Meet with us at CoRL 2024!
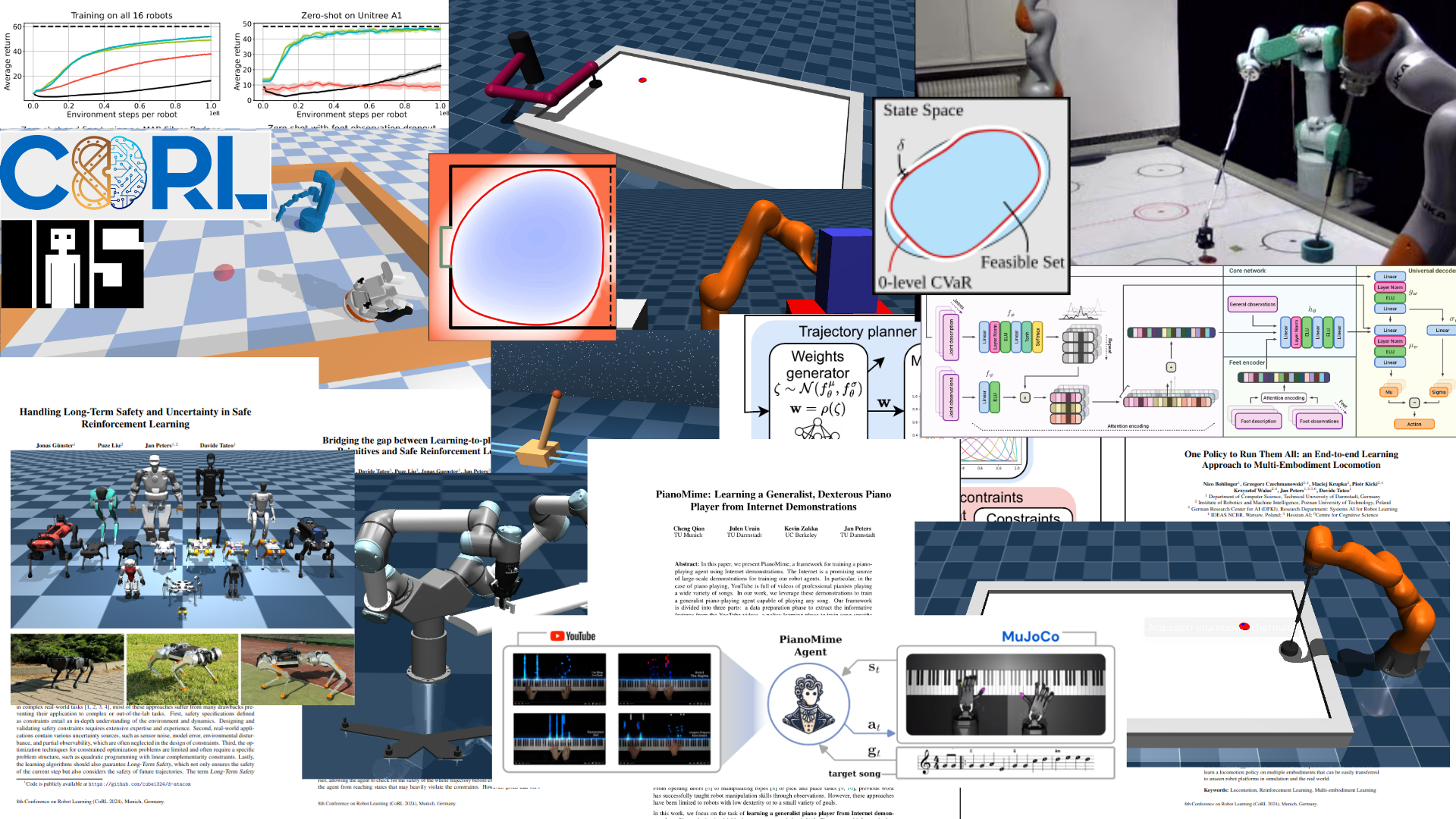
Accepted Papers
We have four papers accepted at CoRL 2024!
- "PianoMime: Learning a Generalist, Dexterous Piano Player from Internet Demonstrations", Cheng Qian, Julen Urain, Kevin Zakka, and Jan Peters [ website ] [ paper ] [ X ]
- "One Policy to Run Them All: an End-to-end Learning Approach to Multi-Embodiment Locomotion", Nico Bohlinger, Grzegorz Czechmanowski, Maciej Piotr Krupka, Piotr Kicki, Krzysztof Walas, Jan Peters, Davide Tateo [ website ] [ paper ] [ X ]
- "Handling Long-Term Safety and Uncertainty in Safe Reinforcement Learning", Jonas Günster, Puze Liu, Jan Peters, Davide Tateo [ website ] [ paper ] [ X ]
- "Bridging the gap between Learning-to-plan, Motion Primitives and Safe Reinforcement Learning", Piotr Kicki, Davide Tateo, Puze Liu, Jonas Günster, Jan Peters, Krzysztof Walas [ website ] [ paper ] [ X ]
take a look at our thread on X for more details.
Accepted Workshop Papers
We also have the following workshop papers accepted at CoRL 2024:
- Lenz, J.; Gruner, T.; Palenicek, D.; Schneider, T.; Pfenning, I.; Peters J. (2024). Analysing the Interplay of Vision and Touch for Dexterous Insertion Tasks, CoRL 2024 Workshop on Learning Robot Fine and Dexterous Manipulation: Perception and Control.
- Le, A. T.; Hansel, K.; Carvalho, J.; Urain, J.; Biess, A.; Chalvatzaki, G.; Peters, J. (2024). Global Tensor Motion Planning, CoRL 2024 Workshop on Differentiable Optimization Everywhere.
