I have moved to University of Tokyo in Japan where I am an Assistant Professor.
Takayuki Osa
Research Interests
Learning for Object Manipulation, Bilateral Control, Surgical Systems
More Information
Contact Information
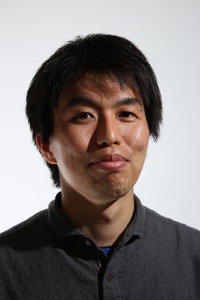
During his doctoral program, Takayuki worked on autonomous assistance in robotic surgery under the supervision of Prof. Mamoru Mitsuishi and Prof. Naohiko Sugita. To improve the performance of conventional master-slave systems for robotic surgery, he worked on automation of surgical tasks by learning from demonstration.
Before starting the doctoral program, from 2010 to 2012, Takayuki worked at Terumo Corporation investigating the mechanical design of medical devices for a cardiac disease. While his master program, he studied at Technical University Munich from 2008 to 2009, and worked on visual servoing under the guidance of Prof. Alois Knoll.
Research interests
- Learning for robotic manipulation
- Bilateral control
- Surgical robots
Key References
-
- Osa, T.; Pajarinen, J.; Neumann, G.; Bagnell, J.A.; Abbeel, P.; Peters, J. (2018). An Algorithmic Perspective on Imitation Learning, Foundations and Trends in Robotics.
- Osa, T.; Pajarinen, J.; Neumann, G.; Bagnell, J. A.; Abbeel, P.; Peters, J. (2017). An Algorithmic Perspective on Imitation Learning.
-
- Osa, T.; Peters, J.; Neumann, G. (2016). Experiments with Hierarchical Reinforcement Learning of Multiple Grasping Policies, Proceedings of the International Symposium on Experimental Robotics (ISER).
-
- Osa, T.; Sugita, N.; Mitsuishi, M. (2014). Online Trajectory Planning in Dynamic Environments for Surgical Task Automation, Proceedings of Robotics: Science and Systems (R:SS).
-
-
- Osa, T.; Abawi, C.F.; Sugita, N.; Chikuda, H.; Sugita, S.; Tanaka, T.; Oshima, H.; Moro, T.; Tanaka, S.; Mitsuishi, M. (2015). Hand-Held Bone Cutting Tool With Autonomous Penetration Detection for Spinal Surgery , IEEE/ASME Transactions on Mechatronics.
Guiding Trajectory Optimization by Demonstrated Distributions
(:youtube rp_znodwlq0:) Trajectory optimization is an essential tool for motion planning under multiple constraints of robotic manipulators. Optimization-based methods can explicitly optimize a trajectory by leveraging prior knowledge of the system and have been used in various applications such as collision avoidance. However, these methods often require a hand-coded cost function in order to achieve the desired behavior. Specifying such cost function for a complex desired behavior, e.g., disentangling a rope, is a non-trivial task that is often even infeasible. Learning from demonstration (LfD) methods offer an alternative way to program robot motion. LfD methods are less dependent on analytical models and instead learn the behavior of experts implicitly from the demonstrated trajectories. However, the problem of adapting the demonstrations to new situations, e.g., avoiding newly introduced obstacles, has not been fully investigated in the literature.
In this work, we present a motion planning framework that combines the advantages of optimization-based and demonstration-based methods. We learn a distribution of trajectories demonstrated by human experts and use it to guide the trajectory optimization process. The resulting trajectory maintains the demonstrated behaviors, which are essential to performing the task successfully, while adapting the trajectory to avoid obstacles. In simulated experiments and with a real robotic system, we verify that our approach optimizes the trajectory to avoid obstacles and encodes the demonstrated behavior in the resulting trajectory.
-
- Osa, T.; Ghalamzan, E. A. M.; Stolkin, R.; Lioutikov, R.; Peters, J.; Neumann, G. (2017). Guiding Trajectory Optimization by Demonstrated Distributions, IEEE Robotics and Automation Letters (RA-L), 2, 2, pp.819-826, IEEE.
Hierarchical Reinforcement Learning of Multiple Grasping Policies
(:youtube irwFYx0PycQ:) Robotic grasping has attracted considerable interest, but it still remains a challenging task. The data-driven approach is a promising solution to the robotic grasping problem; this approach leverages a grasp dataset and generalizes grasps for various objects. However, these methods often depend on the quality of the given datasets, which are not trivial to obtain with sufficient quality. Although reinforcement learning approaches have been recently used to achieve autonomous collection of grasp datasets, the existing algorithms are often limited to specific grasp types.
In this work, we developed a framework for hierarchical reinforcement learning of grasping policies. In our framework, the lower-level hierarchy learns multiple grasp types, and the upper-level hierarchy learns a policy to select from the learned grasp types according to a point cloud of a new object. Through experiments, we validate that our approach learns grasping by constructing the grasp dataset autonomously. The experimental results show that our approach learns multiple grasping policies and generalizes the learned grasps by using local point cloud information.
-
- Osa, T.; Peters, J.; Neumann, G. (2016). Experiments with Hierarchical Reinforcement Learning of Multiple Grasping Policies, Proceedings of the International Symposium on Experimental Robotics (ISER).
Online Trajectory Planning in Dynamic Environments for Surgical Task Automation
Automation of surgical tasks is expected to improve the quality of surgery. In this work, we addressed two issues that must be resolved for automation of robotic surgery: online trajectory planning and force control under dynamic conditions. This study presents a framework of online trajectory planning and force control by learning from demonstrations. By leveraging demonstration under various conditions, we can model the conditional distribution of the trajectories given the task condition. This scheme enables generalization of the trajectories of spatial motion and contact force to new conditions in real time. In addition, we propose a force tracking controller that robustly and stably tracks the planned trajectory of the contact force by learning the spatial motion and contact force simultaneously. The proposed scheme was tested with bimanual tasks emulating surgical tasks that require online trajectory planning and force tracking control, such as tying knots and cutting soft tissues. Experimental results showed that the proposed scheme enables planning of the task trajectory under dynamic conditions in real time. Additionally, the performance of the force control schemes was verified in the experiments.
(:youtube 7J8aUSVUP58:) (:youtube J9edQ7FIoWs:)
-
- Osa, T.; Sugita, N.; Mitsuishi, M. (accepted). Online Trajectory Planning and Force Control for Automation of Surgical Tasks, IEEE Transactions on Automation Science and Engineering.
-
- Osa, T.; Sugita, N.; Mitsuishi, M. (2014). Online Trajectory Planning in Dynamic Environments for Surgical Task Automation, Proceedings of Robotics: Science and Systems (R:SS).