Statistical Machine Learning
Our Robot Learning Lab is at the forefront of pioneering research in statistical machine learning, driving innovation and expanding the boundaries of robotics and automation. Our cutting-edge research leverages optimal transport for trajectory optimization, enabling robots to plan and execute complex movements more efficiently and with greater precision. Furthermore, we delve into likelihood-free inference, a method that allows our robots to make informed decisions even when dealing with complex models for which likelihood is intractable. Additionally, we are advancing probabilistic system identification, which helps robots understand and adapt to their environment by constructing probabilistic models that capture the inherent uncertainties of the real world. Together, these innovative approaches are not just enhancing the capabilities of robots but are also pushing the boundaries of what's possible in the realm of intelligent systems, promising a future where robots can learn, adapt, and operate with unprecedented autonomy and effectiveness.
Bayesian Machine Learning
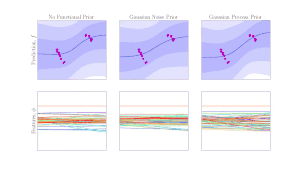
Bayesian methods are a principled way of endowing our predictive models with uncertainty quantification and regularization from overfitting. In the context of robot learning, the uncertainty from these models can be leveraged for exploration, active learning and regularization. We are interested in developing Bayesian models that scale to real-world robotic data which can then be used for downstream robot learning.
- Ting, J.;Mistry, M.;Nakanishi, J.;Peters, J.;Schaal, S. (2006). A Bayesian approach to nonlinear parameter identification for rigid body dynamics, Robotics: Science and Systems (RSS 2006), Cambridge, MA: MIT Press.
- Calandra, R.; Peters, J.; Rasmussen, C.E.; Deisenroth, M.P. (2016). Manifold Gaussian Processes for Regression, Proceedings of the International Joint Conference on Neural Networks (IJCNN).
- Abdulsamad, H.; Nickl, P.; Klink, P.; Peters, J. (2024). Variational Hierarchical Mixtures for Probabilistic Learning of Inverse Dynamics, IEEE Transactions on Pattern Analysis and Machine Intelligence (PAMI), 46, 4, pp.1950-1963.
- Watson, J.; Lin, J. A.; Klink, P.; Peters, J. (2021). Neural Linear Models with Functional Gaussian Process Priors, 3rd Symposium on Advances in Approximate Bayesian Inference (AABI).
Likelihood-Free Inference
Pseudo-Likelihood Inference
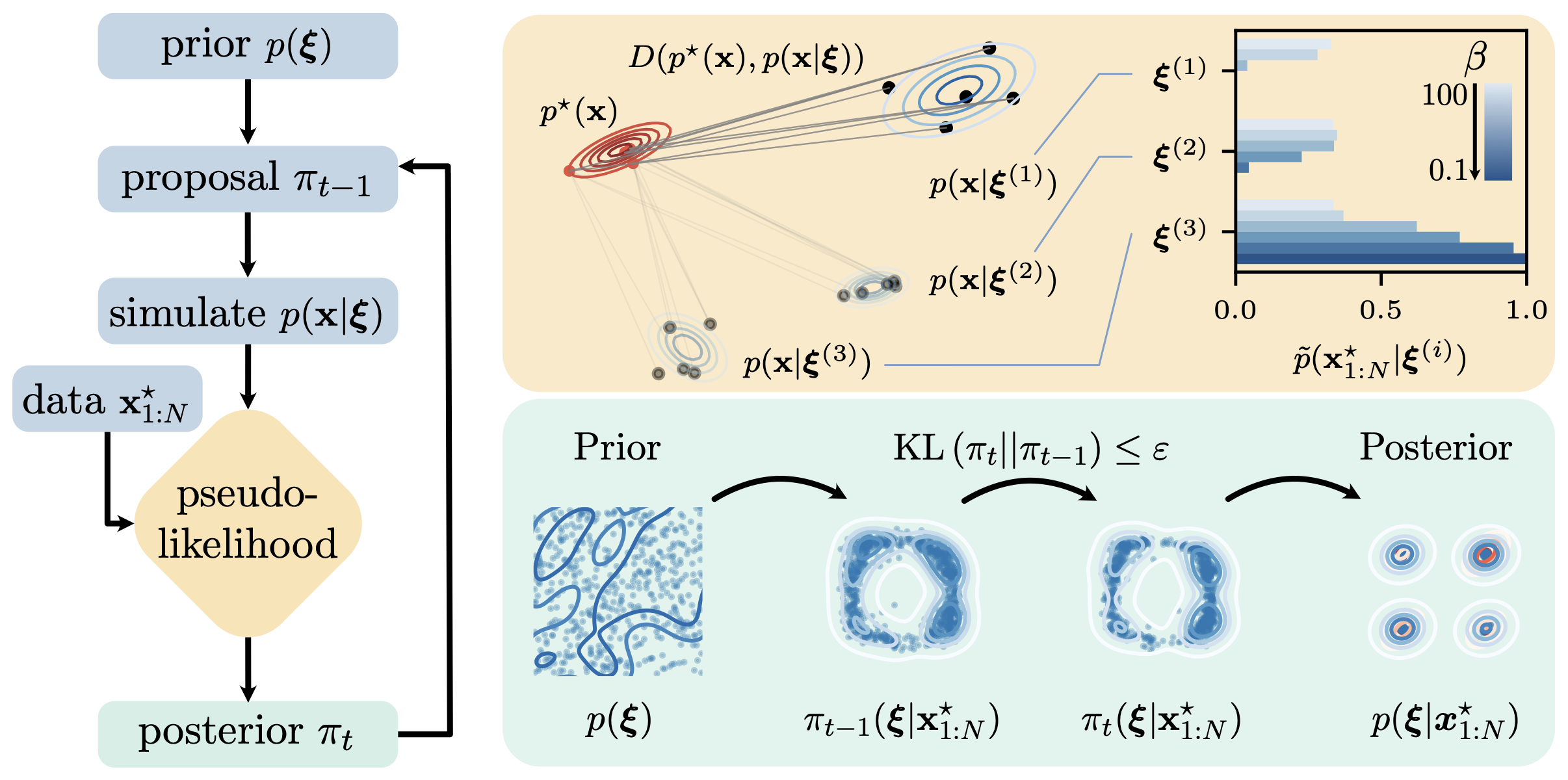
Simulation-Based Inference (SBI) is a common name for an emerging family of approaches that infer the model parameters when the likelihood is intractable. Existing SBI methods either approximate the likelihood, such as Approximate Bayesian Computation (ABC) or directly model the posterior, such as Sequential Neural Posterior Estimation (SNPE). While ABC is efficient on low-dimensional problems, on higher-dimensional tasks, it is generally outperformed by SNPE, which leverages function approximation. In this paper, we propose Pseudo-Likelihood Inference (PLI), a new method that brings neural approximation into ABC, making it competitive on challenging Bayesian system identification tasks. By utilizing integral probability metrics, we introduce a smooth likelihood kernel with an adaptive bandwidth that is updated based on information-theoretic trust regions. Thanks to this formulation, our method (i) allows for optimizing neural posteriors via gradient descent, (ii) does not rely on summary statistics, and (iii) enables multiple observations as input. In comparison to SNPE, it leads to improved performance when more data is available. The effectiveness of PLI is evaluated on four classical SBI benchmark tasks and on a highly dynamic physical system, showing particular advantages on stochastic simulations and multi-modal posterior landscapes.
- Gruner, T.; Belousov, B.; Muratore, F.; Palenicek, D.; Peters, J. (2023). Pseudo-Likelihood Inference, Advances in Neural Information Processing Systems (NIPS / NeurIPS).