Locomotion
Locomotion is one of the fundamental skills in robotics. Legged robots can navigate in complex terrains and overcome complex obstacles, allowing the deployment of robotics platforms in real-world environments. Our research encompasses different types of platforms such as humanoids, quadruped, and bioinspired robots. To learn useful robot gaits, we investigate many different techniques, including reinforcement learning, imitation learning, inverse reinforcement learning, or more structured approaches.
Benchmark
LocoMuJoCo: A Comprehensive Imitation Learning Benchmark for Locomotion
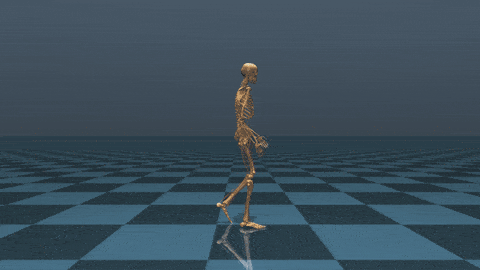
Imitation Learning (IL) holds great promise for enabling agile locomotion in embodied agents. However, many existing locomotion benchmarks primarily focus on simplified toy tasks, often failing to capture the complexity of real-world scenarios and steering research toward unrealistic domains. To advance research in IL for locomotion, we present a novel benchmark designed to facilitate rigorous evaluation and comparison of IL algorithms. This benchmark encompasses a diverse set of environments, including quadrupeds, bipeds, and musculoskeletal human models, each accompanied by comprehensive datasets, such as real noisy motion capture data, ground truth expert data, and ground truth sub-optimal data, enabling evaluation across a spectrum of difficulty levels. To increase the robustness of learned agents, we provide an easy interface for dynamics randomization and offer a wide range of partially observable tasks to train agents across different embodiments. Finally, we provide handcrafted metrics for each task and ship our benchmark with state-of-the-art baseline algorithms to ease evaluation and enable fast benchmarking.
-
- Al-Hafez, F.; Zhao, G.; Peters, J.; Tateo, D. (2023). LocoMuJoCo: A Comprehensive Imitation Learning Benchmark for Locomotion, Robot Learning Workshop, Conference on Neural Information Processing Systems (NeurIPS).