this project, we study human movements during a table tennis match and present a robot system that mimics human striking behavior. Our focus lies on generating hitting motions capable of adapting to variations in environmental conditions, such as changes in ball speed and position.
- Muelling, K.; Kober, J.; Peters, J. (2011). A Biomimetic Approach to Robot Table Tennis, Adaptive Behavior Journal, 19, 5.