- Nguyen, D.H.; Schneider, T.; Duret, G.; Kshirsagar, A.; Belousov, B.; Peters, J. (2024). TacEx: GelSight Tactile Simulation in Isaac Sim – Combining Soft-Body and Visuotactile Simulators, CoRL 2024 Workshop on Learning Robot Fine and Dexterous Manipulation: Perception and Control.
- Kornmann, M.; He, Q.; Kshirsagar, A.; Ploeger, K.; Peters, J. (2024). Learning to Accurately Throw Paper Planes, CoRL 2024 Workshop on Learning Robot Fine and Dexterous Manipulation: Perception and Control.
- Bohlinger, N.; Czechmanowski, G.; Krupka, M.; Kicki, P.; Walas, K.; Peters, J.; Tateo, D. (2024). One Policy to Run Them All: an End-to-end Learning Approach to Multi-Embodiment Locomotion, CoRL 2024 Workshop on Morphology-Aware Policy and Design Learning Workshop.
- Drolet, M.; Stepputtis, S.; Kailas, S.; Jain, A.; Schaal, S.; Peters, J.; Ben Amor, H. (2024). A Comparison of Imitation Learning Algorithms for Bimanual Manipulation, CoRL 2024 Workshop on Whole-body Control and Bimanual Manipulation.
- Funk, N.; Urain, J.; Carvalho, J.; Prasad, V.; Chalvatzaki, G.; Peters, J. (2024). ACTIONFLOW: Equivariant, Accurate, and Efficient Manipulation Policies with Flow Matching, CoRL 2024 Workshop on Mastering Robot Manipulation in a World of Abundant Data.
- Kaidanov, O.; Al-Hafez, F.; Süvari, Y.; Belousov, B.; Peters, J. (2024). The Role of Domain Randomization in Training Diffusion Policies for Whole-Body Humanoid Control, CoRL 2024 Workshop on Whole-body Control and Bimanual Manipulation: Applications in Humanoids and Beyond.
- Holgado-Alvarez, J.H.; Reddi, A.; D'Eramo, C. (2024). Dynamic Obstacle Avoidance with Bounded Rationality Adversarial Reinforcement Learning, CoRL 2024 Locolearn Workshop.
Workshops
You can also meet us at the LocoLearn and the MAPoDeL Workshop!
LocoLearn: From Bioinspired Gait Generation to Active Perception
Workshop date: Saturday, November 9th, 2024
Machine learning, particularly Reinforcement Learning, has revolutionized the development of advanced locomotion controllers. These advancements have enabled legged robots not only to locomote on rough terrain but also navigate in complex environments with remarkable agility.“Robot Parkour” is one of these achievements—robots can now jump on boxes, cross narrow passages, overcome large gaps, or crawl beneath low barriers.
While the agility of robots improves at a fast pace, we are still far from the robustness and versatility we can find in nature. Animals leverage their morphology and senses with their capacity to adapt in the wild to achieve extraordinary locomotion capabilities. For robots to be able to achieve this level of performance, we need a comprehensive view that encompasses what we observe on animals, where perception, action, and learning are tightly coupled.
In this workshop, we will discuss the future of locomotion learning research from this holistic perspective. To achieve this, we will gather top researchers in locomotion learning (LL), bioinspiration (BIO), and multi-modal and active perception (MAP). Our objective is to understand how these diverse disciplines talk to each other and converge toward the development of agile, robust, and safe locomotion algorithms.
In this workshop we aim to answer the following research questions:
- Can we go beyond imitation of animals' locomotion, and use other biological insights, like action-perception loop, to develop better locomotion learning frameworks?
- Can multimodal active perception improve the robot's agility, learning performance, or robustness?
- How important is contact sensing for locomotion? Should we exploit contacts rather than avoid them?
- How important is it to perceive terrain properties during locomotion? Can we adapt locomotion to deal with different terrains? How can we simulate terrain?
- Can we learn directly on real platforms? Do we need safety techniques to learn in the real world?
- How can we learn to switch between different gaits using perception, e.g., from walking in the mud to swimming?
- How to exploit these complex locomotion skills and advanced perception to solve long-term or high-level navigation tasks?
- How can we leverage the foundational models for improving multimodal and active perception for locomotion? Are foundational models an answer to all the questions above?
MAPoDeL: Morphology-Aware Policy and Design Learning Workshop
Workshop date: Saturday, November 9th, 2024
Morphology-aware policy research offers an exciting intersection of multiple control paradigms, including foundation models, multi-task learning, meta-learning, and co-design learning. In this workshop, we are interested in bringing together researchers from the fields of robotics, machine learning and evolutionary robotics who study morphology-aware policies in order to facilitate connections between the fields' perspectives on the subject. Experts in the three areas understand the importance of such policies but bring different ideas to address the problems. Roboticists are concerned especially with sample efficiency and control on real robots, whereas machine learning researchers are interested in scaling large models with larger datasets and creating artificial generalist agents. In this workshop, we hope to bridge these perspectives in such a way that it helps foster new research directions to contribute to research that advances the field of morphology-aware algorithms.
Topics of Interest:
- Multi-Embodiment Learning
- Morphology-Agnostic or -Aware Policy Learning
- Reinforcement/Robot Learning for multiple robot morphologies or platforms
- Evolutionary Robotics
- Representation Learning for Multi-Embodiment / Multi-Task Problems
- Co-Design / Co-Adaptation / Co-Optimization of Robot Morphology and Behavior
- Computational Design
- Foundation models for robotics